Abstract
Colorectal cancer is a diagnosis of particular concern for older Canadians. Treatment of colorectal cancer requires a complex decision-making process of treatment. These treatments may involve surgery and either pre- or post-operative radiation or chemotherapy, which can have a great impact on the quality of life of patients due to the rigorous requirements of treatment and the inconvenient side effects. The conceptual and architectural modelling is challenging due to the diverse and complex dimensions. In this chapter we have proposed a modelling approach based on an additional structure to simplify the design of simulations. The modelling approach considers the complexity of the modelling process, where in the various models are developed. We developed a computer simulation environment of patient care trajectories using the agent in order to evaluate new approaches to increase hospital productivity and adapt hospital clinical practice conditions for the elderly and patients with multiple chronic diseases. So, we have developed a multi-agent framework to simulate the activities and roles in a Health Care (HC) system. This framework can be used to assist the collaborative scheduling of complex tasks that involve multiple personals and resources. In addition, it can be used to study the efficiency of the HC system and the influence of different policies.
Access this chapter
Tax calculation will be finalised at checkout
Purchases are for personal use only
Similar content being viewed by others
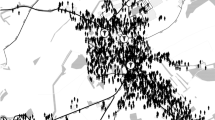
References
Barbolosi, D., Verga, F., You, B., Benabdallah, A., Hubert, F., Mercier, C.: Modélisation du risque d’évolution métastatique chez les patients supposés avoir une maladie localisée. Oncologie 13, 528–533 (2011)
Bolin, S., Nilsson, E., Sjödahl, R.: Carcinoma of the colon and rectum–growth rate. Ann. Surg. 198, 151 (1983)
Chao, S., Wong, F.: A multi-agent learning paradigm for medical data mining diagnostic workbench (2009)
Claret, L., Girard, P., Hoff, P.M., Van Cutsem, E., Zuideveld, K.P., Jorga, K., et al.: Model-based prediction of phase III overall survival in colorectal cancer on the basis of phase II tumor dynamics. J. Clin. Oncol. 27, 4103–4108 (2009)
Devi, M.S., Mago, V.: Multi-agent model for Indian rural health care. Leadersh. Health Serv. 18, 1–11 (2005)
Foster, D., McGregor, C., El-Masri, S.: A survey of agent-based intelligent decision support systems to support clinical management and research. In: Proceedings of the 2nd International Workshop on Multi-agent Systems for Medicine, Computational Biology, and Bioinformatics, pp. 16–34 (2005)
Figueredo, G.P., Aickelin, U.: Comparing system dynamics and agent-based simulation for tumour growth and its interactions with effector cells. In: Proceedings of the 2011 Summer Computer Simulation Conference, pp. 52–59 (2011)
Fujimoto, R.: Parallel and Distributed Simulation Systems. Wiley, Hoboken (2000)
Gilli, Q., Mustapha, K., Frayret, J.M., Lahrichi, N., Karimi, E.: Agent-Based Simulation of Colorectal Cancer Care Trajectory: Patient Model. CIRRELT (Interuniversity Research Centre on Enterprise Networks, Logistics and Transportation) (2014)
Gupta, S., Sarkar, A., Pramanik, I., Mukherjee, B.: Implementation scheme for online medical diagnosis system using multi agent system with JADE. Int. J. Sci. Res. Publ. 2(6) (2012). ISSN 2250-3153
Gyllenberg, M., Webb, G.F.: Quiescence as an explanation of Gompertzian tumor growth. Growth Dev. Aging: GDA 53, 25–33 (1988)
Gupta, S., Mukhopadhyay, S.: Multi agent system based clinical diagnosis system: an algorithmic approach. Int. J. Eng. Res. Appl. (IJERA) 2(5), 1474–1477 (2012). ISSN: 2H8-9622
Gupta, S., Pujari, S.: A multi-aged: based scheme for heath care and clinical diagnosis system. In: IAMA (2009). IEEExplore. ISBN 978-1-4244-4710-7
Han, B.-M., Song, S.-J., Lee, K.M., Kyung-Soo, J., Dong-Ryeol, S.: Multi agent system based efficient healthcare service. In: ICACT 2006, 20–24 February 2006. ISBN 89-551 9-1 29-4
Heun, J.M., Grothey, A., Branda, M.E., Goldberg, R.M., Sargent, D.J.: Tumor status at 12 weeks predicts survival in advanced colorectal cancer: findings from NCCTG N9741. Oncologist 16, 859–867 (2011)
Iantovics, B.: The CMDS medical diagnosis system. In: Ninth International Symposium on Symbolic and Numeric Algorithms for Scientific Computing. IEEE (2008)
Iwata, K., Kawasaki, K., Shigesada, N.: A dynamical model for the growth and size distribution of multiple metastatic tumors. J. Theor. Biol. 203, 177–186 (2000)
Jennings, N.R., Sycara, K., Wooldridge, M.: A roadmap of agent research and development. Auton. Agent. Multi-agent Syst. 1, 7–38 (1998)
Jones, S.S., Evans, R.S.: An agent based simulation tool for scheduling emergency department physicians. In: AMIA Annual Symposium Proceedings, p. 338 (2008)
