Abstract
This study aims to determine indicators that affect students' final performance in an online learning environment using predictive learning analytics in an ICT course and Turkey context. The study takes place within a large state university in an online computer literacy course (14 weeks in one semester) delivered to freshmen students (n = 1209). The researcher gathered data from Moodle engagement analytics (time spent in course, number of clicks, exam, content, discussion), assessment grades (pre-test for prior knowledge, final grade), and various scales (technical skills and "motivation and attitude" dimensions of the readiness, and self-regulated learning skills). Data analysis used multi regression and classification. Multiple regression showed that prior knowledge and technical skills predict the final performance in the context of the course (ICT 101). According to the best probability, the Decision Tree algorithm classified 67.8% of the high final performance based on learners' characteristics and Moodle engagement analytics. The high level of total system interactions of learners with low-level prior knowledge increases their probability of high performance (from 40.4 to 60.2%). This study discussed the course structure and learning design, appropriate actions to improve performance, and suggestions for future research based on the findings.










Similar content being viewed by others
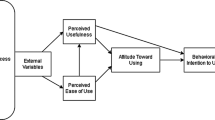
Explore related subjects
Discover the latest articles, news and stories from top researchers in related subjects.Data Availability Statement
The datasets generated during and/or analyzed during the current study are available from the corresponding author on reasonable request.
References
Aguilar, S. J., Karabenick, S. A., Teasley, S. D., & Baek, C. (2021). Associations between learning analytics dashboard exposure and motivation and self-regulated learning. Computers & Education, 162, 104085. https://doi.org/10.1016/j.compedu.2020.104085
Avella, J. T., Kebritchi, M., Nunn, S. G., & Kanai, T. (2016). Learning analytics methods, benefits, and challenges in higher education: A systematic literature review. Online Learning, 20(2), 13–29. https://doi.org/10.24059/olj.v20i2.790
Azevedo, R., Moos, D. C., Johnson, A. M., & Chauncey, A. D. (2010). Measuring cognitive and metacognitive regulatory processes during hypermedia learning: Issues and challenges. Educational Psychologist, 45(4), 210–223. https://doi.org/10.1080/00461520.2010.515934
Chatti, M. A., Dyckhoff, A. L., Schroeder, U., & Thüs, H. (2012). A reference model for learning analytics. International Journal of Technology Enhanced Learning, 4(5–6), 318–331. https://doi.org/10.1504/IJTEL.2012.051815
Clow, D. (2013). An overview of learning analytics. Teaching in Higher Education, 18(6), 683–695. https://doi.org/10.1080/13562517.2013.827653
Conijn, R., Snijders, C., Kleingeld, A., & Matzat, U. (2017). Predicting student performance from LMS data: A comparison of 17 blended courses using Moodle LMS. IEEE Transactions on Learning Technologies, 10(1), 17–29. https://doi.org/10.1109/TLT.2016.2616312
Council of Higher Education. (2020). Procedures and principles regarding distance education in higher education ınstitutions [Yükseköğretim Kurumlarında Uzaktan Öğretime İlişkin Usul Ve Esaslar]. Retrieved from https://t.ly/xX7H
Cui, Y., Chen, F., & Shiri, A. (2020). Scale up predictive models for early detection of at-risk students: A feasibility study. Information and Learning Sciences, 121(3/4), 97–116. https://doi.org/10.1108/ILS-05-2019-0041
De Barba, P. G., Kennedy, G. E., & Ainley, M. D. (2016). The role of students’ motivation and participation in predicting performance in a MOOC. Journal of Computer Assisted Learning, 32(3), 218–231. https://doi.org/10.1111/jcal.12130
Du, X., Yang, J., Shelton, B. E., Hung, J. L., & Zhang, M. (2019). A systematic meta-review and analysis of learning analytics research. Behaviour & Information Technology. https://doi.org/10.1080/0144929X.2019.1669712
Duffy, M. C., & Azevedo, R. (2015). Motivation matters: Interactions between achievement goals and agent scaffolding for self-regulated learning within an intelligent tutoring system. Computers in Human Behavior, 52, 338–348. https://doi.org/10.1016/j.chb.2015.05.041
Er, E., Gómez-Sánchez, E., Dimitriadis, Y., Bote-Lorenzo, M. L., Asensio-Pérez, J. I., & Álvarez-Álvarez, S. (2019). Aligning learning design and learning analytics through instructor involvement: A MOOC case study. Interactive Learning Environments, 27(5–6), 685–698.
