Abstract
Skin diseases are the most important worrying problems in societies because it affects the patients both physically and psychologically. Skin disease is one of the highly prone to risk with an association of climatic factors around the world. Predicting the skin disease cases associated with influencing factors is the most crucial task. It is very difficult task to identify the appropriate and optimal features for skin disease from the large volume of health sector data available in the world. Previous researchers applied different types of ensemble features selection techniques for the appropriate selection of features which gives highest accuracy with minimum computation time. Classification rate of any algorithm depends on feature extraction techniques and classifier used for classification purpose. Data availability is one of the most significant drawbacks in the health sector if data are available that might be in raw format. Filling missing value and type conversion almost takes 70% of the time. The missing value can be addressed by statistical parameters such as mean, average and median with stand mechanism in machine learning. The objective of this paper is the selection of significant attributes and removes irrelevant features that affect model performance. The performance of skin disease data can be experimented through K-nearest neighbor, support vector machine and random forest classifier. The entire research is carried out on the real-time dataset. The efficiency of the proposed approach is measured through confusion matrix, accuracy, F-measure, precision and recall.
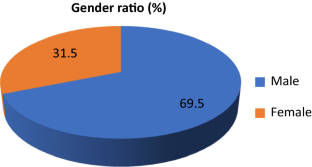


Similar content being viewed by others
Explore related subjects
Discover the latest articles, news and stories from top researchers in related subjects.Availability of data and material
Not applicable.
Change history
15 July 2024
This article has been retracted. Please see the Retraction Notice for more detail: https://doi.org/10.1007/s00500-024-09959-7
References
Ahn G, Hur S (2020) Efficient genetic algorithm for feature Selection for early time series classification. Comput Ind Eng 142:106345
Amuakwa-Mensah F, Marbuah G, Mubanga M (2017) Climate variability and infectious diseases nexus: Evidence from Sweden. Infect Dis Model 2(2):203–217. https://doi.org/10.1016/j.idm.2017.03.003
Azimi F, Shirian S, Jangjoo S, Ai A, Abbasi T (2017) Impact of climate variability on the occurrence of cutaneous leishmaniasis in Khuzestan Province, southwestern Iran. Geospatial Health. https://doi.org/10.4081/gh.2017.478
Bacanin N, Bezdan T, Venkatachalam K, Al-Turjman F (2021) Optimized convolutional neural network by firefly algorithm for magnetic resonance image classification of glioma brain tumor grade. J Real Time Image Process 18(4):1–14
Balk SJ (2011) Ultraviolet radiation: a hazard to children and adolescents. Pediatrics 127(3):e791–e817. https://doi.org/10.1542/peds.2010-3502
Cao P, Liu X, Liu H, Yang J, Zhao D, Huang M, Zaiane O (2018) Generalized fused group lasso regularized multi-task feature learning for predicting cognitive outcomes in Alzheimers disease. Comput Methods Programs Biomed 162:19–45. https://doi.org/10.1016/j.cmpb.2018.04.028
Cecchi L, D’Amato G, Annesi-Maesano I (2018) External exposome and allergic respiratory and skin diseases. J Allergy Clin Immunol 141(3):846–857. https://doi.org/10.1016/j.jaci.2018.01.016
Chalghaf B, Chemkhi J, Mayala B, Harrabi M, Benie GB, Michael E, Salah AB (2018) Ecological niche modeling predicting the potential distribution of Leishmania vectors in the mediterranean basin: impact of climate change. Parasit Vectors. https://doi.org/10.1186/s13071-018-3019-x
Chen J, Zhou S, Kang Z, Wen Q (2020) Locality-Constrained group lasso coding for microvessel image classification. Pattern Recogn Lett 130:132–138. https://doi.org/10.1016/j.patrec.2019.02.011
Combe M, Velvin CJ, Morris A, Garchitorena A, Carolan K, Sanhueza D, Roche B, Couppié P, Guégan JF, Gozlan RE (2017) Global and local environmental changes as drivers of Buruli ulcer emergence: Environmental changes and infectious disease. Emerg Microb Infect 6(1):1–11
Dayrit JF, Bintanjoyo L, Andersen LK, Mark DP (2018) Impact of climate change on dermatological conditions related to flooding: update from the international society of dermatology climate change committee. Int J Dermatol 57(8):901–910. https://doi.org/10.1111/ijd.13901
Jain PK, Pamula R, Srivastava G (2021) A systematic literature review on machine learning applications for consumer sentiment analysis using online reviews. Comput Sci Rev 41:100413. https://doi.org/10.1016/j.cosrev.2021.100413
Jain A, Rao ACS (2022) Praphula Kumar Jain & Ajith Abraham, “ Multi-type skin diseases classification using OP-DNN based feature extraction approach.” Multimed Tools Appl 81:6451–6476
