Abstract
Cholecystectomy and Appendectomy are the most frequent procedures in emergency general surgery. Emergency surgeries represent particularly important procedures in health care as they include various surgical specialties and represent a significant percentage of surgeries sustained in a hospital. This will have a reply is in terms of management of the resources that of costs supported from the hospital. The value of the average hospital stay, LOS, is an important parameter for the proper management of hospitals, providing support to clinicians. This study was conducted with the aim of predicting LOS for all patients undergoing appendectomy and cholecystectomy in the University Hospital “San Giovanni di Dio e Ruggi d’Aragona” of Salerno (Italy) and the A.O.R.N. “Antonio Cardarelli” of Naples (Italy).
Access this chapter
Tax calculation will be finalised at checkout
Purchases are for personal use only
Similar content being viewed by others
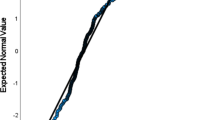
References
Akinbami, F., Askari, R., Steinberg, J., Panizales, M., Rogers, O.: Factors affecting morbidity in emergency general surgery. Am. J. Surg. 201(4), 456–462 (2011). https://doi.org/10.1016/j.amjsurg.2010.11.007. ISSN 0002-9610
Havens, J.M., et al.: The excess morbidity and mortality of emergency general surgery. J. Trauma Acute Care Surg. 78(2), 306–311 (2015). https://doi.org/10.1097/TA.0000000000000517
Becher, R.D., Hoth, J.J., Miller, P.R., Mowery, N.T., Chang, M.C., Meredith, J.W.: A critical assessment of outcomes in emergency versus nonemergency general surgery using the American college of surgeons national surgical quality improvement program (NSQIP) database. Am Surg. 77(7), 951–959 (2011)
Akinbami, F., Askari, R., Steinberg, J., Panizales, M., Rogers, S.O., Jr.: Factors affecting morbidity in emergency general surgery. Am J Surg. 201(4), 456–462 (2011)
Rosa, D., et al.: How to manage a failed cartilage repair: a systematic literature review. Joints 5(2), 93–106 (2017)
Smeraglia, F., Basso, M.A., Famiglietti, G., Eckersley, R., Bernasconi, A., Balato, G.: Partial wrist denervation versus total wrist denervation: a systematic review of the literature. Hand Surg. Rehabil. 39(6), 487–491 (2020)
Dimick, J.B., Chen, S.L., Taheri, P.A., et al.: Hospital costs associated with surgical complications: a report from the private-sector national surgical quality improvement program. J. Am. Coll. Surg. 199, 531–537 (2004)
Semm, K.: Endoscopic appendectomy. Endoscopy 15, 59–64 (1983). https://doi.org/10.1055/s-2007-1021466
Mayir, B., Bilecik, T., Ensari, C.O., Oruc, M.T.: Laparoscopic appendectomy with handmade loop. Videosurg. Miniinv. 9, 152–156 (2014). https://doi.org/10.5114/wiitm.2014.41624
Faiz, O., et al.: Traditional and laparoscopic appendectomy in adults. Ann. Surg. 248(5), 800–806 (2008). https://doi.org/10.1097/SLA.0b013e31818b770c
Strasberg, S.M.: Clinical practice. Acute calculous cholecystitis. N. Engl. J. Med. 358, 2804–2811 (2008). http://www.ncbi.nlm.nih.gov/pubmed/18579815
Yamashita, Y., et al.: Surgical treatment of patients with acute cholecystitis: Tokyo guidelines (2007)
McAleese, P., Odling-Smee, W.: The effect of complications on length of stay. Ann. Surg. 220(6), 740 (1994)
Scala, A., et al.: Regression models to study the total LOS related to valvuloplasty. Int. J. Environ. Res. Public Health 19, 3117 (2022). https://doi.org/10.3390/ijerph19053117
Achanta, A., et al.: Most of the variation in length of stay in emergency general surgery is not related to clinical factors of patient care. J. Trauma Acute Care Surg. 87, 408–412 (2019)