Kanagarajah, A., Parker, D., Xu, H.: Health care supply networks in tightly and loosely coupled structures: exploration using agent-based modelling. Int. J. Syst. Sci. 41, 261–270 (2010)
Kazar, O., Sahnoun, Z., Frecon, L.: Multi-agents system for medical diagnosis. In: International Conference on Intelligent System and Knowledge Engineering, vol. 1, pp. 1265–1270 (2008)
Klusch, M., Lodi, S., Moro, G.: The role of agents in distributed data mining - issues and benefits. In: Proceedings of IEEE/WIC International Conference on Intelligent Agent Technology (IAT 2003), pp. 211–217 (2003)
Knight, V.A., Williams, J.E., Reynolds, I.: Modelling patient choice in healthcare systems: development and application of a discrete event simulation with agent-based decision making. J. Simul. 6, 92–102 (2012)
Krizmaric, M., Zmauc, T., Micetic-Turk, D., Stiglic, G., Kokol, P.: Time allocation simulation model of clean and dirty pathways in hospital environment. In: Proceedings of 18th IEEE Symposium on Computer-Based Medical Systems, pp. 123–127 (2005)
Laskowski, M., McLeod, R.D., Friesen, M.R., Podaima, B.W., Alfa, A.S.: Models of emergency departments for reducing patient waiting times. PLoS ONE 4, e6127 (2009)
Mahmud, R., Sithiq, H.A.A.H., Taharim, H.M.: A Hybrid Technology for a Multi agent Consultation System in Obesity Domain. World Academy of Science, Engineering and Technology (2009)
Mustafee, N., Katsaliaki, K., Taylor, S.J.E.: Profiling literature in healthcare simulation. Simulation 86, 543–558 (2010)
Nealon, J., Moreno, A.: Agent-based applications in health care. In: Applications of Software Agent Technology in the Health Care Domain, pp. 3–18. Springer (2003)
Quesnel, G., Duboz, R., Ramat, E., Traoré, M.K.: VLE: a multimodeling and simulation environment. In: Proceedings of the Summer Simulation Multiconference (SummerSim 2007), San Diego, California, USA, pp. 367–374, 15–18 July 2007
Rosa, M.V., Flores, C.D., Silvestre, A.M., Seixas, L.J., Ladeira, M., Coelho, H.: A multi agent intelligent environment for medical knowledge. Artif. Intell. Med. 27, 335–366 (2003)
Rimassa, G., Bellifemine, F., Poggi, A.: JADE - a FIPA compliant agent framework. In: PMAA 1999, Londres, pp. 97–108 (1999)
Canadian Cancer Society: Subcutaneous port. http://www.cancer.ca/en/cancer-information/diagnosis-and-treatment/tests-and-procedures/subcutaneous-port/?region=on
Canadian Cancer Society: Peripherally inserted central catheter. http://www.cancer.ca/en/cancer-information/diagnosis-and-treatment/tests-and-procedures/peripherally-inserted-central-catheter/?region=on
Stainsby, H., Taboada, M., Luque, E.: Towards an agent-based simulation of hospital emergency departments. In: SCC IEEE International Conference on Services Computing, Bangalore, India, 21–25 September 2009, pp. 536–539 (2009)
Suwinski, R., Wzietek, I., Tarnawski, R., Namysl-Kaletka, A., Kryj, M., Chmielarz, A.: Moderately low alpha/beta ratio for rectal cancer may best explain the outcome of three fractionation schedules of preoperative radiotherapy. Int. J. Radiat. Oncol. Biol. Phys. 69, 793–799 (2007)
Verga, F.: Modélisation mathématique de processus métastatiques, Université de Provence-Aix-Marseille I (2010)
Van Cutsem, E., Findlay, M., Osterwalder, B., Kocha, W., Dalley, D., Pazdur, R., et al.: Capecitabine, an oral fluoropyrimidine carbamate with substantial activity in advanced colorectal cancer: results of a randomized phase II study. J. Clin. Oncol. 18, 1337–1345 (2000)
Wang, P., Feng, Y.: A mathematical model of tumor volume changes during radiotherapy. Sci. World J. 2013 (2013)
Zhang, W., Yao, Z.: A reformed lattice gas model and its application in the simulation of evacuation in hospital fire. In: IEEE International Conference on Industrial Engineering and Engineering Management, IEEM 2010, Macao, China, pp. 1543–1547, 7 December 2010
Zhang, C., Cao, L.: Agents and data mining: mutual enhancement by integration. autonomous. In: Gorodetsky, V., Liu, J., Skormin, V.A. (eds.) Autonomous Intelligent Systems: Agents and Data Mining, pp. 50–61. Springer, Heidelberg (2005)
Author information
Authors and Affiliations
Corresponding author
Editor information
Editors and Affiliations
Rights and permissions
Copyright information
© 2018 Springer International Publishing AG
About this paper
Cite this paper
Mustapha, K., Gilli, Q., Frayret, JM., Lahrichi, N. (2018). Agent-Based Modelling and Simulation Framework for Health Care. In: Obaidat, M., Ören, T., Merkuryev, Y. (eds) Simulation and Modeling Methodologies, Technologies and Applications. SIMULTECH 2016. Advances in Intelligent Systems and Computing, vol 676. Springer, Cham. https://doi.org/10.1007/978-3-319-69832-8_11
Download citation
DOI: https://doi.org/10.1007/978-3-319-69832-8_11
Published:
Publisher Name: Springer, Cham
Print ISBN: 978-3-319-69831-1
Online ISBN: 978-3-319-69832-8
eBook Packages: EngineeringEngineering (R0)