Abstract
As population aging intensifies and automation technology advances, robotic massage has emerged as a significant healthcare innovation. However, participants often experience discomfort and fear when facing cold robot arms. This study proposes and validates a Mixed Reality (MR) solution integrating high-fidelity virtual massage therapists with robotic massage systems to improve participant experience. We evaluated three conditions: real robot arm, virtual robot arm, and virtual massage therapist. Results demonstrate that virtual anthropomorphic representation significantly improves participant trust, positive affect, and engagement while reducing psychological barriers. Despite challenges like increased oculomotor strain, our research confirms MR technology’s potential in enhancing psychological acceptance of robotic massage, opening new avenues for robotic massage services that may extend to other intimate human-robot interaction scenarios.
Similar content being viewed by others
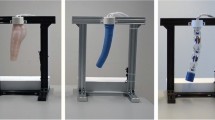
Explore related subjects
Discover the latest articles, news and stories from top researchers in related subjects.Avoid common mistakes on your manuscript.
1 Introduction
The integration of robotic technology into massage therapy has facilitated standardized, efficient, and repeatable treatments (Hu et al. 2013; Paul et al. 2021). However, despite their technical advantages, current robotic massage systems predominantly rely on exposed mechanical arms, which often evoke discomfort and psychological resistance among participants due to their rigid and impersonal nature (Syrdal et al. 2006; Chen et al. 2011; Lasota et al. 2017; Mockel et al. 2024). Research in human-robot interaction (HRI) suggests that beyond mechanical precision, participant acceptance depends on the ability of these systems to provide emotionally engaging and psychologically comfortable experiences (Bauer et al. 2010; Moyer et al. 2011). The lack of human-likeness in robotic massage interactions remains a major barrier to widespread adoption, raising concerns about trust, emotional engagement, and overall relaxation quality.
Mixed Reality (MR) has emerged as a promising approach to enhance the psychological adaptability of robotic interactions by seamlessly integrating virtual human representations with physical robotic systems. MR-based applications have demonstrated their potential in therapeutic and relaxation environments, improving emotional comfort, engagement, and stress reduction (Kizony et al. 2003; Mangal et al. 2017; Powell and Powell 2015). By creating immersive virtual environments where participants can interact with digital agents that simulate human-like behaviors, MR technology enables a more intuitive and comfortable human-robot interaction (Al-Sada et al. 2020; Vonach et al. 2017; Zhao et al. 2017; Suzuki et al. 2020). However, despite these advancements, existing robotic massage systems rarely leverage MR’s potential to integrate high-fidelity virtual massage therapists that can provide synchronized visual-haptic experiences.
This gap in research leads to a critical question: Can a high-fidelity virtual massage therapist, synchronized with a robotic massage system in an MR environment, improve participant trust, emotional engagement, and overall relaxation experience? The effectiveness of such integration remains underexplored, particularly in how virtual embodiment affects psychological comfort, motion sickness, and human-robot interaction quality. Addressing these challenges requires a comprehensive evaluation of how MR-based robotic massage impacts participant perceptions and acceptance.
To investigate this, we introduce RelaxMR, an MR-integrated robotic massage system that synchronizes a high-fidelity virtual massage therapist with a real robotic massage arm. This study systematically examines the system’s effectiveness in enhancing human-robot interaction by assessing participant trust, relaxation levels, engagement, motion sickness, and emotional well-being. Through empirical validation, we provide evidence that MR-based virtual representations significantly improve psychological comfort, reduce perceived mechanical discomfort, and foster stronger participant attachment to the system.
In summary, our key contributions are:
-
We propose and validate a novel MR-integrated robotic massage system that enhances human-robot interaction through a high-fidelity virtual massage therapist synchronized with a robotic massage arm.
-
We provide empirical evidence demonstrating that virtual human representations improve trust, relaxation, and emotional engagement, addressing key psychological barriers in robotic therapy adoption.
-
We analyze the role of virtual embodiment in robotic interaction, highlighting how MR-based systems can enhance emotional connection, reduce discomfort, and improve overall system acceptance.
2 Related work
2.1 Psychological barriers in human-robot interaction
The effectiveness of robotic systems in human interaction largely depends on participants’ psychological acceptance and comfort. Prior studies indicate that robots used in physical contact scenarios, such as caregiving and massage, can induce psychological resistance due to their mechanical nature and lack of human-likeness (Syrdal et al. 2006; Chen et al. 2011; Lasota et al. 2017). In the domain of robotic massage, these barriers can directly impact participant trust, emotional engagement, and relaxation levels (Bauer et al. 2010; Moyer et al. 2011).
participant trust is a key determinant in overcoming these psychological challenges. Research shows that human-like design elements, such as facial expressions, voice, and naturalistic movement patterns, significantly improve trust and perceived warmth in robots (Jung and Lee 2004; Broadbent et al. 2009; Holthöwer and van Doorn 2023). DeVault et al. (2014) found that embodied virtual agents can enhance interaction by providing socially engaging behaviors, which may be applicable to robotic massage scenarios. However, existing studies have primarily focused on general social robots, with limited research addressing intimate physical interactions like massage therapy.
Furthermore, recent research highlights that multi-sensory stimulation, including haptic and visual cues, can significantly affect participant perception in physical HRI. Studies indicate that mismatched sensory feedback-where visual stimuli do not align with haptic feedback-can cause discomfort and reduce trust in robotic systems (Ren and Belpaeme 2024). Current robotic massage approaches struggle to provide a seamless integration of visual and physical stimuli, potentially limiting their effectiveness in real-world applications.
2.2 Mixed reality and psychological comfort in HRI
Mixed Reality has emerged as a promising solution for enhancing participant trust, engagement, and relaxation in HRI applications. MR enables the integration of virtual human representations with robotic systems, allowing participants to perceive robots as more human-like and socially present (Malloy and Milling 2010; Yilmaz Yelvar et al. 2017; Scapin et al. 2018; Dongye et al. 2023). Research on virtual embodiment suggests that participants exhibit stronger emotional attachment and acceptance when interacting with high-fidelity virtual agents (Kim et al. 2016; Latoschik et al. 2019; Kim et al. 2020; Pimentel and Vinkers 2021).
Furthermore, MR-based interventions have demonstrated positive effects in therapeutic and relaxation settings. Studies show that immersive environments can reduce stress, increase positive emotions, and enhance overall well-being (Diniz Bernardo et al. 2021; Botella et al. 2004; Riva et al. 2007). Wagner et al. (2006) suggest that high-fidelity virtual humans can evoke relaxation responses comparable to real human interaction, supporting the idea that MR-enhanced robotic massage may provide similar benefits.
However, despite these advancements, MR has not been extensively studied in the context of robotic massage systems. Current research on MR applications primarily focuses on purely virtual interventions, without exploring how MR can complement physical robotic touch (Reis et al. 2020). Additionally, concerns such as motion sickness and visual fatigue need to be carefully addressed when integrating MR into robotic massage, as Harada et al. (2024) highlight the potential strain caused by prolonged virtual exposure.
