Abstract
An automatic sedimentary microfacies identification technique is developed based on the deep process neural network (DPNN), which consist several neurons and general non-time-varying neurons arranged in a certain topological structure. In this technique, the features of the shape and amplitude of logging curves are considered to form the category outputs. Combined with the deep learning theory, the diversity of the process features of logging curves and the complexity of combined features of multiple geophysical logging information are considered, and DPNN is created through the stacked superimposition of deep belief network and BP classifier. The technique maintains the structure and information relevance of process signal data and can characterize the distribution features of logging curves automatically, and classify the process signals directly. The theoretical nature and performance of the improved algorithm is tested and validated by some field examples.






Similar content being viewed by others
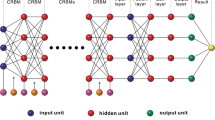
References
Ma, S.: Mathematic method for quantitative automatic identification of logging microfacies. Oil Geophys. Prospect. 35(5), 582–589 (2000)
Liu, H.Q., Chen, P., Xia, H.Q.: Automatic identification of sedimentary microfacies with log data and its application. Well Logging Technol. 30(3), 233–236 (2006)
Villagran, X.S., Balbo, A.L., Madella, M., et al.: Stratigraphic and spatial variability in shell middens: microfacies identification at the ethnohistoric site Tunel VII (Tierra del Fuego, Argentina). Archaeol. Anthropol. Sci. 3(4), 357–378 (2011)
Lu, S., Pan, H., Shuguang, P., et al.: Auto-identified system and study of sedimentary microfacies and elextrofacies—taking snaking stream deposition as an example. Chin. J. Eng. Geophys. 3, 022 (2009)
Valentini, G.L.: An overview of energy efficiency techniques in cluster computing systems. Clust. Comput. 16(1), 3–15 (2013)
Wang, J.R., Liu, H.T.: Identification of sedimentary microfacies of logging and its application. J. Daqing Pet. Inst. 28, 18–20 (2004)
Shi, Y., Liang, X., Mao, Z., et al.: An identification method for diagenetic facies with well logs and its geological significance in low-permeability sandstones: a case study on Chang 8 reservoirs in the Jiyuan region. Ordos Basin. Acta Petrolei Sinica 32(5), 820–828 (2011)
Ye, J., Xu, Z., Ding, Y.: Secure outsourcing of modular exponentiations in cloud and cluster computing. Clust. Comput. 19(2), 811–820 (2016)
Zhang, F., Hong, L.I., Shao, C., et al.: Application of artificial neural network pattern recognition technology to the study of well-logging sedimentology. Pet. Explor. Dev. 30(3), 121–123 (2003)
Cao, J., Chan, A.T., Sun, Y., et al.: A taxonomy of application scheduling tools for high performance cluster computing. Clust. Comput. 9(3), 355–371 (2006)
Cancan, W.U., Zhuangfu, L.I.: Logging facies analysis and sedimentary facies identification based on BP neural network. Coal Geol. Explor. 40(1), 68–71 (2012)
Li, D., Lu, D., Kong, X., et al.: Processing of well log data based on backpropagation neural network implicit approximation. Acta Petrolei Sinica 28(3), 105–108 (2007)
Xu, S.: Sedimentary facies identification based on genatic-BP algorithm and image process. Acta Petrolei Sinica 23(3), 48–51 (2002)
Hinton, G.E., Osindero, S., Teh, Y.W.: A Fast Learning Algorithm for Deep Belief Nets, p. 1527. MIT Press, Cambridge (2006)
Zhou, Q.: Research on heterogeneous data integration model of group enterprise based on cluster computing. Clust. Comput. 19(3), 1–8 (2016)
He, X., Liang, J.: Some theoretical issues on procedure neural networks. Eng. Sci. 2(12), 40–44 (2000)
Acknowledgements
This research was partially supported by the Natural Science Foundation of China (41404089).
Author information
Authors and Affiliations
Corresponding author
Rights and permissions
About this article
Cite this article
Liu, H., Xu, S., Ge, X. et al. Automatic sedimentary microfacies identification from logging curves based on deep process neural network. Cluster Comput 22 (Suppl 5), 12451–12457 (2019). https://doi.org/10.1007/s10586-017-1656-z
Received:
Revised:
Accepted:
Published:
Issue Date:
DOI: https://doi.org/10.1007/s10586-017-1656-z