Abstract
Emotional voice conversion systems are used to formulate mapping functions to transform the neutral speech from output of text-to-speech systems to that of target emotion appropriate to the context. In this work, a learning-based hybrid model is proposed for speaker-independent emotional voice conversion using a combination of deep belief nets (DBN-DNN) and general regression neural net (GRNN). The main acoustic features considered for mapping are shape of the vocal tract given by line spectral frequencies (LSF), glottal excitation given by LP residual and long term prosodic features viz. pitch contour and energy. GRNN is used to attain the transformation function between source and target LSFs. Source and target LP residuals are subjected to wavelet transform before DBN-DNN training. This is helpful to remove phase-change induced distortions which may affect the performance of neural networks when transforming time-domain residual. Low-dimensional pitch (intonation) contour is subjected to feed-forward neural network mapping (ANN). Energy modification is achieved by taking average transformation scales across entire utterance. The system is tested on three different datasets viz. EmoDB (German), IITKGP (Telugu) and SAVEE (English). Relative performances of proposed model are compared with constrained variance GMM (CV-GMM) using objective and subjective metrics. The results obtained show a significant performance improvement of 41% in RMSE (Hz) and 9.72% in Pearson’s correlation coefficient for fundamental frequency (F0) (Fear) compared to CV-GMM across all 3 datasets. Subjective results indicate a maximum MOS score of 3.85 (Fear) and CMOS score of 3.9 (Happiness) across the three datasets considered.








Similar content being viewed by others
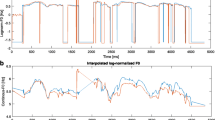
Explore related subjects
Discover the latest articles, news and stories from top researchers in related subjects.References
Aihara, R., Takashima, R., Takiguchi, T., & Ariki, Y. (2012). GMM-based emotional voice conversion using spectrum and prosody features. American Journal of Signal Processing, 2(5), 134–138.
Aihara, R., Ueda, R., Takiguchi, T., & Ariki, Y. (2014). Exemplar-based emotional voice conversion using non-negative matrix factorization. In Proceedings of APSIPA, 2014 (pp. 1–7). IEEE.
Akagi, M., Han, X., Elbarougy, R., Hamada, Y., & Li, J. (2014). Toward affective speech-to-speech translation: Strategy for emotional speech recognition and synthesis in multiple languages. In Proceedings of APSIPA, 2014 (pp. 1–10). IEEE.
Anon. (2013). Technology development for Indian languages programme. In Diety. Retrieved January 22, 2018 from http://tdil.mit.gov.in/AboutUs.aspx.
Benisty, H., & Malah, D. (2011). Voice conversion using GMM with enhanced global variance. In Proceedings of INTERSPEECH.
Burkhardt, F., Paeschke, A., Rolfes, M., Sendlemeier, W., & Weiss, B. (2011). A database of German emotional speech. In Proceedings of INTERSPEECH (pp. 1517–1520).
Burkhardt, F., & Sendlmeier, W. F. (2000). Verification of acoustical correlates of emotional speech using formant-synthesis. In ISCA tutorial and research workshop (ITRW) on speech and emotion.
Cabral, J. P., & Oliveira, L. C. (2006). Emovoice: A system to generate emotions in speech. In Proceedings of the ninth international conference on spoken language processing.
Cahn, J. (1990). The generation of affect in synthesized speech. Journal of the American Voice I/O Society, 8, 1–19.
Desai, S., Black, A. W., Yegnanarayana, B., & Prahallad, K. (2010). Spectral mapping using artificial neural networks for voice conversion. IEEE Transactions on Audio, Speech, and Language Processing, 18(5), 954–964.
Govind, D., & Prasanna, S. R. M. (2013). Dynamic prosody modification using zero frequency filtered signal. International Journal of Speech Technology, 16(1), 41–54.
Haq, S., & Jackson, P. (2009). Speaker-dependent audio-visual emotion recognition. In Proceedings of international conference on audio visual speech processing (pp. 53–58).