2.3 Limitations of current robotic massage systems
Despite technological advancements, existing robotic massage systems remain limited in their ability to optimize participant experience beyond mechanical precision. Current research predominantly focuses on two aspects: (1) hardware design improvements, and (2) massage algorithm optimization, while neglecting psychological and emotional dimensions.
In hardware development, Han et al. (2011) introduced multi-fingered robotic hands that mimic human massage techniques, and Haraguchi and Kitazaki (2023) explored projected virtual enhancements to improve participant perception of robot arms. However, these approaches do not fully address the psychological discomfort associated with mechanical robotic structures.
In algorithmic optimization, Khoramshahi et al. (2020) proposed reinforcement learning-based massage motion strategies, while Xiao et al. (2023) developed force-controlled robotic massage algorithms. While these methods enhance technical precision, they fail to incorporate psychological factors, such as participant comfort, trust, and engagement.
Moreover, existing robotic massage solutions lack a seamless integration of haptic and visual feedback, which is crucial for creating an immersive and psychologically comfortable experience. Studies suggest that multimodal integration-such as combining haptic feedback with synchronized virtual representations-can significantly improve participant perception and relaxation in therapeutic applications (Slevin and McCLELLAND 1999; Brelet and Gaffary 2022).
However, despite these advancements, current robotic massage systems still face a fundamental challenge: they optimize either mechanical execution or virtual immersion but rarely achieve a seamless integration of both modalities. This fragmentation results in a suboptimal participant experience, reducing trust, emotional engagement, and overall relaxation quality. Prior research has not systematically examined how real-time synchronized haptic-visual feedback influences participant acceptance and psychological comfort in robotic massage. Addressing these challenges requires a novel approach that effectively synchronizes high-fidelity virtual representations with physical robotic interaction, ultimately improving the overall participant experience.
3 Methodology
3.1 Conceptual design
This study is dedicated to developing an innovative semi-physical, high-fidelity virtual massage therapist experience system by integrating robot arm technology with Mixed Reality technology. participants of this system wear a virtual reality headset and interact with a digitalized massage therapist in a virtual environment. The core of the system is the synchronization of the massage module on the robot arm with the actions of the virtual massage therapist, ensuring a seamless integration of visual and tactile experiences.
To achieve this, the study utilizes the Shining Physiotherapy Robot manufactured by Limber, which is designed to precisely simulate therapeutic massages, especially abdominal massages (Limber Robot xx). The choice of abdominal massage is primarily based on two factors: firstly, the abdomen is a central area for various visceral organs, and massaging this area can promote digestive system health; secondly, the abdominal area is easily observable by experiment observers and perceivable by participants themselves, which enhances the interactivity and visibility of the experiment.
The system structure diagram is shown in Fig. 1.
3.2 System components
The experiment was set up in a 2 m × 3 m space simulating a traditional massage room, equipped with a professional massage bed and a Shining Physiotherapy robot arm. This robot arm operates in a clockwise circular massage path, with adjustable force (1–30 Newtons) and temperature (20–40 \(^\circ \text {C}\)) settings to accommodate various participant needs. These parameters allow for customization based on individual preferences and therapeutic requirements. Equipped with a high-resolution camera, it enables real-time body positioning by detecting the participant’s navel through color recognition for precise abdominal massage.
The robot arm features 6 degrees of freedom with ±0.05 mm positioning accuracy, providing the precision necessary for effective massage therapy. Massage speed is typically set between 0.05 and 0.06 m/s to ensure comfort and safety. The system uses a high-performance computer (Intel Core i9-7900X, NVIDIA GeForce RTX 3080, 32GB RAM) to support the virtual environment while maintaining smooth operation of both visual elements and robotic components.
3.3 Interaction design and virtual implementation
To enhance immersion and enable precise experimental data comparisons, we employed advanced virtual reality techniques focused on gaze tracking, realistic virtual agent modeling, and full-body motion capture.
Gaze tracking and interaction detection In virtual reality, tracking participant gaze is essential for intuitive interaction, as it reveals focal points and participant intent, thereby enhancing natural engagement (Acker and Levitt 1987). Our system continuously monitors line of sight and head movements by casting a virtual ray from the head-mounted display (HMD). When this ray intersects key areas (e.g., hands, primary limbs) on the virtual massage therapist or robot arm, interaction data is recorded through a collision detection system that excludes smaller parts to optimize performance. This approach provides precise tracking of the participant’s point-of-regard, recording the duration of gaze on each area for data analysis and synchronizing with virtual animations (Steptoe and Steed 2012; Jonker et al. 2021; Clay et al. 2019).
High-fidelity virtual massage therapist construction Research shows that highly anthropomorphic virtual agents significantly increase participant engagement (Bălan et al. 2020; DeVault et al. 2014; Cassell 2001; Wagner et al. 2006. To create an expressive virtual massage therapist, we developed a light-field capture system to collect high-resolution, multi-expression facial images, which were reconstructed into 3D models and rendered in Unreal Engine 5. This produced a virtual therapist with dynamic facial responsiveness. Studies indicate that high-fidelity 3D modeling enhances interactivity and immersion, making virtual agents more appealing and credible, especially in dynamic tasks (Cho et al. 2020; McDonnell et al. 2012. For realistic animation, the model includes 600 blend shapes and 700 bones, with audio-driven lip-sync to synchronize expressions with speech, adding realism to interactions.
Full-body motion capture We used the OptiTrack system to capture the full-body motions of a human masseur, accurately mapping them onto the virtual therapist. This technique ensures lifelike movements, creating an immersive, responsive experience that enhances the virtual agent’s realism and participant engagement.
3.4 Software and system control
The system architecture integrates two key components: a proprietary interactive control software and Limber Company’s robot arm control software. The main control software manages interaction logic, signal synchronization, and language processing through the Spark large language model (iFlytek Open Source 2024), which interprets voice commands into operational instructions. Communication between the Unreal Engine 5 environment and the robotic massage system is optimized for minimal latency. This integration ensures that the virtual experience remains coordinated with the physical feedback delivered by the robot arm.
Our system maintains a consistent timing offset of 0.2 s (virtual preceding physical), which is below the perceptible threshold for most participants (Meehan et al. 2002), ensuring a seamless integration of visual and tactile feedback. This precise synchronization creates a coherent experience where participants perceive the virtual therapist’s actions as directly causing the physical sensations they experience.
4 participant study
4.1 Hypotheses
-
Hypothesis 1: participants demonstrate greater trust in virtual massage experiences than in real robot arm settings.
-
Hypothesis 2: Virtual massage therapist experiences are more relaxing and comforting than other conditions.
-
Hypothesis 3: Virtual agents significantly increase participant’s active participation.
-
Hypothesis 4: Virtual environments increase motion sickness symptoms.
-
Hypothesis 5: Virtual massage therapists enhance positive affect more effectively than other conditions.
