Abstract
Human gait analysis is a well-defined technique for human identification and tracking at distance based on their walking style. It plays an important role in the video surveillance, medical, and defense applications. A number of sensors such as MEMS accelerators, gyroscopes, pressure, and position were deployed for measuring the different gait signals from the body and utilized for the different analysis of human behavior. To effectively reconcile these innovations in medical profession, system is required to identify the most important body features which have an impact on an accurate diagnosis and classification. This study proposes the novel method FOW which intended to choose the best gait features as optimization strategy based on the hybrid integration of whale and firefly algorithms. This approach is utilized for approximating the performance of different classification benchmarks in order to have an efficient medical diagnosis system. In fact, classification issue for the whole set of features is terminated, and it can be significantly pruned. Experimentation has been carried for 35 individuals in which 16 features has been recorded and analyzed. Moreover, the proposed methodology has tested with the different learning algorithms in which integrating with the extreme learning machine has produced nearly 98.5% of accuracy and also outperformed the other existing selection methodologies such as accuracy, sensitivity, and specificity on different classification platforms.








Similar content being viewed by others
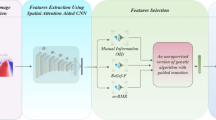
Change history
31 August 2023
This article has been retracted. Please see the Retraction Notice for more detail: https://doi.org/10.1007/s00779-023-01749-6
References
Pirker W, Katzenschlager R (2017) Gait disorders in adults and the elderly. Wien Klin Wochenschr 129:81–95 [CrossRef]
Haynes CA, Lockhart TE (2012) Evaluation of gait and slip parameters for adults with intellectual disability. J Biomech 45:2337–2341 [CrossRef]
Verghese J, Lipton R, Hall CB, Kuslansky G, Katz MJ, Buschke H (2002) Abnormality of gait as a predictor of non-Alzheimer’s dementia. N Engl J Med 347:1761–1768 [CrossRef]
Brandler TC, Wang C, Oh-Park M, Holtzer R, Verghese J (2012) Depressive symptoms and gait dysfunction in the elderly. Am J Geriatr Psychiatry 20:425–432 [CrossRef]
Mendes J Jr, José JA, Vieira MEM, Pires MB, Stevan SL Jr (2016) Sensor fusion and smart sensor in sports and biomedical applications. Sensors 16:–1569 [CrossRef]
Gouwanda D; Senanayake SMNA (2008) Emerging trends of body-mounted sensors in sports and human gait analysis. In Proceedings of the 4th Kuala Lumpur International Conference on Biomedical Engineering, Kuala Lumpur, Malaysia, 25–28 June 2008; Springer: New York, pp. 715–718
Choi SI; Lee SS; Park HC; Kim H (2018) Gait type classification using smart insole sensors. In Proceedings of the TENCON 2018–2018 IEEE Region 10 Conference, Jeju. pp. 1903–1906
Kobylarz J, Bird JJ, Faria DR, Ribeiro EP, Ekár A (2020) Thumbs up, thumbs down: non-verbal human-robot interaction through real-time EMG classification via inductive and supervised transductive transfer learning. J Ambient Intell Humaniz Comput. https://doi.org/10.1007/s12652-020-01852-z
Huang H, Kuiken TA, Lipschutz RD (2009) A strategy for identifying locomotion modes using surface electromyography. IEEE Trans Biomed Eng 56:65–73 [CrossRef]
Min SD, Kwon CK (2012) Step counts and posture monitoring system using insole type textile capacitive pressure sensor for smart gait analysis. J Korea Soc Comput Inf 17:107–114 [CrossRef]
Zhang T, Venture G (2012) Individual recognition from gait using feature value method. Cybern Inf Technol 12:86–95 [CrossRef]
Tong K, Granat MH (1999) A practical gait analysis system using gyroscopes. Med Eng Phys 21:87–94 [CrossRef]
Yun X, Bachmann ER, Moore H, Calusdian J (2007) Self-contained position tracking of human movement using small inertial/magnetic sensor modules. In: Proceedings of the 2007 IEEE International Conference on Robotics and Automation, Roma, 2526–2533
Farah JD, Baddour N, Lemaire ED (2017) Gait phase detection from thigh kinematics using machine learning techniques. In: Proceedings of the 2017 IEEE International Symposium on Medical Measurements and Applications (MeMeA), Rochester, 263–268
Wahid F, Begg RK, Hass CJ, Halgamuge S, Ackland DC (2015) Classification of Parkinson’s disease gait using spatial-temporal gait features. IEEE JBiomed HealthInform 19:1794–1802 [CrossRef]
Dolatabadi E, Mansfield A, Patterson KK, Taati B, Mihailidis A (2016) Mixture-model clustering of pathological gait patterns. IEEE J Biomed Health Inform 21:1297–1305 [CrossRef]
Parkka J, Ermes M, Korpipaa P, Mantyjarvi J, Peltola J, Korhonen I (2006) Activity classification using realistic data from wearable sensors. IEEETrans Inf Technol Biomed 10:119–128 [CrossRef] Sensors 2019, 19, 3785 13 of 13
