Abstract
In this paper, a highly effective Bayesian sampling algorithm based on auxiliary variables is used to estimate the graded response model with non-ignorable missing response data. Compared with the traditional marginal likelihood method and other Bayesian algorithms, the advantages of the new algorithm are discussed in detail. Based on the Markov Chain Monte Carlo samples from the posterior distributions, the deviance information criterion and the logarithm of the pseudomarignal likelihood are employed to compare the different missing mechanism models. Two simulation studies are conducted and a detailed analysis of the sexual compulsivity scale data is carried out to further illustrate the proposed methodology.





Similar content being viewed by others
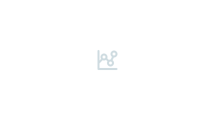
References
Ackerman TA (1996) Developments in multidimensional item response theory. Appl Psychol Measure 20:309–310
Ackerman TA (1996) Graphical representation of multidimensional item response theory analyses. Appl Psychol Measure 20:311–329
Albert JH (1992) Bayesian estimation of normal ogive item response curves using Gibbs sampling. J Edu Stat 17:251–269
Andrich D (1978) Application of a psychometric rating model to ordered categories which are scored with successive integers. Appl Psychol Measure 2:581–594
Baker FB (1985) The basics of item response theory. Heinemann, Portsmouth, NH
Baker FB (1992) Item response theory: parameter estimation techniques. Marcel Dekker, New York
Béguin AA, Glas CAW (2001) MCMC estimation of multidimensional IRT models. Psychometrika 66:541–561
Bjorner JB, Kosinski M, Ware KJE Jr (2003) Calibration of an item pool for assessing the burden of headaches: an application of item response theory to the Headache Impact Test (HIT\(^{\rm TM}\) 453). Qual Life Res 12:913–933
Bock RD (1972) Estimating item parameters and latent ability when responses are scored in two or more nominal categories. Psychometrika 37:29–51
Bock RD, Aitkin M (1981) Marginal maximum likelihood estimation of item parameters: Application of an EM algorithm. Psychometrika 46:443–459
Bolt DM, Hare RD, Vitale JE, Newman JP (2004) A multigroup item response theory analysis of the psychopathy checklist-revised. Psychol Assess 16:155–168
Brooks SP, Gelman A (1998) Alternative methods for monitoring convergence of iterative simulations. J Comput Graph Stat 7:434–455
Brooks S, Gelman A, Jones G, Meng XL (2011) Handbook of Markov chain Monte Carlo. CRC Press, Boca Raton
Carpita M, Manisera M (2011) On the imputation of missing data in surveys with Likert-Type Scales. J Class 28:93–112
Chen M-H, Shao Q-M, Ibrahim JG (2000) Monte Carlo Methods in Bayesian Computation. Springer, New York
Chib S, Greenberg E (1995) Understanding the metropolis-hastings algorithm. Am Stat 49:327–335
Fox JP (2005) Multilevel IRT using dichotomous and polytomous items. Br J Math Stat Psychol 58:145–172
Fox J-P (2010) Bayesian item response modeling: Theory and applications. Springer, New York, NY
Fox J-P, Glas CAW (2001) Bayesian estimation of a multilevel IRT model using Gibbs sampling. Psychometrika 66:269–286
Geisser S, Eddy WF (1979) A predictive approach to model selection. J Am Stat Assoc 74:153–160
Gelfand AE, Dey DK, Chang H (1992) Model determination using predictive distributions with implementation via sampling-based methods (with discussion). In: Bernardo JM, Berger JO, Dawid AP, Smith AFM (eds) Bayesian statistics 4. Oxford University Press, Oxford, UK, pp 147–167
Gelfand AE, Smith AFM (1990) Sampling-based approaches to calculating marginal densities. J Am Stat Assoc 85:398–409
Gelman A, Rubin DB (1992) Inference from iterative simulation using multiple sequences. Stat Sci 7:457–472
Geman S, Geman D (1984) Stochastic relaxation, Gibbs distributions, and the Bayesian restoration of images. IEEE Trans Pattern Anal Mach Intell 6:721–741
Ghosh M, Ghosh A, Chen M, Agresti A (2000) Noninformative priors for one parameter item response models. J Stat Plan Inference 88(486):99–115
Glas CAW, Pimentel JL (2008) Modeling nonignorable missing data in speeded tests. Edu Psychol Measure 68:907–922
Glas CAW, Pimentel JL, Lamers MA (2015) Nonignorable data in IRT mdoels: polytomous response and response propensity models with covariates. Psychol Test Assess Model 57:523–541
Hastings WK (1970) Monte Carlo sampling methods using Markov chains and their applications. Biometrika 57:97–109
Heckman J (1976) The common structure of statistical models of truncation, sample selection and limited dependent variables and a simple estimator for such models. Anna Econo Soc Measure 5:475–492
Heckman J (1979) Sample selection bias as a specification error. Econometrica 47:153–61