Gašević, D., Dawson, S., Rogers, T., & Gašević, D. (2016). Learning analytics should not promote one size fits all: The effects of instructional conditions in predicting learning success. The Internet and Higher Education, 28, 68–84. https://doi.org/10.1016/j.iheduc.2015.10.002
Gülbahar, Y. (2012). Study of developing scales for assessment of the levels of readiness and satisfaction of participants in e-learning environments. Ankara University, Journal of Faculty of Educational Sciences, 45(2), 119–137. https://doi.org/10.1501/Egifak_0000001256
Hair, J. F., Black, W. C., Babin, B. J., & Anderson, R. E. (2010). Advanced diagnostics for multiple regression: A supplement to multivariate data analysis. Prentice Hall.
Helal, S., Li, J., Liu, L., Ebrahimie, E., Dawson, S., Murray, D. J., & Long, Q. (2018). Predicting academic performance by considering student heterogeneity. Knowledge-Based Systems, 161, 134–146. https://doi.org/10.1016/j.knosys.2018.07.042
Herodotou, C., Naydenova, G., Boroowa, A., Gilmour, A., & Rienties, B. (2020). How can predictive learning analytics and motivational ınterventions ıncrease student retention and enhance administrative support in distance education? Journal of Learning Analytics, 7(2), 72–83. https://doi.org/10.18608/jla.2020.72.4
Holmes, W., Nguyen, Q., Zhang, J., Mavrikis, M., & Rienties, B. (2019). Learning analytics for learning design in online distance learning. Distance Education, 40(3), 309–329. https://doi.org/10.1080/01587919.2019.1637716
Horzum, M. B., Kaymak, Z. D., & Gungoren, O. C. (2015). Structural equation modeling towards online learning readiness, academic motivations, and perceived learning. Educational Sciences: Theory and Practice, 15(3), 759–770. https://doi.org/10.12738/estp.2015.3.2410
Hung, J. L., Shelton, B. E., Yang, J., & Du, X. (2019). Improving predictive modeling for at-risk student identification: A multistage approach. IEEE Transactions on Learning Technologies, 12(2), 148–157. https://doi.org/10.1109/TLT.2019.2911072
Ifenthaler, D., & Yau, J. Y. K. (2020). Utilising learning analytics to support study success in higher education: A systematic review. Educational Technology Research and Development, 68(4), 1961–1990. https://doi.org/10.1007/s11423-020-09788-z
Iglesias-Pradas, S., Ruiz-de-Azcárate, C., & Agudo-Peregrina, A. F. (2015). Assessing the suitability of student interactions from Moodle data logs as predictors of cross-curricular competencies. Computers in Human Behavior, 47, 81–89. https://doi.org/10.1016/j.chb.2014.09.065
Jivet, I., Scheffel, M., Schmitz, M., Robbers, S., Specht, M., & Drachsler, H. (2020). From students with love: An empirical study on learner goals, self-regulated learning and sense-making of learning analytics in higher education. The Internet and Higher Education, 47, 100758.
Joosten, T., & Cusatis, R. (2020). Online learning readiness. American Journal of Distance Education. https://doi.org/10.1080/08923647.2020.1726167
Kizilcec, R. F., Pérez-Sanagustín, M., & Maldonado, J. J. (2017). Self-regulated learning strategies predict learner behavior and goal attainment in massive open online courses. Computers & Education, 104, 18–33. https://doi.org/10.1016/j.compedu.2016.10.001
Kocdar, S., Karadeniz, A., Bozkurt, A., & Buyuk, K. (2018). Measuring self-regulation in self-paced open and distance learning environments. International Review of Research in Open and Distributed Learning, 19(1), 25–43. https://doi.org/10.19173/irrodl.v19i1.3255
Laurillard, D., Charlton, P., Craft, B., Dimakopoulos, D., Ljubojevic, D., Magoulas, G., Masterman, E., Pujadas, R., Whitley, E. A., & Whittlestone, K. (2013). A constructionist learning environment for teachers to model learning designs. Journal of Computer Assisted Learning, 29(1), 15–30. https://doi.org/10.1111/j.1365-2729.2011.00458.x
Leitner, P., Khalil, M., & Ebner, M. (2017). Learning analytics in higher education—A literature review. In A. Peña-Ayala (Ed.), Learning analytics: Fundaments, applications, and trends (pp. 1–23). Springer. https://doi.org/10.1007/978-3-319-52977-6_1
Liu, J. C. (2019). Evaluating online learning orientation design with a readiness scale. Online Learning, 23(4), 42–61. https://doi.org/10.24059/olj.v23i4.2078
Lockyer, L., & Dawson, S. (2011). Learning designs and learning analytics. In Proceedings of the 1st international conference on learning analytics and knowledge (pp. 153–156). https://doi.org/10.1145/2090116.2090140
Lu, C.-H., & Yu, C.-H. (2019). Online data stream analytics for dynamic environments using self-regularized learning framework. IEEE Systems Journal, 13(4), 3697–3707. https://doi.org/10.1109/JSYST.2019.2894697
Macayan, J. V. (2017). Implementing outcome-based education (OBE) framework: Implications for assessment of students’ performance. Educational Measurement and Evaluation Review, 8(1), 1–10.