Smeraglia, F., Del Buono, A., Maffulli, N.: Endoscopic cubital tunnel release: a systematic review. Br Med Bull. 116, 155–163 (2015)
Smeraglia, F., Tamborini, F., Garutti, L., Minini, A., Basso, M.A., Cherubino, M.: Chronic exertional compartment syndrome of the forearm: a systematic review. EFORT Open Rev. 6(2), 101–106 (2021)
Detsky, A.S., Stricker, S.C., Mulley, A.G., Thibault, G.E.: Prognosis, survival, and the expenditure of hospital resources for patients in an intensive-care unit. N. Engl. J. Med. 305, 667–672 (1981)
Smeraglia, F., Mariconda, M., Balato, G., Di Donato, S.L., Criscuolo, G., Maffulli, N.: Dubious space for Artelon joint resurfacing for basal thumb (trapeziometacarpal joint) osteoarthritis. A systematic review. Br. Med. Bull. 126(1), 79–84 (2018)
Di Girolamo, R., Esposito, C., Moscato, V., Sperlí, G.: Evolutionary game theoretical on-line event detection over tweet streams. Knowl.-Based Syst. 211, 106563 (2021). https://doi.org/10.1016/j.knosys.2020.106563
Sperlí, G.: A deep learning based community detection approach. In: Proceedings of the 34th ACM/SIGAPP Symposium on Applied Computing, pp. 1107–1110 (2019). https://doi.org/10.1145/3297280.3297574
De Santo, A., Galli, A., Gravina, M., Moscato, V., Sperlì, G.: Deep learning for HDD health assessment: an application based on LSTM. IEEE Trans. Comput. 71(1), 69–80 (2020). https://doi.org/10.1109/TC.2020.3042053
La Gatta, V., Moscato, V., Pennone, M., Postiglione, M., Sperlí, G.: Music recommendation via hypergraph embedding. IEEE Trans. Neural Netw. Learn. Syst. (2022). https://doi.org/10.1109/TNNLS.2022.3146968
Han, Q., Molinaro, C., Picariello, A., Sperli, G., Subrahmanian, V.S., Xiong, Y.: Generating fake documents using probabilistic logic graphs. IEEE Trans. Dependable Secure Comput. (2021). https://doi.org/10.1109/TDSC.2021.3058994
Improta, G., Scala, A., Trunfio, T.A., Guizzi, G.: Application of supply chain management at drugs flow in an Italian hospital district. J. Phys. Conf. Ser. 1828(1) (2021). https://doi.org/10.1088/1742-6596/1828/1/012081
Di Laura, D., D’Angiolella, L., Mantovani, L., et al.: Efficiency measures of emergency departments: an Italian systematic literature review. BMJ Open Qual. 10, e001058 (2021). https://doi.org/10.1136/bmjoq-2020-001058
Improta, G., et al.: Management of the diabetic patient in the diagnostic care pathway. In: Jarm, T., Cvetkoska, A., Mahnič-Kalamiza, S., Miklavcic, D. (eds.) EMBEC 2020. IP, vol. 80, pp. 784–792. Springer, Cham (2021). https://doi.org/10.1007/978-3-030-64610-3_88
Ponsiglione, A.M., Amato, F., Cozzolino, S., Russo, G., Romano, M., Improta, G.: A hybrid analytic hierarchy process and likert scale approach for the quality assessment of medical education programs. Mathematics 10(9), 1426 (2022)
Cesarelli, G., Montella, E., Scala, A., Raiola, E., Triassi, M., Improta, G.: DMAIC approach for the reduction of healthcare-associated infections in the neonatal intensive care unit of the university hospital of Naples ‘Federico II.’ In: Jarm, T., Cvetkoska, A., Mahnič-Kalamiza, S., Miklavcic, D. (eds.) EMBEC 2020. IFMBE Proceedings, vol. 80, pp. 414–423. Springer, Cham (2021). https://doi.org/10.1007/978-3-030-64610-3_48
Raiola, E., et al.: Implementation of lean practices to reduce healthcare associated infections. Int. J. Healthc. Technol. Manag. 18, 51 (2020). https://doi.org/10.1504/IJHTM.2020.10039887
Ponsiglione, A.M., Cosentino, C., Cesarelli, G., Amato, F., Romano, M.: A comprehensive review of techniques for processing and analyzing fetal heart rate signals. Sensors 21(18), 6136 (2021)
