Abstract
To improve precision for model identification result of pumped storage motor, a kind of multivariable bilinear subspace recursive likelihood identification for pumped storage motor is put forward. Firstly, pumped storage motor magnetic filed equation is given, pumped storage motor model based on extension Kalman Filter is established, two-set identification models are constructed by coordination by utilizing two-group state vectors of resistance and inductance and two-set models work cooperatively to construct circulation identification algorithm; then, introduce subspace identification strategy, establish relevant data estimation equation by utilizing block Hankel matrix and realize valid identification to system parameter matrix by adopting least squares (LS); validity of proposed algorithm is verified by simulation experiment in matlab platform and hardware environment.







Similar content being viewed by others
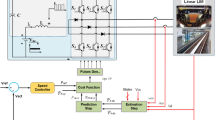
References
Shen, Y., Ding, S.X., Haghani, A., et al.: A comparison study of basic data-driven fault diagnosis and process monitoring methods on the benchmark Tennessee Eastman process. J. Process Control 22(9), 1567–1581 (2012)
Efendiev, Y., Galvis, J., Lazarov, R., et al.: Robust domain decomposition preconditioners for abstract symmetric positive definite bilinear forms. Esaim Math. Model. Numer. Anal. 46(5), 1175–1199 (2011)
Taieb, S.B., Bontempi, G., Atiya, A.F., et al.: A review and comparison of strategies for multi-step ahead time series forecasting based on the NN5 forecasting competition. Expert Syst. Appl. 39(8), 7067–7083 (2011)
Altmann, Y., Dobigeon, N., Tourneret, J.Y.: Nonlinearity detection in hyperspectral images using a polynomial post-nonlinear mixing model. IEEE Trans. Image Process. 22(4), 1267–1276 (2013)
Bosch, J., Kay, D., Stoll, M., et al.: Fast solvers for cahn-hilliard inpainting. Siam J. Imaging Sci. 7(1), 67–97 (2014)
Cui, T., Law, K.J.H., Marzouk, Y.M.: Dimension-independent likelihood-informed MCMC. J. Comput. Phys. 304(C), 109–137 (2015)
Dauge, M., Lafranche, Y., Raymond, N.: Quantum waveguides with corners. Esaim Proc. 35, 14–45 (2011)
Ding, F., Wang, X., Chen, Q., et al.: Recursive least squares parameter estimation for a class of output nonlinear systems based on the model decomposition. Circuit. Syst. Signal Process. 35(9), 1–16 (2016)
Dong, J., Curtmola, R., Nitarotaru, C., et al.: Pollution attacks and defenses in wireless interflow network coding systems. IEEE Trans. Dependable Secure Comput. 9(5), 741–755 (2012)
Burazerovic, D., Heylen, R., Geens, B., et al.: Detecting the adjacency effect in hyperspectral imagery with spectral unmixing techniques. IEEE J. Sel. Top. Appl. Earth Obs. Remote Sens. 6(3), 1070–1078 (2013)
Bouc, S., Stancu, R., Thévenaz, J.: Simple biset functors and double Burnside ring. J. Pure Appl. Algebra 217(3), 546–566 (2012)
Liu, S., Cai, C., Zhu, Q., Arunkumar, N.: A study of software pools for seismogeology-related software based on the Docker technique. Int. J. Comput. Appl. (2017). https://doi.org/10.1080/1206212X.2017.1396429
Hamza, Rafik, Muhammad, Khan, Arunkumar, N., Ramírez González, G.: Hash based encryption for keyframes of diagnostic hysteroscopy. IEEE Access (2017). https://doi.org/10.1109/ACCESS.2017.2762405
Fernandes, S.L., Gurupur, V.P., Sunder, N.R., Arunkumar, N., Kadry, S.: A novel nonintrusive decision support approach for heart rate measurement. Pattern Recognit. Lett. (2017). https://doi.org/10.1016/j.patrec.2017.07.002
Arunkumar, N., Ramkumar, K., Venkatraman, V., Abdulhay, E., Fernandes, S.L., Kadry, S., Segal, S.: Classification of focal and non focal EEG using entropies. Pattern Recognit. Lett. 94, 112–117 (2017)
Arunkumar, N., Kumar, K.R., Venkataraman, V.: Automatic detection of epileptic seizures using new entropy measures. J. Med. Imaging Health Inf. 6(3), 724–730 (2016)
Arunkumar, N., Ram Kumar, K., Venkataraman, V.: Automatic detection of epileptic seizures using permutation entropy, Tsallis entropy and Kolmogorov complexity. J. Med. Imaging Health Inf. 6(2), 526–531 (2016)
Acknowledgements
National Natural Science Foundation of China (51407050).
Author information
Authors and Affiliations
Corresponding author
Rights and permissions
About this article
Cite this article
Xu, Z., Bao-Jun, G. & Dajun, T. Multivariable bilinear subspace recursive likelihood identification for pumped storage motor. Cluster Comput 22 (Suppl 2), 4527–4533 (2019). https://doi.org/10.1007/s10586-018-2066-6
Received:
Revised:
Accepted:
Published:
Issue Date:
DOI: https://doi.org/10.1007/s10586-018-2066-6