Abstract
Despite being the most rapidly evolving biometric trait, the ear suffers from a few drawbacks, such as being affected by posture, illumination, and scaling in the two-dimensional domain. To address these issues, researchers focused on the 3D domain, as the intrinsic features of the 3D ear have significantly contributed to performance enhancement. However, it has also been observed that combining 2D and 3D ear features improves recognition better than using 3D ear features alone. This article presents a hybrid descriptor where the feature vectors for ear recognition are derived from the multimodal ear using classical and learning-based approaches. The classical approach generates features using a covariance matrix, whereas the learning-based approach uses a deep auto-encoder to generate features. Further, these features are combined to create a hybrid descriptor. Thorough experiments were carried out on the largest publicly accessible ear database to demonstrate the effectiveness of the proposed approach and compare its performance to that of state-of-the-art techniques.















Similar content being viewed by others
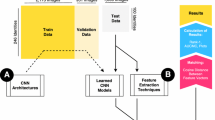
References
Alagarsamy SB, Kondappan S (2020) Ear recognition system using adaptive approach runge–kutta (aark) threshold segmentation with anfis classification. Neural Comput Appl 32(15):10,995–11,006
Alshazly H, Linse C, Barth E et al (2019) Handcrafted versus CNN features for ear recognition. Symmetry 11(12):1493
Alshazly H, Linse C, Barth E et al (2020) Deep convolutional neural networks for unconstrained ear recognition. IEEE Access 8:170,295–170,310
Asthana R, Walia GS, Gupta A (2021) Random area-perimeter method for generation of unimodal and multimodal cancelable biometric templates. Appl Intell:1–17
Besl PJ, McKay ND (1992) Method for registration of 3-D shapes. In: Sensor fusion IV: control paradigms and data structures, pp 586–607
Chen H, Bhanu B (2007) Human ear recognition in 3D. IEEE Trans Pattern Anal Mach Intell 29(4):718–737
Chen L, Mu Z, Nan B et al (2017) Tdsift: a new descriptor for 2D and 3D ear recognition. In: Proceedings of eighth international conference on graphic and image processing (ICGIP), pp 102,250C–102,250C–5
Cirujeda P, Cid Y D, Mateo X, et al. (2015) A 3D scene registration method via covariance descriptors and an evolutionary stable strategy game theory solver. Int J Comput Vis 115(3):306– 329
Emeršič ž, Štruc V, Peer P (2017) Ear recognition: more than a survey. Neurocomputing 255:26–39
Emeršič ž, Meden B, Peer P et al (2020) Evaluation and analysis of ear recognition models: performance, complexity and resource requirements. Neural Comput Appl 32(20):15,785–15,800
Fang Y, Xie J, Dai G et al (2015) 3D deep shape descriptor. In: Proceedings of the IEEE Conference on Computer Vision and Pattern Recognition, pp 2319–2328
Fischler MA, Bolles RC (1981) Random sample consensus: a paradigm for model fitting with applications to image analysis and automated cartography. Commun ACM 24(6):381–395
Ganapathi II, Prakash S (2018) 3D ear recognition using global and local features. IET Biometrics 7(3):232–241
Ganapathi II, Prakash S, Dave I R et al (2018) Ear recognition in 3D using 2D curvilinear features. IET Biometrics 7(6):519– 529
Ganapathi II, Ali SS, Prakash S (2020) Geometric statistics-based descriptor for 3D ear recognition. Vis Comput 36(1):161–173
Gao L, Zhang H, Gao Z et al (2020a) Texture semantically aligned with visibility-aware for partial person re-identification. In: Proceedings of the 28th ACM International Conference on Multimedia, pp 3771–3779
Gao Z, Gao L, Zhang H et al (2020b) Dcr: a unified framework for holistic/partial person reid. IEEE Trans Multimedia 23:3332–3345
Guo Y, Bennamoun M, Sohel F et al (2014) 3D object recognition in cluttered scenes with local surface features: a survey. IEEE Trans Pattern Anal Mach Intell 36(11):2270–2287
Islam S, Bennamoun M, Mian A et al (2008) A fully automatic approach for human recognition from profile images using 2D and 3D ear data. In: Proceedings of international symposium on 3D data processing visualization and transmission (3DPVT 2008)
Islam SM, Davies R, Bennamoun M et al (2011) Efficient detection and recognition of 3D ears. Int J Comput Vis 95(1):52–73