4.2 Stimuli
Our experimental conditions evolved through three design iterations beginning with the comparative framework in Sect. 3.1. As shown in Fig. 2, the final configuration comprised: (1) RA-R (Real robot arm in physical workspace), (2) VA-V (Virtual robot arm in VR environment), and (3) VT-V (Virtual therapist in VR environment). The experiment recorded two complementary perspectives: the participant’s viewpoint through the VR headset (participant POV) and the laboratory environment view (Real Scene) filmed by a camera.
The 4-minute session duration was strategically adopted from therapeutic massage protocols (Poppendieck et al. 2016; Eriksson Crommert et al. 2015) to balance efficacy and experimental control. Notably, a fourth prototype condition where participants received massages in a virtual environment that lacked both a virtual robot arm and a virtual massage therapist (No Agent in Virtual environment, NA-V) was eliminated when preliminary tests revealed 60% of participants (3/5) experienced disorientation and anxiety—phenomena aligning with Kilteni et al. (2013) sensory reference frame theory.
This iterative refinement process yielded the validated three-condition architecture, achieving optimal balance between methodological rigor and participant safety.
4.3 Participants
The study included 27 participants, comprised of 14 females and 13 males. The average age was 27.78 years, with a standard deviation of 5.37, ranging from 22 to 41 years. Participants were queried regarding their prior experiences with virtual reality (VR) and robot arm massages. Of these, 22 reported having prior VR experience, while 5 had no previous VR exposure. Concerning experiences with robot arm massages, only 2 participants had prior exposure, whereas the remaining 25 had no such experience.
4.4 Measurements
The study employed both objective and subjective measurements to assess participant engagement, VR sickness discomfort, emotional responses, trust, comfort, and acceptance of the technology.
4.4.1 Objective measurements
Gaze duration To evaluate participant engagement, a virtual ray emitted from the center of the head-mounted display tracked the participant’s visual focus and head orientation in real-time. This ray intersected with the mesh model of the virtual agent, allowing for precise measurement of the duration the participant gazed at the virtual agent during the massage session.
4.4.2 Subjective measurements
Simulator sickness questionnaire (SSQ) Developed by Kennedy et al., the SSQ is a 16-item questionnaire rated on a four-point scale, where 0 represents “none at all” and 3 indicates “severe.” The SSQ assesses four dimensions of simulator sickness: nausea, oculomotor disturbances, disorientation, and overall severity (Kennedy et al. 1993).
Positive and negative affect schedule (PANAS) The PANAS, designed by Watson et al., consists of 20 items rated on a five-point scale, where 1 represents “absolutely not” and 5 represents “completely yes.” It evaluates participants’ positive and negative emotional states (Watson et al. 1988).
Participant experience questionnaire - short version (UEQ-S) The UEQ-S, as designed by Schrepp et al., measures participant experience across conditions. It uses a 7-point Likert scale, where 1 represents “strongly disagree” and 7 represents “strongly agree.” This scale evaluates Pragmatic Quality (usability and efficiency), Hedonic Quality (stimulation and originality), and provides an Overall Quality score as an average of these dimensions (Schrepp et al. 2017).
Unified theory of acceptance and use of technology (UTAUT): We adopted a modified version of the UTAUT (Block et al. 2023) Onishi et al. (2024) as our theoretical framework, widely used in human-robot and Mixed Reality interactions Heerink et al. (2006) Fitter et al. (2020) Shao and Lee (2020). This model evaluates acceptance by examining performance expectancy, effort expectancy, social influence, facilitating conditions, reciprocity, and attachment. Each item was rated on a 7-point Likert scale. The complete questionnaire is provided in Table 1.
4.4.3 Semi-structured interviews
To gain a well-rounded understanding of participant experiences, semi-structured interviews were conducted, focusing on key features of the virtual massage system. Participants shared their impressions of the virtual massage therapist’s interactivity and realism, the immersive environment, and natural interaction methods like voice control. The interviews also addressed synchronization between the virtual massage therapist and the robot arm, with participants providing suggestions for improvement. Finally, participants compared their preferences across three interaction modes-virtual massage therapist, virtual robot arm, and real robot arm.
4.5 Experiment procedure
Prior to initiating the experiment, the research team provided the 27 participants with a comprehensive explanation of the study’s aims and secured informed consent. The research was structured using a fully balanced Latin square design, and each participant was obliged to complete an informed consent document along with a form to collect basic demographic information at the outset of each experimental session. An initial fitting of the VR headsets and a briefing on the operation of the robot arm for massage were conducted. Subsequently, in a prearranged sequence, participants donned the VR headsets and positioned themselves on the massage chairs in anticipation of the massage. To facilitate an unimpeded massage experience, it was required for participants to expose their abdominal area or ensure that their attire was conducive to the process.
Three experimental conditions were established: 1) RA-R; 2) VA-V; 3) VT-V. The sequence of these conditions was determined by a Latin square design to maintain balance. After each massage session, participants removed the VR headset (if applicable) and completed questionnaires to gather data. Following the experiment, researchers conducted interviews to delve into the participants’ experiences (see Fig. 3). A professional robot arm operator was present during the experiments to ensure safe operation of the equipment.
5 Results
5.1 Results of gaze duration
As shown in Fig. 4, the average gaze duration for the VA-V group is approximately 46.50 s (SD : 47.01 seconds), while the average gaze duration for the VT-V group is 128.74 s (SD : 71.89 seconds). Normality tests indicate that the data for both groups do not follow a normal distribution (Shapiro-Wilk test p-value: 0.002 for the VA-V group and 0.019 for the VT-V group). Therefore, a non-parametric Mann–Whitney U test was used to evaluate the differences between the two groups. The results show a statistically significant difference in gaze duration between the two groups (\(p=0.00004\)), with the VT-V group having a significantly longer gaze duration than the VA-V group. This prolonged gaze duration in the VT-V group suggests that participants exhibited more sustained attention during interactions with the virtual massage therapist, likely due to the high fidelity of the virtual model enhancing engagement and immersion. This finding aligns with the hypothesis that high-fidelity virtual agents can extend participant focus, an important factor in immersive environments.
5.2 Results of SSQ
We conducted a comparative analysis of the SSQ responses from participants equipped with VR headsets. A Wilcoxon signed-rank test was used to analyze the results. No significant difference was observed before and after the group VA-V experiment in nausea (\(Z = -0.54\), \(p=0.589\)), oculomotor (\(Z = -1.81\), \(p=0.070\)), disorientation (\(Z = -1.01,\)\(p=0.314\)), or total score (\(Z = -1.49\), \(p=0.136\)). Similarly, no significant differences were observed before and after the group VT-V experiment in nausea (\(Z = -0.31,\)\(p=0.755\)), disorientation (\(Z = -0.72\), \(p=0.471\)), and the total score (\(Z = -1.89\), \(p=0.059\)). However, a significant difference was observed before and after the group VT-V experiment in oculomotor (\(Z = -2.924\), \(p = 0.003\)), indicating that participants in the VT-V group experienced increased oculomotor strain after the experiment. This increase in oculomotor strain suggests that high-fidelity virtual massage therapist interactions may impose a slight visual burden, highlighting the importance of optimized visual synchronization to reduce physical discomfort in complex virtual environments.