Manap HH, Tahir NM, Yassin AIM (2011) Statistical analysis of Parkinson disease gait classification using artificial neural network. In: Proceedings of the 2011 IEEE International Symposium on Signal Processing and Information Technology (ISSPIT), Bilbao, Spain, 60–65
Taborri J, Rossi S, Palermo E, Patanè F, Cappa P (2014) A novel HMM distributed classifier for the detection of gait phases by means of a wearable inertial sensor network. Sensors 14:16212–16234 [CrossRef]
Saeys Y, Inza I, Larrañaga P (2007) A review of feature selection techniques in bioinformatics. Bioinformatics 23(19):2507–2517 [Online]. Available: http://bioinformatics.oxfordjournals.org/content/23/19/2507.abstract
Smialowski P, Frishman D, Kramer S (2010) Pitfalls of supervised feature selection. Bioinformatics 26(3):440–443 Online]. Available: http://www.ncbi.nlm.nih.gov/pmc/articles/PMC2815655/
Kwak N, Choi C-H (2002) Input feature selection for classification problems. IEEE Trans Neural Netw 13(1):143–159
Song BC, Kim MJ, Ra JB (2001) A fast multiresolution feature matching algorithm for exhaustive search in large image databases. IEEE Trans Circuits Syst Video Technol 11(5):673–678
Iguyon I, Elisseeff A (2003) An introduction to variable and feature selection. J Mach Learn Res 3:1157–1182
Altilio R, Liparulo L, Proietti A, Paoloni M, Panella M A genetic algorithm for feature selection in gait analysis. 2016 IEEE Congress Evol Comput (CEC). https://doi.org/10.1109/CEC.2016.7744374
Faezeh Tafazzoli, George Bebis, Sushil Louis, Muhammad Hussain.Improving Human Gait Recognition Using Feature Selection.Springer International Publishing Switzerland ISVC (2014) Part II. LNCS 8888(2014):830–840
Yeoh TW, Zapotecas-Martınez S, Akimoto Y, Aguirre HE, Tanaka K. (2015) Feature selection in gait classification using geometric PSO assisted by SVM. Springer International Publishing Switzerland, CAIP 2015, Part II, LNCS 9257, pp. 566–578, https://doi.org/10.1007/978-3-319-23117-449
http://eprints.soton.ac.uk/266970/Media (2007) reports of the tunnel are available: 'It's The Way You Walk' Biometrics, BBC [TV],
Mikos V, Heng CH, Tay A, Chia NSY, Koh KML, Tan DML, Au WL. (2017) Optimal window lengths, features and subsets thereof for freezing of gait classification. ICIIBMS, TRACK2: Artificial Intelligence, Robotics, and Human-Computer Interaction, Okinawa
Sanchez-Dela Cruz E, Weber R, Biswal RR, Mejıa J, Hernandez-Chan G, Gomez-Pozos H (2019) Gait biomarkers classification by combining assembled algorithms and deep learning: results of a local study. Hindawi Comp Math Methods Med 2019(3515268):14. https://doi.org/10.1155/2019/3515268
Guo H, Li B, Zhang Y, Yu Z, Li W, Qiao F, Rong X, Zhou S (2020) Gait recognition based on the feature extraction of gabor filter and linear discriminant analysis and improved local coupled extreme learning machine. Hindawi Math Probl Eng 2020(5393058):9. https://doi.org/10.1155/2020/5393058
Alotaibi M, Mahmood A Automatic real time gait recognition based on spatiotemporal templates. 2015 Long Island Systems. Appl Technol. https://doi.org/10.1109/LISAT.2015.7160196
Choi S-I, Moon J, Park H-C, Choi ST (2019) User identification from gait analysis using multi-modal sensors in smart insole. Sensors 19:3785. https://doi.org/10.3390/s19173785
Yang XS, (2009) Firefly algorithms for multimodal optimisation, Proc. 5th Symposium on Stochastic Algorithms, Foundations and Applications, (Eds. O. Watanabe and T. Zeugmann), Lecture Notes in Computer Science, 5792: 169–178
Cover T, Hart P (1967) Nearest neighbor pattern classification. IEEE Trans on Infor Theory 13(1):21–27
Huang G-B, Zhu Q-Y, Siew C-K (2006) Extreme learning machine: theory and applications. Neurocomputing 70(1):489–501
Langley P, Iba W, and Thompson K, (1992) “An analysis of bayesian classifiers,” In Proceedings of the Tenth National Conference on Artificial Intelligence, ser. AAAI’92. AAAI Press, pp. 223–228
Author information
Authors and Affiliations
Corresponding author
Additional information
Publisher’s note
Springer Nature remains neutral with regard to jurisdictional claims in published maps and institutional affiliations.
This article has been retracted. Please see the retraction notice for more detail: https://doi.org/10.1007/s00779-023-01749-6
Rights and permissions
Springer Nature or its licensor (e.g. a society or other partner) holds exclusive rights to this article under a publishing agreement with the author(s) or other rightsholder(s); author self-archiving of the accepted manuscript version of this article is solely governed by the terms of such publishing agreement and applicable law.
About this article
Cite this article
Monica, K.M., Parvathi, R. RETRACTED ARTICLE: Hybrid FOW—a novel whale optimized firefly feature selector for gait analysis. Pers Ubiquit Comput 27, 793–805 (2023). https://doi.org/10.1007/s00779-021-01525-4
Received:
Accepted:
Published:
Issue Date:
DOI: https://doi.org/10.1007/s00779-021-01525-4