Macfadyen, L. P., Lockyer, L., & Rienties, B. (2020). Learning design and learning analytics: Snapshot 2020. Journal of Learning Analytics, 7(3), 6–12. https://doi.org/10.18608/JLA.2020.73.2
Mangaroska, K., & Giannakos, M. (2019). Learning analytics for learning design: A systematic literature review of analytics-driven design to enhance learning. IEEE Transactions on Learning Technologies, 12(4), 516–534. https://doi.org/10.1109/TLT.2018.2868673
Miranda, S., & Vegliante, R. (2019). Learning analytics to support learners and teachers: The navigation among contents as a model to adopt. Journal of e-Learning and Knowledge Society, 15(3), 101–116. https://doi.org/10.20368/1971-8829/1135065
Moos, D. C., & Azevedo, R. (2008). Self-regulated learning with hypermedia: The role of prior domain knowledge. Contemporary Educational Psychology, 33(2), 270–298. https://doi.org/10.1016/j.cedpsych.2007.03.001
Moreno-Marcos, P. M., Munoz-Merino, P. J., Maldonado-Mahauad, J., Perez-Sanagustin, M., Alario-Hoyos, C., & Kloos, C. D. (2020). Temporal analysis for dropout prediction using self-regulated learning strategies in self-paced MOOCs. Computers & Education, 145, 103728. https://doi.org/10.1016/j.compedu.2019.103728
Mwalumbwe, I., & Mtebe, J. S. (2017). Using learning analytics to predict students’ performance in Moodle learning management system: A case of Mbeya University of Science and Technology. The Electronic Journal of Information Systems in Developing Countries, 79(1), 1–13. https://doi.org/10.1002/j.1681-4835.2017.tb00577.x
Namoun, A., & Alshanqiti, A. (2021). Predicting student performance using data mining and learning analytics techniques: A systematic literature review. Applied Sciences, 11(1), 237. https://doi.org/10.3390/app11010237
Olive, D. M., Huynh, D. Q., Reynolds, M., Dougiamas, M., & Wiese, D. (2019). A quest for a one-size-fits-all neural network: Early prediction of students at risk in online courses. IEEE Transactions on Learning Technologies, 12(2), 171–183. https://doi.org/10.1109/TLT.2019.2911068
Omedes, J. (2018). Learning analytics 2018—An updated perspective. IAD Learning. https://www.iadlearning.com/learning-analytics-2018/
Papamitsiou, Z., & Economides, A. A. (2014). Temporal learning analytics for adaptive assessment. Journal of Learning Analytics, 1(3), 165–168. https://doi.org/10.18608/jla.2014.13.13
Papamitsiou, Z., & Economides, A. A. (2019). Exploring autonomous learning capacity from a self-regulated learning perspective using learning analytics. British Journal of Educational Technology, 50(6), 3138–3155. https://doi.org/10.1111/bjet.12747
Pardo, A., Han, F., & Ellis, R. A. (2016). Combining university student self-regulated learning indicators and engagement with online learning events to predict academic performance. IEEE Transactions on Learning Technologies, 10(1), 82–92. https://doi.org/10.1109/TLT.2016.2639508
Premalatha, K. (2019). Course and program outcomes assessment methods in outcome-based education: A review. Journal of Education, 199(3), 111–127. https://doi.org/10.1177/0022057419854351
Ramirez-Arellano, A., Bory-Reyes, J., & Hernández-Simón, L. M. (2019). Emotions, motivation, cognitive–metacognitive strategies, and behavior as predictors of learning performance in blended learning. Journal of Educational Computing Research, 57(2), 491–512. https://doi.org/10.1177/0735633117753935
Saqr, M., Fors, U., & Tedre, M. (2017). How learning analytics can early predict under-achieving students in a blended medical education course. Medical Teacher, 39(7), 757–767. https://doi.org/10.1080/0142159X.2017.1309376
Schumacher, C., & Ifenthaler, D. (2021). Investigating prompts for supporting students’ self-regulation—A remaining challenge for learning analytics approaches? The Internet and Higher Education, 49, 100791. https://doi.org/10.1016/j.iheduc.2020.100791