5.3 Results of PANAS
We conducted normality tests on the “positive affect” and “negative affect” scores of the three experimental groups using the Shapiro-Wilk test. Our analysis revealed that the “positive affect” scores for the RA-R group, VA-V group, and VT-V group closely approached a normal distribution both before and after the experiment, with p-values ranging from 0.161 to 0.694. This supports the application of parametric statistical methods. In contrast, the post-experiment “negative affect” scores for the VT-V group showed a significant deviation from normality (\(W = 0.870\), \(p = 0.003\)), and similar significant non-normalities were observed in the VA-V and RA-R groups, indicating a need for non-parametric analysis methods.
Paired sample t-tests were conducted to assess the changes in “positive affect” scores before and after the experiment among the three experimental groups. The score changes in the RA-R and VA-V groups did not achieve statistical significance with p-values of 0.276 and 0.125, respectively. Conversely, the score change in the VT-V group was statistically significant (\(p = 0.029\)), highlighting a meaningful effect of the virtual massage therapist interaction on positive affect. According to the results of the Wilcoxon signed-rank tests, no statistically significant differences were found in the “negative affect” scores before and after the experiment for any of the groups.
The increase in “positive affect” scores for the VT-V group suggests that interactions with the virtual massage therapist positively impacted participant mood and emotional engagement, supporting the potential for anthropomorphic virtual agents to enhance emotional well-being, a valuable asset for therapeutic and supportive applications (see Fig. 5).
5.4 Results of UEQ-S
As illustrated in Fig. 6, participant experience under different conditions (RA-R, VA-V, VT-V) was evaluated using the UEQ-S scale, covering three dimensions: Pragmatic Quality, Hedonic Quality, and Overall Score. The results indicate notable differences across conditions in each dimension. For Pragmatic Quality, RA-R and VA-V had mean scores of 1.009 and 1.074, both approaching the “Good” standard, while VT-V scored 1.537, reaching the “Excellent” level. For Hedonic Quality, RA-R scored 0.852, while VA-V and VT-V achieved scores of 1.009 and 1.565, reaching the “Good” and “Excellent” levels, respectively. The Overall Score revealed values of 0.93, 1.04, and 1.55 for RA-R, VA-V, and VT-V, respectively, all above the positive benchmark of 0.8, with VT-V achieving the “Excellent” level.
Statistical analysis further confirmed these differences. The Shapiro–Wilk test indicated that scores for all dimensions did not follow a normal distribution, so non-parametric tests were used. The Kruskal–Wallis test showed that differences across groups in Pragmatic (p = 0.146), Hedonic (p = 0.082), and Overall scores (p = 0.053) were not statistically significant, though the latter two were near significance. Pairwise Mann–Whitney U tests revealed significant differences between RA-R and VT-V in Hedonic Quality (p = 0.036) and Overall Score (p = 0.025), indicating that VT-V had significantly better participant experience than RA-R in these dimensions.
5.5 Results of UTAUT
We first used Cronbach’s \(\alpha \) to assess the internal consistency of the UTAUT dimensions. The results show that the Cronbach’s \(\alpha \) values for Attachment (\(\alpha =0.88\)), Attitude Toward Using Technology (\(\alpha =0.88\)), Cultural Context (\(\alpha =0.92\)), Effort Expectancy (\(\alpha =0.90\)), Social Influence (\(\alpha =0.86\)), Performance Expectancy (\(\alpha =0.86\)), and Reciprocity (\(\alpha =0.89\)) are all above 0.8, indicating good internal consistency for the questionnaire.
Descriptive statistical analysis revealed the means, standard deviations, and standard errors of each UTAUT dimension among the three experimental groups (RA-R group, VA-A group, VT-V group). The results show that the VT-V group scored the highest on most dimensions, including Attachment (\(M=5.65\), \(SD=1.05\), \(SE=0.20),\) Social Influence (\(M=5.35\), \(SD=1.14\), \(SE=0.22),\) Performance Expectancy (\(M=5.47\), \(SD=1.22,\)\(SE=0.23\)), and Reciprocity (\(M=5.07\), \(SD=1.30\), \(SE=0.25\)).
We used ANOVA to test for significant differences in UTAUT scores among the three groups. The results show significant differences among the three groups in the dimensions of Attachment (\(F=6.945\), \(p=0.002\)), Attitude Toward Using Technology (\(F=4.866\), \(p=0.010\)), Social Influence (\(F=5.887\), \(p=0.004\)), Performance ExpeWe first used Cronbach’s \(\alpha \) to assess the internal consistency of the UTAUT dimensions. The results show that the Cronbach’s \(\alpha \) values for Attachment (\(\alpha =0.88\)), Attitude Toward Using Technology (\(\alpha =0.88\)), Cultural Context (\(\alpha =0.92),\) Effort Expectancy (\(\alpha =0.90),\) Social Influence (\(\alpha =0.86\)), Performance Expectancy (\(\alpha =0.86\)), and Reciprocity (\(\alpha =0.89\)) are all above 0.8, indicating good internal consistency for the questionnaire.
Descriptive statistical analysis revealed the means, standard deviations, and standard errors of each UTAUT dimension among the three experimental groups (RA-R group, VA-V group, VT-V group). The results show that the VT-V group scored the highest on most dimensions, including Attachment (\(M=5.65\), \(SD=1.05\), \(SE=0.20\)), Social Influence (\(M=5.35\), \(SD=1.14\), \(SE=0.22\)), Performance Expectancy (\(M=5.47\), \(SD=1.22\), \(SE=0.23\)), and Reciprocity (\(M=5.07\), \(SD=1.30\), \(SE=0.25\)) (see Fig. 7).
We used ANOVA to test for significant differences in UTAUT scores among the three groups. The results show significant differences among the three groups in the dimensions of Attachment (\(F=6.945\), \(p=0.002\)), Attitude Toward Using Technology (\(F=4.866\), \(p=0.010\)), Social Influence (\(F=5.887\), \(p=0.004\)), Performance Expectancy (\(F=4.201\), \(p=0.019\)), and Reciprocity (\(F=7.297,\)\(p=0.001\)).
The Tukey HSD test was used in the post-hoc analysis to assess mean differences between groups. In the Attachment dimension, the mean difference between RA-R and VT-V was 1.27 (\(p=0.001\)), and the mean difference between VA-V and VT-V was 0.84 (\(p=0.047\)), both showing significant differences. The VT-V group scored significantly higher on this dimension than the other two groups. In the Attitude Toward Using Technology dimension, the mean difference between RA-R and VT-V was 1.07 (\(p=0.008\)), showing a significant difference. The VT-V group also scored higher on this dimension. In the Social Influence dimension, the mean difference between RA-R and VT-V was 1.26 (\(p=0.003\)), showing a significant difference. In the Performance Expectancy dimension, the mean difference between RA-R and VT-V was 1.00 (\(p=0.018\)), also showing a significant difference. In the Reciprocity dimension, the mean difference between RA-R and VT-V was 1.39 (\(p=0.001\)), and the mean difference between VA-V and VT-V was 0.93 (\(p=0.038\)), both showing significant differences.