Hoffman MD, Gelman A (2014) The No-U-turn sampler: adaptively setting path lengths in Hamiltonian Monte Carlo. J Mach Learn Res 15:1593–1623
Holman R, Glas CAW (2005) Modelling non-ignorable missing-data mechanisms with item response theory models. Br J Math Stat Psychol 58:1–17
Huisman M (2000) Imputation of missing item responses: Some simple techniques. Qual Quant 34:331–351
Ibrahim JG, Chen M-H, Sinha D (2001) Bayesian Survival Analysis. Springer, New York
Jiang ZH, Templin J (2019) Gibbs samplers for logistic item response models via the pólya-gamma distribution: a computationally efficient data-augmentation strategy. Psychometrika 84:358–374
Kalichman SC, Rompa D (1995) Sexual sensation seeking and sexual compulsivity scales: validity, and predicting hiv risk behavior. J Personal Assess 65:586–601
Korobko OK, Glas CAW, Bosker RJ, Luyten JW (2008) Comparing the difficulty of examination subjects with item response theory. J Edu Measure 45:137–155
Likert R (1932) A technique for the measurement of attitudes. Arch Psychol 22:1–55
Little RJA (1993) Pattern-mixture models for multivariate incomplete data. J Am Stat Assoc 88:125–134
Little RJA, Rubin DB (2002) Statistical analysis with missing data, 2nd edn. Wiley, New York
Lord FM (1983) Maximum likelihood estimation of item response parameters when some responses are omitted. Psychometrika 48:477–482
Lord FM, Novick MR (1968) Statistical Theories of Mental Test Scores. Addison-Wesley, Reading, MA
Lu J, Zhang JW, Tao J (2018) Slice-Gibbs sampling algorithm for estimating the parameters of a multilevel item response model. J Math Psychol 82:12–25
Masters GN (1982) A Rasch model for partial credit scoring. Psychometrika 47:149–174
Metropolis N, Rosenbluth AW, Rosenbluth MN, Teller AH, Teller E (1953) Equation of state calculations by fast computing machines. J Chem Phys 21:1087–1092
Moustaki I, Knott M (2000) Weighting for item non-response in attitude scales by using latent variable models with covariates. J R Stat Soc Series A 163:445–459
Muraki E (1992) Fitting a polytomous item response model to Likert-type data. Appl Psychol Measure 14:59–71
Natesan P, Limbers C, Varni JW (2010) Bayesian estimation of graded response multilevel models using gibbs sampling: formulation and illustration. Edu Psychol Measure 70:420–439
Neal RM (2003) Slice sampling. Ann Stat 31:705–767
O’Muircheartaigh C, Moustaki I (1999) Symmetric pattern models: a latent variable approach to item non-response in attitudes scales. J R Stat Soc 162:177–194
Reckase MD (1985) The difficulty of test items that measure more than one ability. Appl Psychol Measure 9:401–412
Reckase MD (1997) A linear logistic multidimensional model for dichotomous item response data. In: van der Linden WJ, Hambleton RK (eds) Handbook of modern item response theory. Springer-Verlag, New York
Rose, N. (2013). Item nonresponses in educational and psychological measurement. Doctoral Thesis, Friedrich-Schiller University, Jena
Rose, N., von Davier, M., & Xu, X. (2010). Modeling nonignorable missing data with IRT. Research Report No. RR-10-11. Princeton, NJ: Educational Testing Service
Rubin DB (1976) Inference and missing data. Biometrika 63:581–592
Samejima, F. (1969). Estimation of latent ability using a response pattern of graded scores (Psychometric Monograph No. 17). Richmond, VA: Psychometric Society. Retrieved from http://www.psychometrika.org/journal/online/MN17.pdf
Schafer JL (1997) Analysis of incomplete multivariate data. Chapman & Hall, London
Spiegelhalter DJ, Best NG, Carlin BP, Van Der Linde A (2002) Bayesian measures of model complexity and fit. J R Stat Soc Series B 64:583–639
Tanner MA, Wong WH (1987) The calculation of posterior distributions by data augmentation. J Am Stat Assoc 82:528–550
Tutz G (1990) Sequential item response models with an ordered response. Br J Math Stat Psychol 43:39–55
Tutz G (1991) Sequential models in categorical regression. Comput Stat Data Anal 11:275–295
Author information
Authors and Affiliations
Corresponding author
Additional information
Publisher's Note
Springer Nature remains neutral with regard to jurisdictional claims in published maps and institutional affiliations.
Supplementary Information
Below is the link to the electronic supplementary material.
Rights and permissions
About this article
Cite this article
Zhang, J., Zhang, Z. & Tao, J. A Bayesian algorithm based on auxiliary variables for estimating GRM with non-ignorable missing data. Comput Stat 36, 2643–2669 (2021). https://doi.org/10.1007/s00180-021-01100-8
Received:
Accepted:
Published:
Issue Date:
DOI: https://doi.org/10.1007/s00180-021-01100-8