Jovanovic M, Radovanovic S, Vukicevic M, Van Poucke S, Delibasic B (2016) Building interpretable predictive models for pediatric hospital readmission using tree-lasso logistic regression. Artif Intell Med 72:12–21. https://doi.org/10.1016/j.artmed.2016.07.003
Kasthurirathne SN, Dixon BE, Gichoya J, Huiping X, Xia Y, Mamlin B, Grannis SJ (2016) Toward better public health reporting using existing off the shelf approaches: a comparison of alternative cancer detection approaches using plaintext medical data and non-dictionary based feature selection. J Biomed Inf 60:145–152. https://doi.org/10.1016/j.jbi.2016.01.008
Khalifian S, Rosenbach M (2018) Dermatology, climate change, and the perils of attacks on expertise. J Am Acad Dermatol 79(2):397–399. https://doi.org/10.1016/j.jaad.2018.02.054
Khamparia A, Gupta D, de Albuquerque VHC, Sangiah AK, Jhaveri RH (2020) Internet of health things-driven deep learning system for detection and classification of cervical cells using transfer learning. J Supercomput 76(11):8590–8608
Khanadar A, Sharma B, Srivastava S (2016) Data mining from smart card data using data clustering school of information technology and engineering. Int J Appl Eng Res 11(1):347–52
Kimaro EG, Toribio JALML, Mor SM (2017) Climate change and cattle vector-borne diseases: use of participatory epidemiology to investigate experiences in pastoral communities in northern Tanzania. Prevent Veter Med 147:79–89. https://doi.org/10.1016/j.prevetmed.2017.08.010
Li T, Horton RM, Bader DA, Liu F, Sun Q, Kinney PL (2018) Long-term projections of temperature-related mortality risks for ischemic stroke, hemorrhagic stroke, and acute ischemic heart disease under changing climate in Beijing, China. Environ Int 112:1–9. https://doi.org/10.1016/j.envint.2017.12.006
Liang L, Gong P (2017) Climate change and human infectious diseases: a synthesis of research findings from global and spatio-temporal perspectives. Environ Int 103:99–108. https://doi.org/10.1016/j.envint.2017.03.011
Links, Dynamic Article. 2012. “Change: Progress Report, 2011.” https://doi.org/10.1039/c1pp90033a
López Figueroa F (2011) Climate change and the thinning of the ozone layer: implications for dermatology. Actas Dermo-Sifiliográficas (English Edition) 102(5):311–315. https://doi.org/10.1016/S1578-2190(11)70813-7
Pinault L, Fioletov V. 2017. “Sun Exposure, Sun protection and sunburn among Canadian Adults,” no. 82
Pinault LL, Bushnik T, Fioletov V, Peters CE, King WD, Tjepkema M. The risk of melanoma associated with ambient summer ultraviolet radiation. Statistics Canada; 2017
Purse BV, Masante D, Golding N, Pigott D, Day JC, Ibañez-Bernal S, Kolb M, Jones L (2017) How will climate change pathways and mitigation options alter incidence of vector-borne diseases? A framework for leishmaniasis in South and Meso-America. PloS one. 12(10):e0183583
Sangaiah AK, Arumugam M, Bian GB (2020) An intelligent learning approach for improving ECG signal classification and arrhythmia analysis. Artif Intell Med 103:101788
Shah SM, Shah FA, Hussain SA, Batool S (2020) Support vector machines-based heart disease diagnosis using feature subset, wrapping selection and extraction methods. Comput Electr Eng 84:106628
Wang Y, Li T (2020) Local feature selection based on artificial immune system for classification. Appl Soft Comput 87:105989. https://doi.org/10.1016/j.asoc.2019.105989
Acknowledgements
Not applicable.
Funding
Not applicable.
Author information
Authors and Affiliations
Contributions
Both coauthors contributed significantly to the research and this paper, and the first author is the main contributor.
Corresponding author
Ethics declarations
Conflict of interest
The authors declare that they have no conflict of interest.
Additional information
Publisher's Note
Springer Nature remains neutral with regard to jurisdictional claims in published maps and institutional affiliations.
This article has been retracted. Please see the retraction notice for more detail:https://doi.org/10.1007/s00500-024-09959-7
Rights and permissions
Springer Nature or its licensor (e.g. a society or other partner) holds exclusive rights to this article under a publishing agreement with the author(s) or other rightsholder(s); author self-archiving of the accepted manuscript version of this article is solely governed by the terms of such publishing agreement and applicable law.
About this article
Cite this article
Meena, K., Veni, N.N.K., Deepapriya, B.S. et al. RETRACTED ARTICLE: A novel method for prediction of skin disease through supervised classification techniques. Soft Comput 26, 10527–10533 (2022). https://doi.org/10.1007/s00500-022-07435-8
Accepted:
Published:
Issue Date:
DOI: https://doi.org/10.1007/s00500-022-07435-8