To assess the practical significance of these findings, we calculated the effect size (Cohen’s d). The results show moderate to large differences between the virtual human massage (VT-V group) and other groups in several dimensions, particularly in Attachment (d = \(-\)1.05 with RA-R; d = \(-\)0.67 with VA-V), Attitude Toward Using Technology (d = \(-\)0.88 with RA-R; d = \(-\)0.51 with VA-V), Social Influence (d = \(-\)0.95 with RA-R; d = \(-\)0.59 with VA-V), Performance Expectancy (d = \(-\)0.78 with RA-R; d = \(-\)0.57 with VA-V), and Reciprocity (d = \(-\)0.98 with RA-R; d = \(-\)0.73 with VA-V).
These findings indicate that the VT-V group exhibited stronger attachment, social influence, and acceptance of the virtual massage therapist. This supports the hypothesis that high-fidelity virtual agents foster participant engagement and acceptance, offering valuable insights for designing interactive virtual experiences.
5.6 Validation of hypotheses
Based on our analyses in previous sections, we can validate our five hypotheses as follows:
Hypothesis 1
Participants demonstrate greater trust in virtual massage experiences than in real robot arm settings.
Validation: This hypothesis is supported by the UTAUT Attachment dimension data (Sect. 5.5), which includes trust-related items. The VT-V group scored significantly higher than the RA-R group (mean difference = 1.27, \(p = 0.001\)), indicating substantially greater trust in the virtual massage therapist condition. This finding aligns with prior research suggesting that virtual environments can mitigate perceived risks associated with direct robotic interaction Meehan et al. (2002) Riva (2003).
Hypothesis 2
Virtual massage therapist experiences are more relaxing and comforting than other conditions.
Validation: This hypothesis is supported by both UEQ-S and UTAUT analyses. In UEQ-S data (Sect. 5.4), the VT-V group scored significantly higher than the RA-R group in Hedonic Quality (\(p = 0.036\)) and Overall Score (\(p = 0.025\)), indicating a more enjoyable and satisfactory experience. UTAUT results further confirmed this with higher scores in Performance Expectancy (\(MD = 1.00\), \(p = 0.018\)) for the VT-V group, suggesting greater perceived effectiveness and comfort.
Hypothesis 3
Virtual agents significantly increase participant’s active participation.
Validation: Strong support for this hypothesis comes from both behavioral and self-report measures. Gaze duration analysis (Sect. 5.1) shows significantly longer attention in the VT-V group (128.74 s) compared to the VA-V group (46.50 s, \(p = 0.00004\)). This is complemented by higher UTAUT scores for the VT-V group in Social Influence and Attachment dimensions, indicating increased engagement and interaction quality.
Hypothesis 4
Virtual environments increase motion sickness symptoms.
Validation: This hypothesis is partially supported. SSQ results (Sect. 5.2) showed a significant increase in oculomotor symptoms (\(Z = -2.924\), \(p = 0.003\)) only in the VT-V condition, with no significant changes in the VA-V or total SSQ scores. This suggests that complex virtual human interactions, rather than virtual environments alone, may contribute to some visual strain.
Hypothesis 5
Virtual massage therapists enhance positive affect more effectively than other conditions.
Validation: PANAS data (Sect. 5.3) strongly supports this hypothesis, with a significant increase in positive affect scores for the VT-V group (\(p = 0.029\)), while no significant changes were observed in the RA-R and VA-V groups. This indicates the virtual massage therapist’s effectiveness in enhancing emotional well-being.
These validated hypotheses provide a foundation for discussing the broader implications of our findings in the following section.
6 Discussion
In this study, we investigated the impact of integrating a high-fidelity virtual massage therapist into a Mixed Reality robotic massage system. Our hypotheses focused on trust, relaxation, engagement, motion sickness, and emotional impact. Below, we discuss each hypothesis in relation to our findings and their implications.
Trust in virtual massage experiences. Our results support Hypothesis 1, indicating that participants exhibit greater trust in virtual massage experiences compared to direct robotic interactions. The significantly higher Attachment scores in the UTAUT model (M = 5.65, p = 0.001) for the VT-V condition suggest that anthropomorphic virtual representations can effectively bridge the psychological gap between participants and robotic massage systems. This aligns with previous research indicating that virtual agents can foster a sense of familiarity and reduce the perceived mechanical nature of robotic interactions. Prior research suggests that systems designed with human-like characteristics are more likely to establish trust and engagement, as demonstrated by DeVault et al. (2014), who found that virtual agents can create a more natural and reassuring interaction. Qualitative feedback further supports this finding, as multiple participants mentioned feeling more at ease with the virtual massage therapist compared to interacting with a robot arm.
Relaxation and comfort enhancement. Hypothesis 2 proposed that virtual massage therapists would provide a more relaxing and comforting experience. Our PANAS results showed a significant increase in positive affect (p = 0.029) for the VT-V group, while other conditions did not yield statistically significant changes. Additionally, UEQ-S scores for Hedonic Quality were highest in the VT-V condition, reaching the Excellent category. These findings suggest that a visually present virtual therapist contributes to a greater sense of relaxation and psychological comfort, potentially by reducing uncertainty and increasing immersion. Wagner et al. (2006) suggest that high-fidelity virtual agents enhance participant relaxation by creating a more immersive and emotionally engaging experience, aligning with our findings. Participant interviews revealed that many participants found the presence of a virtual therapist reassuring, and some reported a stronger psychological connection compared to experiencing a robot arm massage alone.
Increased participant engagement through virtual agents. Hypothesis 3, which predicted increased participant engagement in the presence of a virtual massage therapist, was strongly supported by the gaze duration data. The VT-V condition resulted in significantly longer gaze duration (M=128.74s, p=0.00004) compared to the VA-V condition, suggesting that participants were more engaged when interacting with the high-fidelity virtual therapist. This aligns with prior studies on avatar presence and engagement, indicating that participants form stronger connections with virtual entities that exhibit human-like behaviors. Additionally, the high scores in Social Influence and Reciprocity from the UTAUT analysis reinforce the idea that participants perceive the virtual therapist as an interactive and socially engaging entity. Reis et al. (2020) highlight that enhanced human-robot interaction designs significantly improve participant engagement and overall satisfaction, further supporting our findings. Notably, qualitative feedback indicated that some participants actively responded to the virtual therapist’s presence, treating it as a social entity rather than just a visual overlay, demonstrating an elevated level of engagement.