Haq, S., & Jackson, P. (2010). Multimodal emotion recognition. In W. Wang (Ed.), Machine audition: Principles, algorithms and systems. Hershey: IGI Global Press.
Haque, A., & Rao, K. S. (2017). Modification of energy spectra, epoch parameters and prosody for emotion conversion in speech. International Journal of Speech Technology, 20(1), 15–25.
Helander, E., Virtanen, T., Nurminen, J., & Gabbouj, M. (2010). Voice conversion using partial least squares regression. IEEE Transactions on Audio, Speech, and Language Processing, 18(5), 912–921.
Hinton, G. E., Osindero, S., & Teh, Y. (2006). A fast learning algorithm for deep belief nets. Neural Computation, 18(7), 1527–1554.
Hunt, A. J., & Black, A. W. (1996). Unit selection in a concatenative speech synthesis system using a large speech database. In Proceedings of ICASSP (Vol. 1, pp. 373–376). IEEE.
Inanoglu, Z., & Young, S. (2007). A system for transforming the emotion in speech: Combining data-driven conversion techniques for prosody and voice quality. In Eighth annual conference of the international speech communication association.
Kawahara, H., & Morise, M. (2011). Technical foundations of TANDEM-STRAIGHT, a speech analysis, modification and synthesis framework. Sadhana, 36(5), 713–727.
Kawanami, H., Iwami, Y., Toda, T., Saruwatari, H., & Shikano, K. (2003). GMM-based voice conversion applied to emotional speech synthesis. In Eighth European conference on speech communication and technology.
Koolagudi, S. G., Maity, S., Kumar, V. A., Chakrabarti, S., & Rao, K. S. (2009). IITKGP-SESC: Speech database for emotion analysis. In Proceedings of IC3 (pp. 485–492). Springer.
Krothapalli, S. R., Yadav, J., Sarkar, S., Koolagudi, S. G., & Vuppala, A. (2012). Neural network based feature transformation for emotion independent speaker identification. International Journal of Speech Technology, 15(3), 335–349.
Liu, K., Zhang, J., & Yan, Y. (2007). High quality voice conversion through phoneme-based linear mapping functions with STRAIGHT for mandarin. In Proceedings of the international conference on fuzzy systems and knowledge discovery (Vol. 4, pp. 410–414). IEEE.
Luo, Z., Chen, J., Takiguchi, T., & Ariki, Y. (2017a). Emotional voice conversion with adaptive scales F0 based on wavelet transform using limited amount of emotional data. In Proceedings of Interspeech (pp. 3399–3403).
Luo, Z., Chen, J., Takiguchi, T., & Ariki, Y. (2017b). Emotional voice conversion using neural networks with arbitrary scales F0 based on wavelet transform. EURASIP Journal on Audio, Speech, and Music Processing, 2017(1), 18.
Luo, Z., Takiguchi, T., & Ariki, Y. (2016). Emotional voice conversion using deep neural networks with MCC and F0 features. In Proceedings of ICIS (pp. 1–5). IEEE.
Ming, H., Huang, D., Dong, M., Li, H., Xie., L., & Zhang, S. (2015). Fundamental frequency modeling using wavelets for emotional voice conversion. In Proceedings of (ACII) (pp. 804–809). IEEE.
Ming, H., Huang, D., Xie, L., Zhang, S., Dong, M., & Li, H. (2016). Exemplar-based sparse representation of timbre and prosody for voice conversion. In Proceedings of ICASSP (pp. 5175–5179). IEEE.
Mohamed, A., Dahl, G., & Hinton, G. (2009). Deep belief networks for phone recognition. In NIPS workshop on deep learning for speech recognition and related applications (Vol. 1, p. 39). Vancouver, Canada.
Moulines, E., & Laroche, J. (1995). Non-parametric techniques for pitch-scale and time-scale modification of speech. Speech Communication, 16(2), 175–205.