Shulruf, B., Bagg, W., Begun, M., Hay, M., Lichtwark, I., Turnock, A., Warnecke, E., Wilkinson, T. J., & Poole, P. J. (2018). The efficacy of medical student selection tools in Australia and New Zealand. Medical Journal of Australia, 208(5), 214–218. https://doi.org/10.5694/mja17.00400
Strang, K. D. (2016). Do the critical success factors from learning analytics predict student outcomes? Journal of Educational Technology Systems, 44(3), 273–299. https://doi.org/10.1177/0047239515615850
Strang, K. D. (2017). Beyond engagement analytics: Which online mixed-data factors predict student learning outcomes? Education & Information Technology, 22, 917–937. https://doi.org/10.1007/s10639-016-9464-2
Sun, J. C. Y., Lin, C. T., & Chou, C. (2018). Applying learning analytics to explore the effects of motivation on online students’ reading behavioral patterns. International Review of Research in Open and Distributed Learning. https://doi.org/10.19173/irrodl.v19i2.2853
van Merriënboer, J. J. G., & Kirschner, P. A. (2017). Ten steps to complex learning: A systematic approach to four-component instructional design. Routledge. https://doi.org/10.4324/9780203096864
Viberg, O., Khalil, M., & Baars, M. (2020). Self-regulated learning and learning analytics in online learning environments: A review of empirical research. In Proceedings of the tenth international conference on learning analytics & knowledge (pp. 524–533). https://doi.org/10.1145/3375462.3375483
Wong, J., Baars, M., de Koning, B. B., van der Zee, T., Davis, D., Khalil, M., Houben, G., & Paas, F. (2019). Educational theories and learning analytics: From data to knowledge. In D. Ifenthaler, D.-K. Mah, & J.Y.-K. Yau (Eds.), Utilizing learning analytics to support study success (pp. 3–25). Springer. https://doi.org/10.1007/978-3-319-64792-0_1
Yau, J. Y. K., & Ifenthaler, D. (2020). Reflections on different learning analytics indicators for supporting study success. International Journal of Learning Analytics and Artificial Intelligence for Education: IJAI, 2(2), 4–23. https://doi.org/10.3991/ijai.v2i2.15639
Yilmaz, R. (2017). Exploring the role of e-learning readiness on student satisfaction and motivation in flipped classroom. Computers in Human Behavior, 70, 251–260. https://doi.org/10.1016/j.chb.2016.12.085
You, J. (2016). Identifying significant indicators using LMS data to pre- dict course achievement in online learning. The Internet and Higher Education, 29, 23–30. https://doi.org/10.1016/j.iheduc.2015.11.003
Zacharis, N. Z. (2015). A multivariate approach to predicting student outcomes in web-enabled blended learning courses. Internet and Higher Education, 27, 44–53. https://doi.org/10.1016/j.iheduc.2015.05.002
Acknowledgements
This research was supported and funded by the Scientific and Technological Research Council of Turkey (TUBİTAK).
Author information
Authors and Affiliations
Corresponding author
Ethics declarations
Conflict of interest
The authors have no conflicts of interest to declare that are relevant to the content of this article.
Ethical Approval
The authors of the current study have approved the manuscript for submission. All procedures performed in the current study involving human participants were in accordance with the ethical standards of the institutional research committee. With regard to ethical considerations, a consent form was prepared and explained to the participants both while collecting log data and applying the scales. Written informed consent was also obtained from the all participants prior to the research in LMS.
Additional information
Publisher's Note
Springer Nature remains neutral with regard to jurisdictional claims in published maps and institutional affiliations.
Rights and permissions
About this article
Cite this article
Yildirim, D., Gülbahar, Y. Implementation of Learning Analytics Indicators for Increasing Learners' Final Performance. Tech Know Learn 27, 479–504 (2022). https://doi.org/10.1007/s10758-021-09583-6
Accepted:
Published:
Issue Date:
DOI: https://doi.org/10.1007/s10758-021-09583-6