Motion sickness and visual strain considerations. Hypothesis 4 examined whether virtual environments would contribute to motion sickness. While no significant differences were found in nausea and disorientation symptoms across conditions, the VT-V condition resulted in significantly higher oculomotor strain (p = 0.003). This suggests that prolonged exposure to high-fidelity virtual agents may introduce additional visual load. One possible explanation is that participants maintain prolonged gaze fixation on the virtual therapist, which may lead to eye fatigue. Future studies should investigate techniques to mitigate visual strain, such as optimized rendering techniques, dynamic focus adjustments, and gaze-contingent interaction designs to alleviate cognitive load. Additionally, Harada et al. (2024) propose that the use of fixed VR headset configurations in supine positions can improve visual stability and reduce strain. Some participants also reported mild visual discomfort, particularly when the virtual therapist’s movement did not perfectly synchronize with their expectations, suggesting that motion smoothness and synchronization remain important factors in participant comfort.
Additionally, interview feedback revealed that a small subset of participants mentioned discrepancies between the virtual and physical robot arms in terms of size, structure, or visual style, causing some initial perceptual dissonance. Although this represented a minority experience, with most participants indicating smooth overall experiences, it merits consideration. Research suggests that in multimodal interaction environments, individuals can adapt to certain degrees of visual inconsistency, particularly when haptic feedback provides a coherent experience (Kilteni et al. 2012). Wijntjes et al. further demonstrated that tactile input may play a more significant role in multisensory integration processes than visual consistency (Wijntjes et al. 2009). Therefore, while visual discrepancies may not significantly impact experience quality for most participants, future systems should maintain high-quality tactile feedback while gradually improving visual presentation consistency, especially for individuals more sensitive to visual details.
Emotional impact and psychological well-being. Hypothesis 5 predicted that virtual massage therapists would enhance positive affect more effectively than other conditions. The PANAS results confirmed this hypothesis, with the VT-V condition showing a significant increase in positive affect, while the RA-R and VA-V conditions did not. These findings suggest that virtual human representation plays a crucial role in fostering emotional well-being in MR-based therapeutic applications. This effect may be linked to the perceived social presence of the virtual therapist, aligning with prior research on avatar-mediated interactions. Interviews provided further validation, as participants noted that the virtual therapist’s human-like gestures and expressions made the experience feel more natural, fostering a greater sense of presence and emotional connection.
7 Future directions and limitations
Although this study demonstrates the potential of integrating Mixed Reality into robotic massage systems, several limitations warrant further investigation. First, the study focused on abdominal massage, limiting the generalizability of the findings to other types of robotic-assisted massage. Future research should extend the system to different body regions, explore the effectiveness of first-person versus third-person perspectives, and evaluate fixed VR headset applications in supine positions. Second, occasional discrepancies existed between the virtual therapist’s visual representation and the physical feedback delivered by the robotic massage arm, including differences in size and appearance between virtual and actual robot arms. Future improvements should refine real-time synchronization between visual and haptic stimuli and investigate how physical properties of the contact area influence participant experience.
Third, the current system resulted in increased oculomotor strain, suggesting that alternative display mechanisms and optimized tracking techniques should be explored. Additionally, improving system response time and visual fidelity of the virtual therapist’s movements and expressions remains important.
Finally, this study primarily relied on subjective measures. Future research should incorporate physiological measurements such as heart rate variability, explore personalization mechanisms based on factors including gender, attire, and personality, and analyze the influence of demographic factors on participant experience.
Despite these limitations, this study provides empirical evidence supporting the benefits of MR-integrated robotic massage systems in enhancing emotional engagement, participant trust, and psychological well-being. Addressing these challenges will further expand the system’s applications in treatment settings.
8 Conclusion
This study demonstrates the substantial potential of integrating Mixed Reality technology within robotic massage systems. Our findings reveal that virtual anthropomorphic representation transforms participant perceptions of robotic systems, creating a coherent therapeutic experience and addressing psychological barriers that have limited robotic therapy adoption. The results suggest that visually embodied virtual agents play a crucial role in mitigating psychological discomfort associated with robotic massage, improving participant trust and system acceptance.
While the system showed significant advantages in emotional engagement and psychological comfort, some technical challenges remain-particularly in addressing visual fatigue and ensuring seamless virtual-physical congruence. However, these limitations highlight future research opportunities rather than fundamental obstacles. Further refinements in real-time synchronization between visual and haptic stimuli, adaptive interaction techniques, and alternative display mechanisms may enhance the system’s effectiveness and participant experience.
In conclusion, the RelaxMR system provides empirical evidence supporting the application of MR technology in robotic massage. These findings reinforce the importance of integrating virtual agents in robotic interaction, making such systems more viable for broader adoption in therapeutic settings where human-robot interaction quality is essential. Moreover, the principles demonstrated in this study may extend to other intimate human-robot interaction scenarios where psychological comfort and emotional connection directly impact intervention efficacy.
Data availibility
No datasets were generated or analysed during the current study.
References
Acker S, Levitt S (1987) Designing videoconference facilities for improved eye contact. J Broadcast Electr Media 31:181
Al-Sada M, Jiang K, Ranade S, Kalkattawi M, Nakajima T (2020) Hapticsnakes: multi-haptic feedback wearable robots for immersive virtual reality. Virtual reality 24, 191–209
Bălan O, Cristea S, Moise G, Petrescu L, IvaŞcu S, Moldoveanu A, Moldoveanu F, Leordeanu M (2020) Ether–an assistive virtual agent for acrophobia therapy in virtual reality. In: HCI International 2020–Late Breaking Papers: virtual and Augmented Reality: 22nd HCI International Conference, HCII 2020, Copenhagen, Denmark, July 19–24, 2020, Proceedings 22. Springer, pp. 12–25
