Abstract
Collaborative representation-based classification has shown promising results on cognitive vision tasks like face recognition. It solves a linear problem with \(l_1\) or \(l_2\) norm regularization to obtain a stable sparse representation. Previous studies showed that the collaboration representation assisted the output of optimum sparsity constraint, but the choice of regularization also played a crucial role in stable representation. In this paper, we proposed a novel discriminative collaborative representation-based classification method via regularization implemented by truncated total least squares algorithm. The key idea of the proposed method is combining two coefficients obtained by \(l_2\) regularization and truncated TLS-based regularization. After evaluated by extensive experiments conducted on several benchmark facial databases, the proposed method is demonstrated to outperform the naive collaborative representation-based method, as well as some other state-of-the-art methods for face recognition. The regularization by truncation effectively and dramatically enhances sparsity constraint on coding coefficients in collaborative representation and increases robustness for face recognition.




Similar content being viewed by others
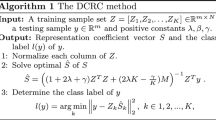
Explore related subjects
Discover the latest articles and news from researchers in related subjects, suggested using machine learning.References
Baglama J, Kane M, Lewis B, Poliakov A (2015) Efficient thresholded correlation using truncated singular value decomposition. arXiv preprint arXiv:151207246
Beck A, Teboulle M (2009) A fast iterative shrinkage-thresholding algorithm for linear inverse problems. SIAM J Imaging Sci 2(1):183–202
Brignone M, Delfino F, Procopio R, Girdinio P (2016) Regularization techniques for the high-frequency electromagnetic field coupling problem with terminated lines. J Eng Math 96(1):73–93
Chen X, Ramadge PJ (2014) Collaborative representation, sparsity or nonlinearity: What is key to dictionary based classification? In: 2014 IEEE international conference on acoustics, speech and signal processing (ICASSP). IEEE, pp 5227–5231
Cheng Y, Jin Z, Gao T, Chen H, Kasabov N (2016) An improved collaborative representation based classification with regularized least square (CRC–RLS) method for robust face recognition. Neurocomputing 215:250–259
Elgamal T, Hefeeda M (2015) Analysis of PCA algorithms in distributed environments. arXiv preprint arXiv:150305214
Fan J, Chow TW (2017) Matrix completion by least-square, low-rank, and sparse self-representations. Pattern Recogn 71:290–305
Fan J, Chow TW (2017) Sparse subspace clustering for data with missing entries and high-rank matrix completion. Neural Netw 93:36–44
Fan Z, Xu Y, Ni M, Fang X, Zhang D (2016) Individualized learning for improving kernel fisher discriminant analysis. Pattern Recognit 58:100–109
Fierro RD, Golub GH, Hansen PC, O’Leary DP (1997) Regularization by truncated total least squares. SIAM J Sci Comput 18(4):1223–1241
Gao Y, Sun Z, Wang Y, Liu X, Yan J, Zeng J (2015) A comparative study on parallel LDA algorithms in mapreduce framework. In: Bailey J, Khan L, Washio T, Dobbie G, Huang JZ, Wang R (eds) Advances in knowledge discovery and data mining. Springer, Berlin, pp 675–689
Gratton S, Titley-Peloquin D, Ilunga JT (2013) Sensitivity and conditioning of the truncated total least squares solution. SIAM J Matrix Anal Appl 34(3):1257–1276
Han ZF, Leung CS, Huang LT, So HC (2016) Sparse and truncated nuclear norm based tensor completion. Neural Process Lett 45:729–743
He K, Zhang X, Ren S, Sun J (2016) Deep residual learning for image recognition. In: Proceedings of the IEEE conference on computer vision and pattern recognition, pp 770–778
