Abstract
In this paper, we report on the developed system for assessment of ground unit terrain traversal cost using aerial reconnaissance of the expected mission environment. The system combines an aerial vehicle with ground robot terrain learning in the traversal cost modeling utilized in the mission planning for ground units. The aerial vehicle is deployed to capture visual data used to build a terrain model that is then used for the extraction of the terrain features of the expected operational area of the ground units. Based on the previous traversal experience of the ground units in similar environments, the learned model of the traversal cost is employed to predict the traversal cost of the new expected operational area to plan a cost-efficient path to visit the desired locations of interest. The particular modules of the system are demonstrated in an experimental scenario combining the deployment of an unmanned aerial vehicle with a multi-legged walking robot used for learning the traversal cost model.
Access this chapter
Tax calculation will be finalised at checkout
Purchases are for personal use only
Similar content being viewed by others
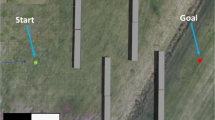
References
Alatartsev, S., Stellmacher, S., Ortmeier, F.: Robotic task sequencing problem: a survey. J. Intell. Robot. Syst. 80(2), 279–298 (2015). https://doi.org/10.1007/s10846-015-0190-6
Belter, D., Wietrzykowski, J., Skrzypczyński, P.: Employing natural terrain semantics in motion planning for a multi-legged robot. J. Intell. Rob. Syst. 93(3), 723–743 (2018). https://doi.org/10.1007/s10846-018-0865-x
Brunner, M., Brüggemann, B., Schulz, D.: Rough terrain motion planning for actuated, tracked robots. In: Filipe, J., Fred, A. (eds.) ICAART 2013. CCIS, vol. 449, pp. 40–61. Springer, Heidelberg (2014). https://doi.org/10.1007/978-3-662-44440-5_3
Felizardo, L.F., Mota, R.L., Shiguemori, E.H., Neves, M.T., Ramos, A.B., Mora-Camino, F.: Using ANN and UAV for terrain surveillance. In: International Conference on Hybrid Intelligent Systems (HIS), pp. 1–5 (2014). https://doi.org/10.1109/HIS.2013.6920414
Gutin, G., Punnen, A.P. (eds.): The Traveling Salesman Problem and Its Variations. Springer, Boston (2007). https://doi.org/10.1007/b101971
Helsgaun, K.: An effective implementation of the Lin-Kernighan traveling salesman heuristic. Eur. J. Oper. Res. 126(1), 106–130 (2000)
Helsgaun, K.: LKH solver 2.0.9. http://www.akira.ruc.dk/~keld/research/LKH. Accessed 29 Aug 2019
Hudjakov, R., Tamre, M.: Aerial imagery terrain classification for long-range autonomous navigation. In: International Symposium on Optomechatronic Technologies, pp. 88–91 (2009). https://doi.org/10.1109/ISOT.2009.5326104
Kohonen, T.: The self-organizing map. Proc. IEEE 78(9), 1464–1480 (1990). https://doi.org/10.1109/5.58325
Kottege, N., Parkinson, C., Moghadam, P., Elfes, A., Singh, S.P.N.: Energetics-informed hexapod gait transitions across terrains. In: IEEE International Conference on Robotics and Automation (ICRA), pp. 5140–5147. IEEE (2015). https://doi.org/10.1109/ICRA.2015.7139915
LWPR library (2007). https://github.com/jdlangs/lwpr. Accessed 28 May 2019
Prágr, M., Čížek, P., Bayer, J., Faigl, J.: Online incremental learning of the terrain traversal cost in autonomous exploration. In: Robotics: Science and Systems (RSS) (2019). https://doi.org/10.15607/RSS.2019.XV.040
Prágr, M., Čížek, P., Faigl, J.: Cost of transport estimation for legged robot based on terrain features inference from aerial scan. In: IEEE/RSJ International Conference on Intelligent Robots and Systems (IROS), pp. 1745–1750 (2018). https://doi.org/10.1109/IROS.2018.8593374
Prágr, M., Čížek, P., Faigl, J.: Incremental learning of traversability cost for aerial reconnaissance support to ground units. In: Mazal, J. (ed.) MESAS 2018. LNCS, vol. 11472, pp. 412–421. Springer, Cham (2019). https://doi.org/10.1007/978-3-030-14984-0_30
Rossi, G., Tanteri, L., Tofani, V., Vannocci, P., Moretti, S., Casagli, N.: Multitemporal UAV surveys for landslide mapping and characterization. Landslides 15(5), 1045–1052 (2018). https://doi.org/10.1007/s10346-018-0978-0
Sofman, B., Lin, E., Bagnell, J.A., Cole, J., Vandapel, N., Stentz, A.: Improving robot navigation through self-supervised online learning. J. Field Robot. 23(11–12), 1059–1075 (2006). https://doi.org/10.1002/rob.20169
Soleimani, B., Ashtiani, M.Z., Soleimani, B.H., Moradi, H.: A Disaster invariant feature for localization. In: IEEE/RSJ International Conference on Intelligent Robots and Systems (IROS), pp. 1096–1101 (2010). https://doi.org/10.1109/IROS.2010.5651930
Stelzer, A., Hirschmüller, H., Görner, M.: Stereo-vision-based navigation of a six-legged walking robot in unknown rough terrain. Int. J. Robot. Res. 31(4), 381–402 (2012). https://doi.org/10.1177/0278364911435161
Vijayakumar, S., Schaal, S.: Locally weighted projection regression: an o(n) algorithm for incremental real time learning in high dimensional space. In: International Conference on International Conference on Machine Learning (ICML), pp. 1079–1086 (2000)
Acknowledgement
The presented work has been supported under the OP VVV funded project CZ.02.1.01/0.0/0.0/16_019/0000765 “Research Center for Informatics”. The support under grant No. SGS19/176/OHK3/3T/13 to Miloš Prágr and Petr Váňa is also gratefully acknowledged.
Author information
Authors and Affiliations
Corresponding author
Editor information
Editors and Affiliations
Rights and permissions
Copyright information
© 2020 Springer Nature Switzerland AG
About this paper
Cite this paper
Prágr, M., Váňa, P., Faigl, J. (2020). Aerial Reconnaissance and Ground Robot Terrain Learning in Traversal Cost Assessment. In: Mazal, J., Fagiolini, A., Vasik, P. (eds) Modelling and Simulation for Autonomous Systems. MESAS 2019. Lecture Notes in Computer Science(), vol 11995. Springer, Cham. https://doi.org/10.1007/978-3-030-43890-6_1
Download citation
DOI: https://doi.org/10.1007/978-3-030-43890-6_1
Published:
Publisher Name: Springer, Cham
Print ISBN: 978-3-030-43889-0
Online ISBN: 978-3-030-43890-6
eBook Packages: Computer ScienceComputer Science (R0)