Abstract
Now-a-days, submerged arc welding processes (SAW) are immensely being applied for joining the thick plates and surfacing application. However, the selection of optimal SAW process parameters is indeed an intricate task which aims to accomplish the desired quality of welded part at an economic way. Therefore, in the present paper, the research efforts are made on an implementation of efficient hybrid intelligent algorithms, i.e., hybrid particle swarm optimization and genetic algorithm (hybrid PSO-GA) for the optimization of SAW process parameters. The emphasis was given on different direct parameters such as voltage, wire feed rate, welding speed and nozzle to plate distance and indirect parameters such as flux condition and plate thickness, respectively. The parameters were chosen at two levels using fractional factorial design to study their effect on responses including flux consumption, metal deposition rate and heat input. Besides, the linear regression technique and analysis of variance were used for mathematical modeling of each response. Then, the direct effect and interaction effect on selected responses were investigated by 3D surface plots. At the end, the performance of hybrid PSO-GA is compared with general PSO and GA algorithms for indices including success rate, best solution, mean, computational time, standard deviation and mean absolute percentage error between. The overall results suggested that the hybrid PSO-GA is better option than other two algorithms, i.e., PSO and GA for obtaining the optimum SAW process parameters.






Similar content being viewed by others
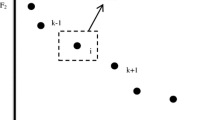
Change history
05 September 2019
In the original publication, the fourth author name was incorrectly published.
References
Vimal KEK, Vinodh S, Raja A (2015) Optimization of process parameters of SMAW process using NN-FGRA from the sustainability view point. J Intell Manuf 28(6):1459–1480. https://doi.org/10.1007/s10845-015-1061-5
Choudhary A, Kumar M, Unune DR (2018) Parametric modeling and optimization of novel water-cooled advanced submerged arc welding process. Int J Adv Manuf Technol 97(1–4):927–938. https://doi.org/10.1007/s00170-018-1944-7
Singh B, Khan ZA, Siddiquee AN, Maheshwari S (2018) Experimental study on effect of flux composition on element transfer during submerged arc welding. Sādhanā 43(26):26. https://doi.org/10.1007/s12046-018-0782-5
da Silva MM, Batista VR, Maciel TM, dos Santos MA, Brasileiro TL (2017) Optimization of submerged arc welding process parameters for overlay welding. Weld Int 32(2):122–129. https://doi.org/10.1080/09507116.2017.1347325
Kusiak A (2017) Smart manufacturing. Int J Prod Res 56(1–2):508–517. https://doi.org/10.1080/00207543.2017.1351644
Choudhary A, Kumar M, Unune DR (2017) Investigating effects of resistance wire heating on AISI 1023 weldment characteristics during ASAW. Mater Manuf Process 33(7):759–769. https://doi.org/10.1080/10426914.2017.1415441
Chaki S, Bathe RN, Ghosal S, Padmanabham G (2015) Multi-objective optimisation of pulsed Nd:YAG laser cutting process using integrated ANN–NSGAII model. J Intell Manuf 29(1):175–190. https://doi.org/10.1007/s10845-015-1100-2
Zhang F, Zhou T (2017) Process parameter optimization for laser-magnetic welding based on a sample-sorted support vector regression. J Intell Manuf. https://doi.org/10.1007/s10845-017-1378-3
Zhang Z, Chen S (2014) Real-time seam penetration identification in arc welding based on fusion of sound, voltage and spectrum signals. J Intell Manuf 28(1):207–218. https://doi.org/10.1007/s10845-014-0971-y
Zhou Q, Cao L, Zhou H, Huang X (2018) Prediction of angular distortion in the fiber laser keyhole welding process based on a variable-fidelity approximation modeling approach. J Intell Manuf 29(3):719–736. https://doi.org/10.1007/s10845-018-1391-1
Singh A, Datta S, Mahapatra SS, Singha T, Majumdar G (2011) Optimization of bead geometry of submerged arc weld using fuzzy based desirability function approach. J Intell Manuf 24(1):35–44. https://doi.org/10.1007/s10845-011-0535-3
Sarkar A, Majumder A, Pawar M, Saha SC, Rai RN (2014) Optimization of process parameters of submerged arc welding by using grey–fuzzy-based Taguchi method for AISI 1518 grade steel. Proc Inst Mech Eng Part B 228(11):1491–1500. https://doi.org/10.1177/0954405414521064
Rao RV, Rai DP (2017) Optimization of submerged arc welding process parameters using quasi-oppositional based Jaya algorithm. J Mech Sci Technol 31(5):2513–2522. https://doi.org/10.1007/s12206-017-0449-x
Pu J, Yu S, Li Y (2017) Parameter optimization of flux-aided backing-submerged arc welding by using Taguchi method. Int J Mod Phys B 31(16–19):1744046. https://doi.org/10.1142/s0217979217440465
Patnaik A, Biswas S, Mahapatra SS (2007) An evolutionary approach to parameter optimisation of submerged arc welding in the hardfacing process. Int J Manuf Res 2(4):462. https://doi.org/10.1504/ijmr.2007.015089
Moradpour M, Hashemi S, Khalili K (2015) Multi-objective optimization of welding parameters in submerged arc welding of API X65 steel plates. J Iron Steel Res Int 22(9):870–878
Lafdani MHK, Ghadiri Zahrani E, Galloway AM (2014) Optimization study of weld geometry in the tandem submerged arc welding process. Proc Inst Mech Eng Part B 229(6):1068–1077. https://doi.org/10.1177/0954405414535578
