Abstract
Lung cancer, which is the leading cause of cancer-related death worldwide and is characterized by genetic changes and heterogeneity, presents a significant treatment challenge. Existing approaches utilizing Machine Learning (ML) techniques for identifying driver modules lack specificity, particularly for lung cancer. This study addresses this limitation by proposing a novel method that combines gene-gene interaction network construction with ML-based clustering to identify lung cancer-specific driver modules. The methodology involves mapping biological processes to genes and constructing a weighted gene-gene interaction network to identify correlations within gene clusters. A clustering algorithm is then applied to identify potential cancer-driver modules, focusing on biologically relevant modules that contribute to lung cancer development. The results highlight the effectiveness and robustness of the clustering approach, identifying 110 unique clusters ranging in size from 4 to 10. These clusters surpass evaluation requirements and demonstrate significant relevance to critical cancer-related pathways. The identified driver modules hold promise for influencing future approaches to lung cancer diagnosis, prognosis, and treatment. This research expands our understanding of lung cancer and sets the stage for further investigations and potential clinical advancements.
Access this chapter
Tax calculation will be finalised at checkout
Purchases are for personal use only
Similar content being viewed by others
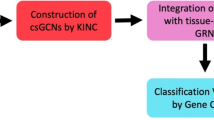
References
Hanahan, D., Weinberg, R.A.: Hallmarks of cancer: the next generation. Cell 144(5), 646–674 (2011)
Noone, A.M., Cronin, K.A., Altekruse, S.F., Howlader, N., et al.: Cancer incidence and survival trends by subtype using data from the surveillance epidemiology and end results program, 1992–2013. Cancer Epidemiol. Biomark. Prev. 26(4), 632–41 (2017)
Ridge, C.A., McErlean, A.M., Ginsberg, M.S.: Seminars in Interventional Radiology, pp. 093–098. Thieme Medical Publishers (2013)
Thun, M.J., Hannan, L.M., Adams-Campbell, L.L., Boffetta, P., et al.: Lung cancer occurrence in never-smokers: an analysis of 13 cohorts and 22 cancer registry studies. PLoS Med. 5(9), e185 (2008)
Cruz, C.S., Tanoue, L.T., Matthay, R.A.: Lung cancer: epidemiology, etiology, and prevention. Clin. Chest Med. 32(4), 605–44 (2011)
Pikor, L.A., Ramnarine, V.R., Lam, S., Lam, W.L.: Genetic alterations defining NSCLC subtypes and their therapeutic implications. Lung Cancer 82(2), 179–89 (2013)
Chen, Z., Fillmore, C.M., Hammerman, P.S., Kim, C.F., Wong, K.K.: Non-small-cell lung cancers: a heterogeneous set of diseases. Nat. Rev. Cancer 14(8), 535–46 (2014)
Lung Cancer Modules Repository. https://github.com/Golnazthr/LungCancerModules
Cancer Genome Atlas (TCGA) Research Network: Comprehensive genomic characterization defines human glioblastoma genes and core pathways. Nature 455(7216), 1061 (2008)
Vogelstein, B., Papadopoulos, N., Velculescu, V.E., Zhou, S., Diaz, L.A., Jr., Kinzler, K.W.: Cancer genome landscapes. Science 339(6127), 1546–1558 (2013)
Taheri, G., Habibi, M.: Using unsupervised learning algorithms to identify essential genes associated with SARS-CoV-2 as potential therapeutic targets for COVID-19. bioRxiv 5(1) (2022)
Taheri, G., Habibi, M.: Identification of essential genes associated with SARS-CoV-2 infection as potential drug target candidates with machine learning algorithms. Sci. Rep. 13(1), 15141 (2023)
Dopazo, J., Erten, C.: Graph-theoretical comparison of normal and tumor networks in identifying BRCA genes. BMC Syst. Biol. 1(11), 1–7 (2017)
Yang, H., Wei, Q., Zhong, X., Yang, H., Li, B.: Cancer driver gene discovery through an integrative genomics approach in a non-parametric Bayesian framework. Bioinformatics 33(4), 483–90 (2017)
