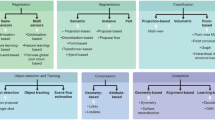
Overview
- The first comprehensive book on deep learning-based 3D point cloud technologies
- Illustrating 3D pre-trained and large models, multi-modal learning
- Discussions on the open source projects and engineering applications of 3D point clouds
Access this book
Tax calculation will be finalised at checkout
Other ways to access
About this book
As an efficient 3D vision solution, point clouds have been widely applied into diverse engineering scenarios, including immersive media communication, autonomous driving, reverse engineering, robots, topography mapping, digital twin city, medical analysis, digital museum, etc. Thanks to the great developments of deep learning theories and methods, 3D point cloud technologies have undergone fast growth during the past few years, including diverse processing and understanding tasks. Human and machine perception can be benefited from the success of using deep learning approaches, which can significantly improve 3D perception modeling and optimization, as well as 3D pre-trained and large models. This book delves into these research frontiers of deep learning-based point cloud technologies.
The subject of this book focuses on diverse intelligent processing technologies for the fast-growing 3D point cloud applications, especially using deep learning-based approaches. The deep learning-based enhancement and analysis methods are elaborated in detail, as well as the pre-trained and large models with 3D point clouds. This book carefully presents and discusses the newest progresses in the field of deep learning-based point cloud technologies, including basic concepts, fundamental background knowledge, enhancement, analysis, 3D pre-trained and large models, multi-modal learning, open source projects, engineering applications, and future prospects.
Readers can systematically learn the knowledge and the latest developments in the field of deep learning-based point cloud technologies. This book provides vivid illustrations and examples, and the intelligent processing methods for 3D point clouds. Readers can be equipped with an in-depth understanding of the latest advancements of this rapidly developing research field.
Similar content being viewed by others
Keywords
Table of contents (11 chapters)
Authors and Affiliations
About the authors
Dr. Wei Gao is an assistant professor at the School of Electronic and Computer Engineering, Peking University, Shenzhen, China. His research interests include 3D point cloud compression and processing, image/video coding and processing, deep learning, and artificial intelligence. He actively participates in standardization activities for multimedia compression and leads the development of the open source project for point cloud technologies, namely OpenPointCloud. He is a senior member of IEEE. He has authored the book “Point Cloud Compression - Technologies and Standardization” published by Springer Nature.
Dr. Ge Li is a professor at the School of Electronic and Computer Engineering, Peking University, Shenzhen, China. He chairs the standardization of 3D point cloud compression in the Audio Video Coding Standard (AVS) working group in China. His research interests include 3D point cloud compression and processing, image/video processing and analysis, machine learning, and signal processing. He has authored the book “Point Cloud Compression - Technologies and Standardization” published by Springer Nature.
Bibliographic Information
Book Title: Deep Learning for 3D Point Clouds
Authors: Wei Gao, Ge Li
DOI: https://doi.org/10.1007/978-981-97-9570-3
Publisher: Springer Singapore
eBook Packages: Computer Science, Computer Science (R0)
Copyright Information: The Editor(s) (if applicable) and The Author(s), under exclusive license to Springer Nature Singapore Pte Ltd. 2025
Hardcover ISBN: 978-981-97-9569-7Published: 07 December 2024
Softcover ISBN: 978-981-97-9572-7Due: 21 December 2025
eBook ISBN: 978-981-97-9570-3Published: 06 December 2024
Edition Number: 1
Number of Pages: XVI, 322
Number of Illustrations: 20 b/w illustrations, 98 illustrations in colour
Topics: Image Processing and Computer Vision, Computer Imaging, Vision, Pattern Recognition and Graphics, Computer Graphics, Signal, Image and Speech Processing, Coding and Information Theory