Paper:
Design of a Database-Driven Kansei Feedback Control System Using a Hydraulic Excavators Simulator
Takuya Kinoshita*1, Hiroaki Ikeda*2, Toru Yamamoto*1, Maro G. Machizawa*3, Kiyokazu Tanaka*4, and Yoichiro Yamazaki*4
*1Graduate School of Advanced Science and Engineering, Hiroshima University
1-4-1 Kagamiyama, Higashi-hiroshima, Hiroshima 739-8527, Japan
*2Graduate School of Engineering, Hiroshima University
1-4-1 Kagamiyama, Higashi-hiroshima, Hiroshima 739-8527, Japan
*3Center for Brain, Mind and KANSEI Sciences Research, Hiroshima University
1-2-3 Kasumi, Minami-ku, Hiroshima 734-8553, Japan
*4KOBELCO Construction Machinery Co., Ltd.
Osaki Bright Core Bldg., 5-15 Kitashinagawa 5-chome, Shinagawa-ku, Tokyo 141-8626, Japan
In Japan, the level of happiness is considered low despite the gross domestic product (GDP) being high, and a wide gap separates “products wealth” related to GDP and “mental wealth such as Kansei” related to the level of happiness. To fill this gap, products should be controlled to enhance Kansei according to human feelings. However, it is difficult to obtain the Kansei model because of time-variant and nonlinear system. In this paper, the design of a data-oriented cascade control system based on Kansei is newly proposed. In particular, a database-driven controller is designed for a human based on Kansei. The effectiveness of the proposed scheme is verified by using the electroencephalograph (EEG).
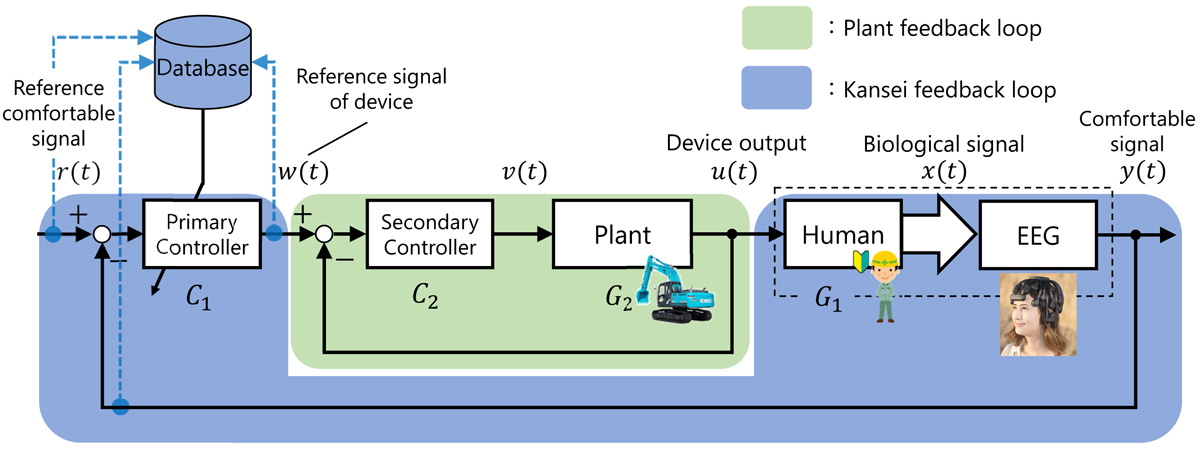
Block diagram of the proposed Kansei feedback control system
- [1] The Commission on Measuring Well-being, Japan, “Measuring National Well-Being – Proposed Well-being Indicators –,” Cabinet Office, Government of Japan, 2011 (in Japanese).
- [2] K. Hiraki and N. Kanayama (Eds.), “Introduction to electroencephalogram analysis – Using EEGLAB and SPM –,” University of Tokyo Press, 2016
- [3] T. Yamamoto, K. Takao, and T. Yamada, “Design of a Data-Driven PID Controller,” IEEE Trans. on control systems Technology, Vol.17, No.1, pp. 29-39, 2009.
- [4] S. Wakitani and T. Yamamoto, “A Learning Algorithm for a Data-Driven Controller Based on Fictitious Reference Iterative Tuning,” Proc. of 2016 Int. Joint Conf. on Neural Networks (IJCNN 2016), pp. 4908-4913, 2016.
- [5] T. Kinoshita and T. Yamamoto, “Design of a Data-Oriented Cascade Control System,” Proc. of the 6th Int. Symp. on Advanced Control of Industrial Processes (AdCONIP), pp. 365-370, 2017.
- [6] Y. Oshima, T. Kinoshita, K. Koiwai, T. Yamamoto, T. Nanjo, Y. Yamazaki, and Y. Fujimoto, “Data-Driven Torque Controller for a Hydraulic Excavator,” J. Robot. Mechatron., Vol.28, No.5, pp. 752-758, 2016.
- [7] O. Kaneko, “Data-Driven Controller Tuning: FRIT Approach,” Proc. of the 11th IFAC Int. Workshop on Adaptation and Learning in Control and Signal Processing, pp. 326-336, 2013.
- [8] T. Yamamoto and S. L. Shah, “Design and Experimental Evaluation of a Multivariable Self-Tuning PID Controller,” Proc. of IEEE Conf. on Control Appl., Trieste, pp. 1230-1234, 1997.
- [9] J. G. Zieglar and N. B. Nichols, “Optimum settings for automatic controllers,” Trans. ASME, Vol.64, No.8, pp. 759-768, 1942.
- [10] K. L. Chien, J. A. Hrones, and J. B. Reswick, “On the Automatic Control of Generalized Passive Systems,” Trans. ASME, Vol.74, pp. 175-185, 1972.
- [11] Y. Okamoto and I. Homma, “Electroencephalography and its Inverse Analysis,” IEEJ Trans. on Electronics, Information and Systems, Vol.122, No.9, pp. 1417-1425, 2002 (in Japanese).
- [12] H. H. Jasper, “The Ten-Twenty Electrode System of the International Federation,” Electroenceph. Clin. Neurophysiol., Vol.10, pp. 371-375, 1958.
- [13] G. Lisi, T. Noda, and J. Morimoto, “Decoding the ERD/ERS: influence of afferent input induced by a leg assistive robot,” Frontiers in Systems Neuroscience, Vol.8, pp. 1-12, 2014.
- [14] M. Machizawa, G. Lisi, N. Kanayama, R. Mizuochi, K. Makita, T. Sasaoka, and S. Yamawaki, “Quantification of anticipation of excitement with a three-axial model of emotion with EEG,” J. Neural. Eng., 2020 (in press).
- [15] K. Koiwai, Y. Liao, T. Yamamoto, T. Nanjo, Y. Yamazaki, and Y. Fujimoto, “Feature Extraction for Excavator Operation Skill Using CMAC,” J. Robot. Mechatron., Vol.28, No.5, pp. 715-721, 2016.
- [16] I. P. Herman, “Physics of the Human Body: Biological and Medical Physics, Biomedical Engineering,” Springer, 2007.
This article is published under a Creative Commons Attribution-NoDerivatives 4.0 Internationa License.