Vanunu, O., Magger, O., Ruppin, E., Shlomi, T., Sharan, R.: Associating genes and protein complexes with disease via network propagation. PLoS Comput. Biol. 6(1), e1000641 (2010)
Deng, Y., Luo, S., Deng, C., Luo, T., Yin, W., Zhang, H., et al.: Identifying mutual exclusivity across cancer genomes: computational approaches to discover genetic interaction and reveal tumor vulnerability. Brief. Bioinform. 20(1), 254–266 (2019)
Zhang, J., Zhang, S.: The discovery of mutated driver pathways in cancer: models and algorithms. IEEE/ACM Trans. Comput. Biol. Bioinf. 15(3), 988–998 (2018)
Ciriello, G., Cerami, E., Sander, C., Schultz, N.: Mutual exclusivity analysis identifies oncogenic network modules. Genome Res. 22(2), 398–406 (2012)
Cho, A., Shim, J.E., Kim, E., Supek, F., Lehner, B., Lee, I.: MUFFINN: cancer gene discovery via network analysis of somatic mutation data. Genome Biol. 17(1), 1–6 (2016)
Dimitrakopoulos, C.M., Beerenwinkel, N.: MUFFINN: computational approaches for the identification of cancer genes and pathways. Syst. Biol. Med. 9(1), e1364 (2017)
Zhang, W., Wang, S.L., Liu, Y.: Identification of cancer driver modules based on graph clustering from multiomics data. J. Comput. Biol. 28(10), 1007–1020 (2021)
Habibi, M., Taheri, G.: Topological network based drug repurposing for Coronavirus 2019. PLoS ONE 16(7), e0255270 (2021)
Habibi, M., Taheri, G.: A new machine learning method for cancer mutation analysis. PLoS Comput. Biol. 18(10), e1010332 (2022)
Traag, V.A., Waltman, L., Van Eck, N.J.: From Louvain to Leiden: guaranteeing well-connected communities. Sci. Rep. 9(1), 5233 (2019)
Spirin, V., Mirny, L.A.: Protein complexes and functional modules in molecular networks. In: Proceedings of the National Academy of Sciences, pp. 12123–12128 (2010)
Taheri, G., Habibi, M., Wong, L., Eslahchi, C.: Disruption of protein complexes. J. Bioinform. Comput. Biol. 11(03), 1341008 (2013)
Ahmad, A.: Breast Cancer Metastasis and Drug Resistance: Challenges and Progress. Springer (2019)
Taheri, G., Habibi, M.: A novel machine learning method for mutational analysis to identifying driver genes in breast cancer. bioRxiv 11(01), 1341008 (2022)
Stabile, L.P., Siegfried, J.M.: Estrogen receptor pathways in lung cancer. Curr. Oncol. Rep. 6(01), 259–267 (2004)
Taheri, G., Habibi, M.: Comprehensive analysis of pathways in Coronavirus 2019 (COVID-19) using an unsupervised machine learning method. Appl. Soft Comput. 128, 109510 (2022)
Ekman, S., Wynes, M.W., Hirsch, F.R.: The mTOR pathway in lung cancer and implications for therapy and biomarker analysis. J. Thorac. Oncol. 7(06), 947–953 (2012)
Hao, X.L., Han, F., Zhang, N., Chen, H.Q., et al.: TC2N, a novel oncogene, accelerates tumor progression by suppressing p53 signaling pathway in lung cancer. Cell Death Differ. 26(7), 1235–1250 (2019)
Frezzetti, D., Gallo, M., Maiello, M.R., D’Alessio, A., Esposito, C., et al.: EGF as a potential target in lung cancer. Expert Opin. Ther. Targets 21(10), 959–66 (2017)
Stewart, D.J.: Wnt signaling pathway in non-small cell lung cancer. J. Natl. Cancer Inst. 106(1), 1–11 (2014)
Author information
Authors and Affiliations
Corresponding author
Editor information
Editors and Affiliations
Rights and permissions
Copyright information
© 2025 The Author(s), under exclusive license to Springer Nature Switzerland AG
About this paper
Cite this paper
Taheri, G., Szalai, M., Habibi, M., Papapetrou, P. (2025). Unveiling Driver Modules in Lung Cancer: A Clustering-Based Gene-Gene Interaction Network Analysis. In: Meo, R., Silvestri, F. (eds) Machine Learning and Principles and Practice of Knowledge Discovery in Databases. ECML PKDD 2023. Communications in Computer and Information Science, vol 2136. Springer, Cham. https://doi.org/10.1007/978-3-031-74640-6_4
Download citation
DOI: https://doi.org/10.1007/978-3-031-74640-6_4
Published:
Publisher Name: Springer, Cham
Print ISBN: 978-3-031-74639-0
Online ISBN: 978-3-031-74640-6
eBook Packages: Artificial Intelligence (R0)