Nakamura, K., Toda, T., Saruwatari, H., & Shikano, K. (2012). Speaking-aid systems using GMM-based voice conversion for electrolaryngeal speech. Speech Communication, 54(1), 134–146.
Nakashika, T., Takashima, R., Takiguchi, T., & Ariki, Y. (2013). Voice conversion in high-order eigen space using deep belief nets. In Proceedings of Interspeech (pp. 369–372).
Nirmal, J., Zaveri, M., Patnaik, S., & Kachare, P. (2014). Voice conversion using general regression neural network. Applied Soft Computing, 24, 1–12.
Přibil, J., & Přibilová, A. (2014). GMM-based evaluation of emotional style transformation in czech and slovak. Cognitive Computation, 6(4), 928–939.
Raitio, T., Suni, A., Juvela, L., Vainio, M., & Alku, P. (2014). Deep neural network based trainable voice source model for synthesis of speech with varying vocal effort. In 15th annual conference of international speech communication association
Ramırez, J., Segura, J. C., Benıtez, C., De La Torre, A., & Rubio, A. (2004). Efficient voice activity detection algorithms using long-term speech information. Speech Communication, 42(3–4), 271–287.
Rao, K. S., & Vuppala, A. K. (2013). Non-uniform time scale modification using instants of significant excitation and vowel onset points. Speech Communication, 55(6), 745–756.
Ribeiro, M. S., & Clark, R. A. (2015). A multi-level representation of F0 using the continuous wavelet transform and the discrete cosine transform. In Proceedings of ICASSP (pp. 4909–4913). IEEE.
Sarkar, P., Haque, A., Dutta, A. K., Reddy, G., Harikrishna, D., Dhara, P., et al. (2014). Designing prosody rule-set for converting neutral TTS speech to storytelling style speech for Indian languages: Bengali, Hindi and Telugu. In 2014 seventh international conference on contemporary computing (IC3) (pp. 473–477). IEEE.
Specht, D. F. (1991). A general regression neural network. IEEE Transactions on Neural Networks, 2(6), 568–576.
Stylianou, Y., Olivier, C., & Moulines, E. (1998). Continuous probabilistic transform for voice conversion. IEEE Transactions on Speech and Audio Processing, 6(2), 131–142.
Suni, A., Aalto, D., Raitio, T., Alku, P., Vainio, M., et al. (2013). Wavelets for intonation modeling in HMM speech synthesis. In Proceedings of the 8th ISCA workshop on speech synthesis, Barcelona, August 31–September 2.
Tanaka, M., & Okutomi, M. (2014). A novel inference of a restricted Boltzmann machine. In 22nd international conference on pattern recognition (ICPR) (pp. 1526–1531). IEEE.
Tao, J., Kang, Y., & Li, A. (2006). Prosody conversion from neutral speech to emotional speech. IEEE Transactions on Audio, Speech, and Language Processing, 14(4), 1145–1154.
Theune, M., Meijis, K., & Heylen, D. (2006). Generating expressive speech for storytelling applications. IEEE Transactions on Audio, Speech, and Language Processing, 14(4), 1137–1144.
Vainio, M., Suni, A., & Aalto, D. (2013). Continuous wavelet transform for analysis of speech prosody. In Proceedings of the TRASP 2013-tools and resources for the analysys of speech prosody.
Vegesna, V. V. R., Gurugubelli, K., & Vuppala, A. (2018). Prosody modification for speech recognition in emotionally mismatched conditions. International Journal of Speech Technology, 21, 521–532.
Vekkot, S. (2017). Building a generalized model for multi-lingual vocal emotion conversion. In Seventh international conference on affective computing and intelligent interaction, ACII 2017 (pp. 576–580), San Antonio, TX, USA, 23–26 October, 2017. https://doi.org/10.1109/ACII.2017.8273658.