Ponsiglione, A.M., Amato, F., Romano, M.: Multiparametric investigation of dynamics in fetal heart rate signals. Bioengineering 9, 8 (2022). https://doi.org/10.3390/bioengineering9010008
Improta, G., Mazzella, V., Vecchione, D., Santini, S., Triassi, M.: Fuzzy logic–based clinical decision support system for the evaluation of renal function in post-transplant patients. J. Eval. Clin. Pract. 26(4), 1224–1234 (2020)
Giovanni, I., Pasquale, N., Carmela, S.L., Maria, T.: Health worker monitoring: Kalman-based software design for fault isolation in human breathing. In: EMSS 2014 Proceedings (2014)
Balato, G., et al.: Laboratory-based versus qualitative assessment of α-defensin in periprosthetic hip and knee infections: a systematic review and meta-analysis. Arch. Orthop. Trauma Surg. 140(3), 293–301 (2019). https://doi.org/10.1007/s00402-019-03232-5
Balato, G., et al.: Bacterial biofilm formation is variably inhibited by different formulations of antibiotic-loaded bone cement in vitro. Knee Surg. Sports Traumatol. Arthrosc. 27(6), 1943–1952 (2018). https://doi.org/10.1007/s00167-018-5230-x
Rosa, D., Balato, G., Ciaramella, G., Soscia, E., Improta, G., Triassi, M.: Long-term clinical results and MRI changes after autologous chondrocyte implantation in the knee of young and active middle aged patients. J. Orthop. Traumatol. 17(1), 55–62 (2015). https://doi.org/10.1007/s10195-015-0383-6
Scala, A., Loperto, I., Carrano, R., Federico, S., Triassi, M., Improta, G.: Assessment of proteinuria level in nephrology patients using a machine learning approach. In: 2021 5th International Conference on Medical and Health Informatics (ICMHI 2021), pp. 13–16. Association for Computing Machinery, New York (2021). https://doi.org/10.1145/3472813.3472816
Santini, S., et al.: Using fuzzy logic for improving clinical daily-care of β-thalassemia patients. In 2017 IEEE International Conference on Fuzzy Systems (FUZZ-IEEE), pp. 1–6. IEEE (2017)
Latessa, I., et al.: Implementing fast track surgery in hip and knee arthroplasty using the lean six sigma methodology. TQM J. 33(7), 131–147 (2020)
De Franco, C., et al.: The active knee extension after extensor mechanism reconstruction using allograft is not influenced by “early mobilization”: a systematic review and meta-analysis. J. Orthop. Surg. Res. 17(1), 153 (2022)
Balato, G., et al.: Debridement and implant retention in acute hematogenous periprosthetic joint infection after knee arthroplasty: a systematic review. Orthop. Rev. (Pavia) 14(2), 33670 (2022)
Baldini, A., Balato, G., Franceschini, V.: The role of offset stems in revision knee arthroplasty. Curr. Rev. Musculoskelet. Med. 8(4), 383–389 (2015). https://doi.org/10.1007/s12178-015-9294-7
Scala, A., Trunfio, T.A., Borrelli, A., Ferrucci, G., Triassi, M., Improta, G.: Modelling the hospital length of stay for patients undergoing laparoscopic cholecystectomy through a multiple regression model. In: 2021 5th International Conference on Medical and Health Informatics (ICMHI 2021), pp. 68–72. Association for Computing Machinery, New York (2021). https://doi.org/10.1145/3472813.3472826
Combes, C., Kadri, F., Chaabane, S.: Predicting hospital length of stay using regression models: application to emergency department (2014)
Al Taleb, A.R., Hoque, M., Hasanat, A., Khan, M.B.: Application of data mining techniques to predict length of stay of stroke patients. In: 2017 International Conference on Informatics, Health Technology (ICIHT), pp. 1–5 (2017)