Koenderink JJ, Van Doorn AJ (1992) Surface shape and curvature scales. Image Vis Comput 10(8):557–564
Kumar N, Singh S, Kumar A (2018) Random permutation principal component analysis for cancelable biometric recognition. Appl Intell 48(9):2824–2836
Moolla Y, De Kock A, Mabuza-Hocquet G et al (2021) Biometric recognition of infants using fingerprint, iris, and ear biometrics. IEEE Access 9:38,269–38,286
Mursalin M, Islam SMS (2020) Epnet: a deep neural network for ear detection in 3D point clouds. In: International Conference on Advanced Concepts for Intelligent Vision Systems, pp 15–26
Nejati H, Zhang L, Sim T et al (2012) Wonder ears: identification of identical twins from ear images. In: Proceedings of international conference on pattern recognition (ICPR 2012), pp 1201– 1204
Ojala T, Pietikainen M, Harwood D (1994) Performance evaluation of texture measures with classification based on kullback discrimination of distributions. In: Proceedings of international conference on pattern recognition (ICPR 1994), pp 582–585
Prakash S, Gupta P (2014) Human recognition using 3D ear images. Neurocomputing 140:317–325
Prakash S, Gupta P (2015) Ear biometrics in 2D and 3D: localization and recognition, vol 10. Springer, Berlin
Priyadharshini RA, Arivazhagan S, Arun M (2021) A deep learning approach for person identification using ear biometrics. Appl Intell 51(4):2161–2172
Radhika K, Devika K, Aswathi T et al (2020) Performance analysis of nasnet on unconstrained ear recognition. In: Nature Inspired Computing for Data Science. Springer, pp 57–82
Salti S, Tombari F, Di Stefano L (2014) Shot: unique signatures of histograms for surface and texture description. Comput Vis Image Underst 125:251–264
Srivastava P, Agrawal D, Bansal A (2020) Ear detection and recognition techniques: a comparative review. In: Advances in Data and Information Sciences. pp 533–543
Sun X, Wang G, Wang L et al (2014) 3D ear recognition using local salience and principal manifold. Graph Model 76(5):402–412
Tarek M, Hamouda E, Abohamama A (2021) Multi-instance cancellable biometrics schemes based on generative adversarial network. Appl Intell:1–13
Toprak I, Toygar Ö (2021) Detection of spoofing attacks for ear biometrics through image quality assessment and deep learning. Expert Syst Appl 172:114,600
Wang Z, Yang J, Zhu Y (2021) Review of ear biometrics. Archives Comput Method Eng 28(1):149–180
Yaman D, Eyiokur FI, Ekenel HK (2021) Multimodal soft biometrics: combining ear and face biometrics for age and gender classification. Multimed Tools Appl:1–19
Yan P, Bowyer KW (2007) Biometric recognition using 3D ear shape. IEEE Trans Pattern Anal Mach Intell 29(8):1297–1308
Zhang J, Yu W, Yang X et al (2019) Few-shot learning for ear recognition. In: Proceedings of international conference on image, video and signal processing (IVSP), pp 50–54
Zhang Y, Mu Z, Yuan L et al (2017) 3D ear normalization and recognition based on local surface variation. Appl Sci 7(1):104
Zhou J, Cadavid S, Abdel-Mottaleb M (2011) A computationally efficient approach to 3D ear recognition employing local and holistic features. In: Proceedings of IEEE computer society conference on computer vision and pattern recognition workshop (CVPRW 2011), pp 98–105
Zhu Q, Mu Z (2020) Pointnet++ and three layers of features fusion for occlusion three-dimensional ear recognition based on one sample per person. Symmetry 12(1):78
Author information
Authors and Affiliations
Corresponding author
Additional information
Publisher’s note
Springer Nature remains neutral with regard to jurisdictional claims in published maps and institutional affiliations.
Rights and permissions
Springer Nature or its licensor holds exclusive rights to this article under a publishing agreement with the author(s) or other rightsholder(s); author self-archiving of the accepted manuscript version of this article is solely governed by the terms of such publishing agreement and applicable law.
About this article
Cite this article
Ganesan, K., A, C., Ganapathi, I.I. et al. Multimodal hybrid features in 3D ear recognition. Appl Intell 53, 11618–11635 (2023). https://doi.org/10.1007/s10489-022-04071-5
Accepted:
Published:
Issue Date:
DOI: https://doi.org/10.1007/s10489-022-04071-5