Hu Y, Zhang D, Ye J, Li X, He X (2013) Fast and accurate matrix completion via truncated nuclear norm regularization. IEEE Trans Softw Eng 35(9):2117–2130
Huang GB, Learned-Miller E (2014) Labeled faces in the wild: updates and new reporting procedures. Department of Computer Science, University of Massachusetts Amherst, Amherst, MA, USA, Tech Report, pp 14–003
Huang W, Wang X, Jin Z, Li J (2015) Penalized collaborative representation based classification for face recognition. Appl Intell 43(4):722–731
Jiang Z, Lin Z, Davis LS (2011) Learning a discriminative dictionary for sparse coding via label consistent K-SVD. In: 2011 IEEE conference on computer vision and pattern recognition (CVPR), IEEE, pp 1697–1704
Krizhevsky A, Sutskever I, Hinton GE (2012) Imagenet classification with deep convolutional neural networks. In: Tesauro G, Touretzky D, Leed T (eds) Advances in neural information processing systems. Springer, Berlin, pp 1097–1105
Landi G, Piccolomini EL, Tomba I (2016) A stopping criterion for iterative regularization methods. Appl Numer Math 106:53–68
Learned-Miller E, Huang GB, RoyChowdhury A, Li H, Hua G (2016) Labeled faces in the wild: a survey. In: Kawulok M, Celebi E, Smolka B (eds) Advances in face detection and facial image analysis. Springer, Berlin, pp 189–248
Li G, Liu ZY, Li HB, Ren P (2016) Target tracking based on biological-like vision identity via improved sparse representation and particle filtering. Cogn Comput 8:910–923
Li W, Du Q, Zhang B (2015) Combined sparse and collaborative representation for hyperspectral target detection. Pattern Recognit 48(12):3904–3916
Liao S (2004) On the homotopy analysis method for nonlinear problems. Appl Math Comput 147(2):499–513
Liu Q, Lai Z, Zhou Z, Kuang F (2016) A truncated nuclear norm regularization method based on weighted residual error for matrix completion. IEEE Trans Image Process 25(1):316–330
Liu S, Zhang X, Peng Y, Cao H (2016) Virtual images inspired consolidate collaborative representation-based classification method for face recognition. J Mod Opt 63(12):1181–1188
Liu W, Tao D, Cheng J, Tang Y (2013) Multiview Hessian discriminative sparse coding for image annotation. Comput Vis Image Underst 118(1):50–60
Long T, Jiao W, He G (2015) RPC estimation via \(l_1\)-norm-regularized least squares (L1LS). IEEE Trans Geosci Remote Sens 53(8):4554–4567
Ma H, Gou J, Wang X, Ke J, Zeng S (2017) Sparse coefficient-based k-nearest neighbor classification. IEEE Access 5:16618–16634
Na JH, Chang HJ (2016) Blockwise collaborative representation-based classification via l2-norm of query data for accurate face recognition. Electron Lett 52(13):1114–1116
Online CV (2017) Georgia tech face database. http://www.anefian.com/face_reco.htm. Accessed 3 Feb 2017
Phillips PJ, Moon H, Rizvi SA, Rauss PJ (2000) The feret evaluation methodology for face-recognition algorithms. IEEE Trans Pattern Anal Mach Intell 22(10):1090–1104
Timofte R, Van Gool L (2012) Weighted collaborative representation and classification of images. In: International conference on pattern recognition, pp 1606–1610
Wang SJ, Yang J, Sun MF, Peng XJ, Sun MM, Zhou CG (2012) Sparse tensor discriminant color space for face verification. IEEE Trans Neural Netw Learn Syst 23(6):876–888
Wang Z, Yang W, Yin J, Sun C (2013) Kernel collaborative representation with regularized least square for face recognition. Springer, Berlin
Wang Z, Liu J, Xue JH (2017) Joint sparse model-based discriminative K-SVD for hyperspectral image classification. Sig Process 133:144–155
Wong WJ, Teoh AB, Kho YH, Wong MD (2016) Kernel PCA enabled bit-string representation for minutiae-based cancellable fingerprint template. Pattern Recognit 51:197–208
Wright J, Ma Y, Mairal J, Sapiro G (2010) Sparse representation for computer vision and pattern recognition. Proc IEEE 98(6):1031–1044
