Abstract
Our country’s market economy is composed of enterprises. However, due to their inherent credit deficiencies and high risks of management, it is very difficult for them to obtain financing support. Based on this, this article studies Error Back Propagation (BP) to establish (SMEs). Based on the relevant concepts of the supply chain management budget model, it explores the main factors influencing the financial impact of SMEs and the benefits of the supply chain budget in solving problems expenditure of SMEs, support vector machine is mainly based on solving the main credit risks of small and medium-sized enterprises, such as poor information transparency, low credit and various risk unknown factors. BP neural network is an algorithm that takes into account the components of supply chain financial financing. This article first gives a simple background and theoretical introduction to the under the current supply chain finance model, and then proposes to use SVM and BP neural network algorithms to build and the model has been trained and tested. After collecting relevant references, we will establish authoritative risk assessment rules in accordance with this article according to these standards. These experts are mainly people with many years of experience in the financial industry, and they also have a certain influence in the industry. The risk assessment established for this can be the analysis of factors such as risk indicators and government intervention in this experiment.








Similar content being viewed by others
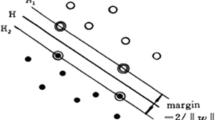
Explore related subjects
Discover the latest articles, news and stories from top researchers in related subjects.References
Zheng Z, Huang XJ (2018) Performance evaluation of small and medium-sized enterprises in the supply chain finance model. J Anqing Norm Univ (Soc Sci Ed) 037(002):78–81
Ji XL, He S (2019) Supply chain finance, commercial banks and private enterprises’ financing dilemma relief. J Henan Inst Educ (Philos Soc Sci Ed) 038(004):87–93
Shen AZ, Guo JL, Suo Q et al (2017) Modeling and analysis of supply chain finance based on multi-layer network. Appl Res Comput 034(012):3628–3631
Ma X, Lv S (2019) Financial credit risk prediction in internet finance driven by machine learning. Neural Comput Applic 31:8359–8367
Rajkumar MV, Prakasam P, Manoharan PS (2016) Investigational validation of PV based DCD-MLI using simplified SVM algorithm utilizing FPGA tied with independent sources. Circuits Syst 07(11):3831–3848
Wei J, Zhang R, Yu Z et al (2017) A BPSO-SVM algorithm based on memory renewal and enhanced mutation mechanisms for feature selection. Appl Soft Comput 58(1):176–192
Ghansah B, Benuwa B-B, Monney A (2021) A Discriminative locality-sensitive dictionary learning with kernel weighted KNN classification for video semantic concepts analysis. Int J Intell Inf Technol 17(1):68–91
He ZJ, Wang X, Li K et al (2017) Fault diagnosis of railway bearings based on FIR-EMD and improved SVM algorithm. Noise Vib Control 037(002):143–147
Li S, Shi W, Wang J, Zhou H (2021) A deep learning-based approach to constructing a domain sentiment lexicon: a case study in financial distress prediction. Inf Process Manag 58(5):102673
Feng L (2018) Study on prediction of coal roadway support scheme based on improved BP neural network algorithm. China Coal 044(005):61–64
Wang Z, Wang B, Liu C et al (2017) Improved BP neural network algorithm to wind power forecast. J Eng 2017(13):940–943
Yu H (2016) Robust analysis model of interest rate marketization from the perspective of supply chain finance. China Manag Sci 24(2):19–26
Zhou LQ, Li ZH (2016) Application of blockchain in supply chain finance. Inf Syst Eng 07(7):49–51
Quayes S, Jamal AMM (2016) Impact of demographic change on stock prices. Q Rev Econ Finance 60(May):172–179
Ill WGH, Huang GC, Liano K et al (2019) Firm and industry informational content from REIT FFO announcements. J Prop Res 36(2):1–22
Fréry F, Lecocq X, Warnier V (2017) Competing with ordinary resources. Post-Print 56(3):69–77
Tian J, Chen C (2016) Research on the credit risk evaluation index system of third-party logistics enterprises——based on the perspective of supply chain finance. Sci Manag 036(002):65–71
Liu YG (2019) Analysis and insights on the distribution characteristics of supply chain finance big data. Comput Sci 046(002):1–10
Huang H, Liu Y (2018) Research on supply chain financial risk assessment based on large samples. Value Eng 037(005):84–88
Xiang D, Zhang Y, Worthington AC (2021) Determinants of the use of fintech finance among Chinese small and medium-sized enterprises. IEEE Trans Eng Manage 68(6):1590–1604
Jiang J, Yang M, Kiang MY, Cameron AF (2021) Exploring the freemium business model for online medical consultation services in China. Inf Process Manag 58(3):102515
Teerasoponpong S, Sopadang A (2021) A simulation-optimization approach for adaptive manufacturing capacity planning in small and medium-sized enterprises. Expert Syst Appl 168:114451
Author information
Authors and Affiliations
Corresponding author
Ethics declarations
Conflict of interest
These no potential competing interests in our paper. And all authors have seen the manuscript and approved to submit to your journal. We confirm that the content of the manuscript has not been published or submitted for publication elsewhere.
Additional information
Publisher's Note
Springer Nature remains neutral with regard to jurisdictional claims in published maps and institutional affiliations.
Rights and permissions
About this article
Cite this article
Zhao, J., Li, B. Credit risk assessment of small and medium-sized enterprises in supply chain finance based on SVM and BP neural network. Neural Comput & Applic 34, 12467–12478 (2022). https://doi.org/10.1007/s00521-021-06682-4
Received:
Accepted:
Published:
Issue Date:
DOI: https://doi.org/10.1007/s00521-021-06682-4