Bauer BA, Cutshall SM, Wentworth LJ, Engen D, Messner PK, Wood CM, Brekke KM, Kelly RF, Sundt TM III (2010) Effect of massage therapy on pain, anxiety, and tension after cardiac surgery: a randomized study. Compl Ther Clin Pract 16(2):70–75
Block AE, Seifi H, Hilliges O, Gassert R, Kuchenbecker KJ (2023) In the arms of a robot: designing autonomous hugging robots with intra-hug gestures. ACM Trans Human-Robot Interact 12(2):1–49
Botella C, Martín HV, García-Palacios A, Baños RM, Perpiñá C, Alcañiz M (2004) Clinically significant virtual environments for the treatment of panic disorder and agoraphobia. Cyberpsychol Behav 7(5):527–535
Brelet L, Gaffary Y (2022) Stress reduction interventions: a scoping review to explore progress toward use of haptic feedback in virtual reality. Front Virt Real 3:900970
Broadbent E, Stafford R, MacDonald B (2009) Acceptance of healthcare robots for the older population: Review and future directions. Int J Soc Robot 1:319–330
Cassell J (2001) Embodied conversational agents: representation and intelligence in user interfaces. AI Mag 22(4):67–67
Chen TL, King C-H, Thomaz AL, Kemp CC (2011) Touched by a robot: An investigation of subjective responses to robot-initiated touch. In: Proceedings of the 6th international conference on human-robot interaction, pp. 457–464
Cho S, Kim S-w, Lee J, Ahn J, Han J (2020) Effects of volumetric capture avatars on social presence in immersive virtual environments. In: 2020 IEEE Conference on virtual reality and 3D user interfaces (VR). IEEE, pp. 26–34
Clay V, König P, König S (2019) Eye tracking in virtual reality. J Eye Move Res 12(1):1–18
DeVault D, Artstein R, Benn G, Dey T, Fast E, Gainer A, Georgila K, Gratch J, Hartholt A, Lhommet M, (2014) et al.: Simsensei kiosk: A virtual human interviewer for healthcare decision support. In: Proceedings of the 2014 international conference on autonomous agents and multi-agent systems, pp. 1061–1068
Diniz Bernardo P, Bains A, Westwood S, Mograbi DC (2021) Mood induction using virtual reality: a systematic review of recent findings. J Technol Behav Sci 6:3–24
Dongye X, Weng D, Jiang H, Feng L (2023) A modular haptic agent system with encountered-type active interaction. Electronics 12(9):2069
Eriksson Crommert M, Lacourpaille L, Heales L, Tucker K, Hug F (2015) Massage induces an immediate, albeit short-term, reduction in muscle stiffness. Scandinavian J Med Sci Sport 25(5):490–496
Fitter NT, Mohan M, Kuchenbecker KJ, Johnson MJ (2020) Exercising with baxter: preliminary support for assistive social-physical human-robot interaction. J Neuroeng Rehabil 17:1–22
Han L, Tian MQ, Chen JZ (2011) Development of a multi-fingered massaging robot hand. Adv Mater Res 279:429–433
Harada N, Kitazaki M, Tasaki R (2024) Robotic VR massage system: Physical care robot with out-of-body experience in virtual karesansui. In: 2024 IEEE Conference on Virtual Reality and 3D User Interfaces Abstracts and Workshops (VRW). IEEE, pp. 1039–1040
Haraguchi G, Kitazaki M (2023) Cast shadow and color change improve social impression of robotic massage with virtual out-of-body experience. In: Proceedings of the Augmented Humans International Conference 2023, pp. 301–303
Heerink M, Kröse B, Wielinga BJ, Evers V et al (2006) Studying the acceptance of a robotic agent by elderly users. Int J Assist Robot Mechatr 7(3):33–43
Holthöwer J, Doorn J (2023) Robots do not judge: service robots can alleviate embarrassment in service encounters. J Acad Mark Sci 51(4):767–784
Hu L, Wang Y, Zhang J, Zhang J, Cui Y, Ma L, Jiang J, Fang L, Zhang B (2013) A massage robot based on chinese massage therapy. Ind Robot: An Int J 40(2):158–172
iFlytek Open Source: iFlytek Spark API. Accessed: 2024-04-29. https://xinghuo.xfyun.cn/sparkapi
Jonker T, et al.: Towards gaze-based prediction of the intent to interact in virtual reality. In: Proceedings of the ETRA ’21 Conference (2021)
Jung Y, Lee KM (2004) Effects of physical embodiment on social presence of social robots. Proceed Presence 2004:80–87
Kennedy RS, Lane NE, Berbaum KS, Lilienthal MG (1993) Simulator sickness questionnaire: An enhanced method for quantifying simulator sickness. Int J Aviat Psychol 3(3):203–220
Khoramshahi M, Henriks G, Naef A, Salehian SSM, Kim J, Billard A (2020) Arm-hand motion-force coordination for physical interactions with non-flat surfaces using dynamical systems: toward compliant robotic massage. In: 2020 IEEE International conference on robotics and automation (ICRA). IEEE, pp. 4724–4730
Kilteni K, Groten R, Slater M (2012) The sense of embodiment in virtual reality. Presence: Teleoperat Virt Environ 21(4):373–387
Kilteni K, Bergstrom I, Slater M (2013) Drumming in immersive virtual reality: the body shapes the way we play. IEEE Trans Visual Comput Graphics 19(4):597–605
Kim K, Melo CM, Norouzi N, Bruder G, Welch GF (2020) Reducing task load with an embodied intelligent virtual assistant for improved performance in collaborative decision making. In: 2020 IEEE conference on virtual reality and 3d user interfaces (VR). IEEE, pp. 529–538
Kim K, Schubert R, Welch G (2016) Exploring the impact of environmental effects on social presence with a virtual human. In: Intelligent Virtual Agents: 16th International Conference, IVA 2016, Los Angeles, CA, USA, September 20–23, 2016, Proceedings 16. Springer, pp. 470–474
Kizony R, Katz N, Weiss PL (2003) Adapting an immersive virtual reality system for rehabilitation. J Vis Comput Animat 14(5):261–268
Lasota PA, Fong T, Shah JA (2017) et al.: A survey of methods for safe human-robot interaction. Foundat Trends® Robot, 5(4):261–349
Latoschik ME, Kern F, Stauffert J-P, Bartl A, Botsch M, Lugrin J-L (2019) Not alone here?! scalability and user experience of embodied ambient crowds in distributed social virtual reality. IEEE Trans Visual Comput Graphics 25(5):2134–2144
Limber Robot. http://www.limberrobot.com.cn/. Accessed: 2024-04-29
Malloy KM, Milling LS (2010) The effectiveness of virtual reality distraction for pain reduction: a systematic review. Clin Psychol Rev 30(8):1011–1018
Mangal NK, Pal S, Khosla A (2017) Frozen shoulder rehabilitation using microsoft kinect. In: 2017 International Conference on Innovations in Green Energy and Healthcare Technologies (IGEHT). IEEE, pp. 1–6
McDonnell R, Breidt M, Bülthoff HH (2012) Render me real? Investigating the effect of render style on the perception of animated virtual humans. ACM Trans Graphics (TOG) 31(4):91–19111
Meehan M, Insko B, Whitton M, Brooks FP (2002) Physiological measures of presence in stressful virtual environments. ACM Trans Graphics 21(3):645–652
Mockel S, Preston RC, Herring J, Fitter NT (2024) A pilot investigation of human preference for robot arm visual form. In: Companion of the 2024 ACM/IEEE international conference on human-robot interaction, pp. 765–768
Moyer CA, Seefeldt L, Mann ES, Jackley LM (2011) Does massage therapy reduce cortisol? a comprehensive quantitative review. J Bodyw Mov Ther 15(1):3–14
Onishi Y, Sumioka H, Shiomi M (2024) Moffuly-ii: a robot that hugs and rubs heads. Int J Soc Robot 16(2):299–309