Bender, G.J., et al.: Neonatal intensive care unit: predictive models for length of stay. J. Perinatol. Off. J. Calif. Perinat. Assoc. 33, 147–153 (2013)
Bacchi, S., Tan, Y., Oakden-Rayner, L., Jannes, J., Kleinig, T., Koblar, S.: Machine learning in the prediction of medical inpatient length of stay. Intern. Med. J. 52, 176–185 (2022)
Trunfio, T.A., Scala, A., Borrelli, A., Sparano, M., Triassi, M., Improta, G.: Application of the lean six sigma approach to the study of the LOS of patients who undergo laparoscopic cholecystectomy at the San Giovanni di Dio and Ruggi d’Aragona University Hospital. In: 2021 5th International Conference on Medical and Health Informatics (ICMHI 2021), pp. 50–54. Association for Computing Machinery, New York (2021). https://doi.org/10.1145/3472813.3472823
Maria Ponsiglione, A., et al.: Modeling the variation in length of stay for appendectomy and cholecystectomy interventions in the emergency general surgery. In: 2021 International Symposium on Biomedical Engineering and Computational Biology (2021)
Guarino, F., Improta, G., Triassi, M., Castiglione, S., Cicatelli, A.: Air quality biomonitoring through Olea europaea L.: The study case of “Land of pyres.” Chemosphere 282, 131052 (2021). https://doi.org/10.1016/j.chemosphere.2021.131052
Guarino, F., Improta, G., Triassi, M., Cicatelli, A., Castiglione, S.: Effects of zinc pollution and compost amendment on the root microbiome of a metal tolerant poplar clone. Front. Microbiol. 11, 1677 (2020). https://doi.org/10.3389/fmicb.2020.01677
Guarino, F., Conte, B., Improta, G., Sciarrillo, R., Castiglione, S., Cicatelli, A., Guarino, C.: Genetic characterization, micropropagation, and potential use for arsenic phytoremediation of Dittrichia viscosa (L.) Greuter. Ecotoxicol. Environ. Saf. 148, 675–683 (2018). https://doi.org/10.1016/j.ecoenv.2017.11.010
Guarino, F., Cicatelli, A., Brundu, G., Improta, G., Triassi, M., Castiglione, S.: The use of MSAP reveals epigenetic diversity of the invasive clonal populations of Arundo donax L. PLoS One 14 (2019) https://doi.org/10.1371/journal.pone.0215096
De Agostini, A., et al.: Heavy metal tolerance of orchid populations growing on abandoned mine tailings: a case study in Sardinia Island (Italy). Ecotoxicol. Environ. Saf. 189, 110018 (2020). https://doi.org/10.1016/j.ecoenv.2019.110018
Moccia, E., et al.: Use of Zea mays L. in phytoremediation of trichloroethylene. Environ. Sci. Pollut. Res. 24, 11053–11060 (2017). https://doi.org/10.1007/s11356-016-7570-8
Smeraglia, F., Basso, M.A., Famiglietti, G., Cozzolino, A., Balato, G., Bernasconi, A.: Pyrocardan® interpositional arthroplasty for trapeziometacarpal osteoarthritis: a minimum four year follow-up. Int. Orthop. 46(8), 1803–1810 (2022)
Author information
Authors and Affiliations
Corresponding author
Editor information
Editors and Affiliations
Rights and permissions
Copyright information
© 2023 The Author(s), under exclusive license to Springer Nature Switzerland AG
About this paper
Cite this paper
Ponsiglione, A.M. et al. (2023). Analyzing LOS Variation for Patients Under Emergency Interventions: A Bicentric Study. In: Wen, S., Yang, C. (eds) Biomedical and Computational Biology. BECB 2022. Lecture Notes in Computer Science(), vol 13637. Springer, Cham. https://doi.org/10.1007/978-3-031-25191-7_42
Download citation
DOI: https://doi.org/10.1007/978-3-031-25191-7_42
Published:
Publisher Name: Springer, Cham
Print ISBN: 978-3-031-25190-0
Online ISBN: 978-3-031-25191-7
eBook Packages: Computer ScienceComputer Science (R0)