Vekkot, S., & Gupta, D. (2019a). Emotion conversion in Telugu using constrained variance GMM and continuous wavelet transform-\(F_0\). In TENCON 2019-2019 IEEE Region 10 Conference (TENCON) (pp. 991–996). IEEE.
Vekkot, S., & Gupta, D. (2019b). Prosodic transformation in vocal emotion conversion for multi-lingual scenarios: A pilot study. International Journal of Speech Technology, 22(3), 533–549.
Vekkot, S., Gupta, D., Zakariah, M., & Alotaibi, Y. A. (2019). Hybrid framework for speaker-independent emotion conversion using i-vector PLDA and neural network. IEEE Access, 7, 81883–81902.
Vekkot, S., & Tripathi, S. (2016). Inter-emotion conversion using dynamic time warping and prosody imposition. In The international symposium on intelligent systems technologies and applications (pp. 913–924). Springer.
Vekkot, S., & Tripathi, S. (2016). Significance of glottal closure instants detection algorithms in vocal emotion conversion. International workshop soft computing applications (pp. 462–473). Springer.
Vekkot, S., & Tripathi, S. (2017). Vocal emotion conversion using WSOLA and linear prediction. In Proceedings of the speech and computer—19th international conference, SPECOM 2017 (pp. 777–787), Hatfield, UK, 12–16 September, 2017.
Verhelst, W., & Roelands, M. (1993). An overlap-add technique based on waveform similarity (WSOLA) for high quality time-scale modification of speech. In 1993 IEEE international conference on acoustics, speech, and signal processing. ICASSP-93 (Vol. 2, pp. 554–557). IEEE.
Verma, R., Sarkar, P., & Rao, K. S. (2015). Conversion of neutral speech to storytelling style speech. In Proceedings of IEEE ICAPR.
Vuppala, A., & Kadiri, S. (2014). Neutral to anger speech conversion using non-uniform duration modification. In 9th international conference on industrial and information systems (ICIIS) (pp. 1–4).
Vydana, H., Kadiri, S., & Vuppala, A. (2016). Vowel-based non-uniform prosody modification for emotion conversion. Circuits, Systems, and Signal Processing, 35(5), 1643–1663.
Vydana, H., Raju, V. V., Gangashetty, S. V., & Vuppala, A. (2015). Significance of emotionally significant regions of speech for emotive to neutral conversion. In International conference on mining intelligence and knowledge exploration (pp. 287–296). Springer.
Wu, Z., Chng, E. S., & Li, H. (2013). Conditional restricted Boltzmann machine for voice conversion. In Proceedings of the international conference on signal and information processing (ChinaSIP) (pp. 104–108). IEEE.
Wu, C., Hsia, C., Lee, C., & Lin, M. (2010). Hierarchical prosody conversion using regression-based clustering for emotional speech synthesis. IEEE Transactions on Audio, Speech, and Lang Processing, 18(6), 1394–1405.
Wu, Z., Virtanen, T., Chng, E., & Li, H. (2014). Exemplar-based sparse representation with residual compensation for voice conversion. IEEE/ACM Transactions on Audio, Speech, and Language Processing, 22(10), 1506–1521.
Yadav, J., & Rao, K. S. (2016). Prosodic mapping using neural networks for emotion conversion in Hindi language. Circuits, Systems, and Signal Processing, 35(1), 139–162.
Acknowledgements
This research is supported by Govt. of India’s Visveswaraya Ph D scheme through scholarship for first author towards completion of her Ph D.
Author information
Authors and Affiliations
Corresponding author
Additional information
Publisher's Note
Springer Nature remains neutral with regard to jurisdictional claims in published maps and institutional affiliations.
Rights and permissions
About this article
Cite this article
Vekkot, S., Gupta, D. Speaker-independent expressive voice synthesis using learning-based hybrid network model. Int J Speech Technol 23, 597–613 (2020). https://doi.org/10.1007/s10772-020-09691-1
Received:
Accepted:
Published:
Issue Date:
DOI: https://doi.org/10.1007/s10772-020-09691-1