Paul A, Usman J, Ahmad MY, Hamidreza M, Maryam H, Ong ZC, Hasikin K, Lai KW (2021) Health efficacy of electrically operated automated massage on muscle properties, peripheral circulation, and physio-psychological variables: a narrative review. EURASIP J Adv Signal Process 2021:1–22
Pimentel D, Vinkers C (2021) Copresence with virtual humans in mixed reality: the impact of contextual responsiveness on social perceptions. Front Robot AI 8:634520
Poppendieck W, Wegmann M, Ferrauti A, Kellmann M, Pfeiffer M, Meyer T (2016) Massage and performance recovery: a meta-analytical review. Sports Med 46:183–204
Powell V, Powell W (2015) Therapy-led design of home-based virtual rehabilitation. In: 2015 IEEE 1st workshop on everyday virtual reality (WEVR). IEEE, pp. 11–14
Reis J, Melão N, Salvadorinho J, Soares B, Rosete A (2020) Service robots in the hospitality industry: The case of Henn-na hotel, Japan. Technol Soc 63:101423
Ren Q, Belpaeme T (2024) Tactile interaction with social robots influences attitudes and behaviour. Int J Soc Robot 16(11):2297–2317
Riva G (2003) Virtual environments in clinical psychology. Psychother: Theory, Res, Pract, Train, 40(1-2): 68
Riva G, Mantovani F, Capideville CS, Preziosa A, Morganti F, Villani D, Gaggioli A, Botella C, Alcañiz M (2007) Affective interactions using virtual reality: the link between presence and emotions. Cyberpsychol Behav 10(1):45–56
Scapin S, Echevarría-Guanilo ME, Junior PRBF, Gonçalves N, Rocha PK, Coimbra R (2018) Virtual reality in the treatment of burn patients: a systematic review. Burns 44(6):1403–1416
Schrepp M, Hinderks A, et al. (2017) Design and evaluation of a short version of the user experience questionnaire (ueq-s)
Shao D, Lee I-J (2020) Acceptance and influencing factors of social virtual reality in the urban elderly. Sustainability 12(22):9345
Slevin E, McCLELLAND A (1999) Multisensory environments: are they therapeutic? a single-subject evaluation of the clinical effectiveness of a multisensory environment. J Clin Nurs 8(1):48–56
Steptoe, W., Steed, A.: High-fidelity avatar eye-representation. In: Proceedings of the ACM symposium on virtual reality software and technology, pp. 83–90 (2012)
Suzuki R, Hedayati H, Zheng C, Bohn JL, Szafir D, Do EY-L, Gross MD, Leithinger D (2020) Roomshift: room-scale dynamic haptics for vr with furniture-moving swarm robots. In: Proceedings of the 2020 CHI conference on human factors in computing systems, pp. 1–11
Syrdal DS, Dautenhahn K, Woods S, Walters ML, Koay KL (2006) ’doing the right thing wrong’-personality and tolerance to uncomfortable robot approaches. In: ROMAN 2006-The 15th IEEE International symposium on robot and human interactive communication. IEEE, pp. 183–188
Vonach E, Gatterer C, Kaufmann H (2017) Vrrobot: Robot actuated props in an infinite virtual environment. In: 2017 IEEE Virtual Reality (VR). IEEE, pp. 74–83
Wagner D, Billinghurst M, Schmalstieg D (2006) How real should virtual characters be? In: Proceedings of the 2006 ACM SIGCHI international conference on advances in computer entertainment technology, p. 57
Watson D, Clark LA, Tellegen A (1988) Development and validation of brief measures of positive and negative affect: the panas scales. J Pers Soc Psychol 54(6):1063
Wijntjes MWA, Doorn GH, Kappers AML (2009) Haptic perception disambiguates visual perception of 3d shape. Exp Brain Res 193(4):639–644
Xiao M, Zhang T, Zou Y, Yan X, Wu W (2023) Study on force control for robot massage with a model-based reinforcement learning algorithm. Intel Serv Robot 16(4):509–519
Yilmaz Yelvar GD, Çırak Y, Dalkılınç M, Parlak Demir Y, Guner Z, Boydak A (2017) Is physiotherapy integrated virtual walking effective on pain, function, and kinesiophobia in patients with non-specific low-back pain? randomised controlled trial. Eur Spine J 26:538–545
Zhao Y, Kim LH, Wang Y, Le Goc M, Follmer S (2017) Robotic assembly of haptic proxy objects for tangible interaction and virtual reality. In: Proceedings of the 2017 ACM international conference on interactive surfaces and spaces, pp. 82–91
Acknowledgements
We would like to thank Haiyan Jiang, Jie Guo, Yuchuan Tang, and Yidan Pan for their valuable suggestions on preliminary experiments, analysis, and assistance in organizing the experiments. We also extend our appreciation to Limber Company and SoulShell Company for their support in providing experimental equipment and facilities.
Funding
This work was supported by the National Key R&D Program of China (2022YFF0902303).
Author information
Authors and Affiliations
Contributions
The authors confirm contribution to the paper as follows: Y.B.: Conceptualization, Methodology, Project administration, Hardware and Software Development, Experiment Execution, Data Collection and Formal Analysis, Visualization, Journal Communication, Writing—original draft, Writing—review & editing, Funding acquisition. D.W.: Conceptualization, Supervision, Project administration, Technical Guidance, Experimental Design, System Architecture Review, Writing—review & editing, Journal Communication, Funding acquisition. J.C.: Formal Analysis, Software Development, Visualization, Writing—review & editing. N.G.: Methodology, Experiment Execution, Data Interpretation, System Testing. X.D.: Theoretical Support, Formal Analysis, Writing—review & editing, Funding acquisition. M.S.: Technical Guidance, Hardware Integration, Resources, Visualization.
Corresponding author
Ethics declarations
Conflict of interest
The authors declare that they have no conflict of interest.
Ethical approval
This study was conducted in accordance with the principles specified in the Declaration of Helsinki and was approved by the Research Ethics Committee of Beijing Institute of Technology (2024-255). Written informed consent was obtained from all participants prior to the experiment.
Additional information
Publisher's Note
Springer Nature remains neutral with regard to jurisdictional claims in published maps and institutional affiliations.
Rights and permissions
Open Access This article is licensed under a Creative Commons Attribution-NonCommercial-NoDerivatives 4.0 International License, which permits any non-commercial use, sharing, distribution and reproduction in any medium or format, as long as you give appropriate credit to the original author(s) and the source, provide a link to the Creative Commons licence, and indicate if you modified the licensed material. You do not have permission under this licence to share adapted material derived from this article or parts of it. The images or other third party material in this article are included in the article’s Creative Commons licence, unless indicated otherwise in a credit line to the material. If material is not included in the article’s Creative Commons licence and your intended use is not permitted by statutory regulation or exceeds the permitted use, you will need to obtain permission directly from the copyright holder. To view a copy of this licence, visit http://creativecommons.org/licenses/by-nc-nd/4.0/.
About this article
Cite this article
Bao, Y., Weng, D., Chen, J. et al. Relaxmr: a mixed reality-based semi-physical high-fidelity digital human massage system. Virtual Reality 29, 52 (2025). https://doi.org/10.1007/s10055-025-01133-0
Received:
Accepted:
Published:
DOI: https://doi.org/10.1007/s10055-025-01133-0