Xu G, Cao Z, Hu BG, Principe JC (2017) Robust support vector machines based on the rescaled hinge loss function. Pattern Recognit 63:139–148
Xu J, Yang G, Yin Y, Man H, He H (2014) Sparse-representation-based classification with structure-preserving dimension reduction. Cogn Comput 6(3):608–621
Xu Y, Zhu Q, Chen Y, Pan JS (2013) An improvement to the nearest neighbor classifier and face recognition experiments. Int J Innov Comput Inf Control IJICIC 9(2):543–554
Xu Y, Zhu X, Li Z, Liu G, Lu Y, Liu H (2013) Using the original and ‘symmetrical face’ training samples to perform representation based two-step face recognition. Pattern Recognit 46(4):1151–1158
Xu Y, Zhang B, Zhong Z (2015) Multiple representations and sparse representation for image classification. Pattern Recognit Lett 68:9–14
Xu Y, Zhong Z, Yang J, You J, Zhang D (2016) A new discriminative sparse representation method for robust face recognition via \(l_2\) regularization. IEEE Trans Neural Netw Learn Syst 28:2233–2242
Yao Y, Guo P, Xin X, Jiang Z (2014) Image fusion by hierarchical joint sparse representation. Cogn Comput 6(3):281–292
Yin J, Wei L, Song M, Zeng W (2016) Optimized projection for collaborative representation based classification and its applications to face recognition. Pattern Recognit Lett 73:83–90
Zeng S, Yang X, Gou J (2016) Multiplication fusion of sparse and collaborative representation for robust face recognition. Multimed Tools Appl. https://doi.org/10.1007/s11042-016-4035-5
Zeng S, Gou J, Deng L (2017) An antinoise sparse representation method for robust face recognition via joint l1 and l2 regularization. Expert Syst Appl. https://doi.org/10.1016/j.eswa.2017.04.001
Zeng S, Gou J, Yang X (2017) Improving sparsity of coefficients for robust sparse and collaborative representation-based image classification. Neural Comput Appl. https://doi.org/10.1007/s00521-017-2900-4
Zhang H, Cao X, Ho JKL, Chow TWS (2017) Object-level video advertising: an optimization framework. IEEE Trans Ind Inf 13(2):520–531
Zhang H, Yang J, Xie J, Qian J, Zhang B (2017) Weighted sparse coding regularized nonconvex matrix regression for robust face recognition. Inf Sci 394:1–17
Zhang L, Yang M, Feng X (2011) Sparse representation or collaborative representation: Which helps face recognition? In: IEEE International Conference on Computer Vision, ICCV 2011. Spain, November, Barcelona, pp 471–478
Zhao J, Wang Y, Liu B (2015) Kernel collaborative representation for face recognition. In: 2014 12th international conference on signal processing (ICSP), pp 1423–1427
Zhu Q, Xu Y (2013) Multi-directional two-dimensional PCA with matching score level fusion for face recognition. Neural Comput Appl 23(1):169–174
Acknowledgements
This work was supported in part by National Natural Science Foundation of China (NSFC) (Grant Nos. 61602540, 61502208), Natural Science Foundation of Jiangsu Province of China (Grant No. BK20150522), Science and Technology Development Fund (FDCT) of Macao SAR (Grant No. 124/2014/A3), National Social Science Fund of China (Grant No. 15BTJ024), Fund Project of Humanities and Social Science Research of Chinese Ministry of Education (Grant No. 14YJAZH040), and Science and Technology Program of Huizhou City (Grant Nos. 2016X0422037, 2017C0405021).
Author information
Authors and Affiliations
Corresponding author
Ethics declarations
Conflict of interest
There are no conflicts of interest.
Rights and permissions
About this article
Cite this article
Zeng, S., Zhang, B., Lan, Y. et al. Robust collaborative representation-based classification via regularization of truncated total least squares. Neural Comput & Applic 31, 5689–5697 (2019). https://doi.org/10.1007/s00521-018-3403-7
Received:
Accepted:
Published:
Issue Date:
DOI: https://doi.org/10.1007/s00521-018-3403-7