Dhas JER, Kumanan S (2011) Optimization of parameters of submerged arc weld using non conventional techniques. Appl Soft Comput 11(8):5198–5204. https://doi.org/10.1016/j.asoc.2011.05.041
Datta S, Bandyopadhyay A, Pal PK (2008) Grey-based taguchi method for optimization of bead geometry in submerged arc bead-on-plate welding. Int J Adv Manuf Technol 39(11–12):1136–1143. https://doi.org/10.1007/s00170-007-1283-6
Vedrtnam A, Singh G, Kumar A (2018) Optimizing submerged arc welding using response surface methodology, regression analysis, and genetic algorithm. Defence Technol. https://doi.org/10.1016/j.dt.2018.01.008
Raju M, Gupta MK, Bhanot N, Sharma VS (2018) A hybrid PSO–BFO evolutionary algorithm for optimization of fused deposition modelling process parameters. J Intell Manuf. https://doi.org/10.1007/s10845-018-1420-0
Anderson MJ, Whitcomb PJ (2016) DOE simplified: practical tools for effective experimentation. CRC Press, Boca Raton
Anderson MJ, Whitcomb PJ (2016) RSM simplified: optimizing processes using response surface methods for design of experiments. Productivity Press, New York
Montgomery DC (2001) Design and analysis of experiments. Wiley, New York, pp 64–65
Chang A-C, Hung J-C (2010) Combining GA and iterative searching DOA estimation for CDMA signals. Neural Comput Appl 19(7):1003–1011. https://doi.org/10.1007/s00521-010-0338-z
Chen X-y, Chen Z, Zhao Y (2018) Numerical research on virtual reality of vibration characteristics of the motor based on GA-BPNN model. Neural Comput Appl 29(5):1343–1355. https://doi.org/10.1007/s00521-017-2923-x
Hasanipanah M, Jahed Armaghani D, Bakhshandeh Amnieh H, Majid MZA, Tahir MMD (2017) Application of PSO to develop a powerful equation for prediction of flyrock due to blasting. Neural Comput Appl 28(1):1043–1050. https://doi.org/10.1007/s00521-016-2434-1
Wu Z, Wu Z, Zhang J (2017) An improved FCM algorithm with adaptive weights based on SA-PSO. Neural Comput Appl 28(10):3113–3118. https://doi.org/10.1007/s00521-016-2786-6
Singh G, Gupta MK, Mia M, Sharma VS (2018) Modeling and optimization of tool wear in MQL-assisted milling of Inconel 718 superalloy using evolutionary techniques. Int J Adv Manuf Technol. https://doi.org/10.1007/s00170-018-1911-3
Mia M, Dhar NR (2017) Prediction and optimization by using SVR, RSM and GA in hard turning of tempered AISI 1060 steel under effective cooling condition. Neural Comput Appl. https://doi.org/10.1007/s00521-017-3192-4
Joseph J, Muthukumaran S (2015) Optimization of activated TIG welding parameters for improving weld joint strength of AISI 4135 PM steel by genetic algorithm and simulated annealing. Int J Adv Manuf Technol 93(1–4):23–34. https://doi.org/10.1007/s00170-015-7599-8
Marjani A, Shirazian S, Asadollahzadeh M (2018) Topology optimization of neural networks based on a coupled genetic algorithm and particle swarm optimization techniques (c-GA–PSO-NN). Neural Comput Appl 29(11):1073–1076. https://doi.org/10.1007/s00521-016-2619-7
Ali AF, Tawhid MA (2017) A hybrid particle swarm optimization and genetic algorithm with population partitioning for large scale optimization problems. Ain Shams Eng J 8(2):191–206. https://doi.org/10.1016/j.asej.2016.07.008
Garg H (2016) A hybrid PSO-GA algorithm for constrained optimization problems. Appl Math Comput 274:292–305. https://doi.org/10.1016/j.amc.2015.11.001
Mia M, Bashir MA, Dhar NR (2016) Effects of cutting parameters and machining environments on surface roughness in hard turning using design of experiment. AIP Conf Proc 1754(1):060012. https://doi.org/10.1063/1.4958453
Mia M (2017) Multi-response optimization of end milling parameters under through-tool cryogenic cooling condition. Measurement 111:134–145. https://doi.org/10.1016/j.measurement.2017.07.033
Chandel RS (1998) The effect of process variables on the flux consumption in submerged arc welding. Mater Manuf Process 13(2):181–188. https://doi.org/10.1080/10426919808935234
Cho D, Kiran D, Na S (2015) Analysis of the flux consumption and metal transfer for tandem submerged arc welding process under iso-heat input condition. Weld J 94:396s–401s
Chandel RS, Seow HP, Cheong FL (1997) Effect of increasing deposition rate on the bead geometry of submerged arc welds. J Mater Process Technol 72(1):124–128. https://doi.org/10.1016/s0924-0136(97)00139-8
Kanjilal P, Pal TK, Majumdar SK (2007) Prediction of mechanical properties in submerged arc weld metal of C-Mn steel. Mater Manuf Process 22(1):114–127. https://doi.org/10.1080/10426910601016038
Author information
Authors and Affiliations
Corresponding author
Ethics declarations
Conflict of interest
The authors declare that there is no conflict of interest.
Additional information
Publisher's Note
Springer Nature remains neutral with regard to jurisdictional claims in published maps and institutional affiliations.
Rights and permissions
About this article
Cite this article
Choudhary, A., Kumar, M., Gupta, M.K. et al. Mathematical modeling and intelligent optimization of submerged arc welding process parameters using hybrid PSO-GA evolutionary algorithms. Neural Comput & Applic 32, 5761–5774 (2020). https://doi.org/10.1007/s00521-019-04404-5
Received:
Accepted:
Published:
Issue Date:
DOI: https://doi.org/10.1007/s00521-019-04404-5