Abstract
Recently, artificial intelligence (AI) has become a primary tool of serving science and humanity in all fields. This is due to the significant development in computing. The use of AI and machine learning (ML) has extended to wireless networks that are constantly evolving. This enables better operation and management of networks, through algorithms that learn and utilize available data and measurements to optimize network performance. This article provides a detailed review on cognitive, self-organized, and Software-defined networks. We discuss ML concepts and put emphasis on how ML can contribute to the development of optimal management solutions of wireless networks. A focus is put on discussion and analysis of recent research trends and challenges that remain open and require further research and exploration.
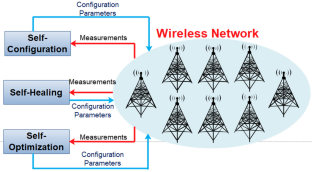





Similar content being viewed by others
Explore related subjects
Discover the latest articles, news and stories from top researchers in related subjects.References
Zhao, Y., Li, Y., Zhang, X., Geng, G., Zhang, W., Sun, Y.: A survey of networking applications applying the software defined networking concept based on machine learning. IEEE Access 7, 95397–95417 (2019)
Elsayed, M., Erol-Kantarci, M.: AI-enabled future wireless networks: challenges, opportunities, and open issues. IEEE Veh. Technol. Mag. 14(3), 70–77 (2019)
Mao, Q., Hu, F., Hao, Q.: Deep learning for intelligent wireless networks: a comprehensive survey. IEEE Commun. Surv. Tutor. 20(4), 2595–2621 (2018)
Zhang, C., Patras, P., Haddadi, H.: Deep learning in mobile and wireless networking: a survey. IEEE Commun. Surv. Tutor. 21(3), 2224–2287 (2019)
Hämäläinen, S., Sanneck, H., Sartori, C., Self-Organising, L.T.E.: Networks (SON): Network Management Automation for Operational Efficiency. Wiley, Hoboken (2011)
Gacanin, H., Ligata, A.: Wi-fi self-organizing networks: challenges and use cases. IEEE Commun. Mag. 55(7), 158–164 (2017)
Thang, V., Pashchenko, F.: Multistage system-based machine learning techniques for intrusion detection in WiFi network. J. Comput. Netw. Commun. (2019). https://doi.org/10.1155/2019/4708201
Russell, S., Norvig, P.: Artificial Intelligence (a Modern Approach), 3rd edn. Prentice Hall, Hoboken (1995)
Liu, Y., Bi, S., Shi, Z., Hanzo, L.: When machine learning meets big: a wireless communication perspective. IEEE Veh. Technol. Mag. 15, 63–72 (2020)
Hu, F., Hao, Q., Bao, K.: A survey on software-defined network and openflow: from concept to implementation. IEEE Commun. Surv. Tutor. 16, 2181–2206 (2014)
Agarwal, S., Kodialam, M., Lakshman, T.: Traffic engineering in software defined networks. In: Proc. IEEE INFOCOM, pp. 2211–2219 (2013)
Lin, P., Bi, J., Wolff, S.: A west-east bridge based SDN inter-domain testbed. IEEE Commun. Mag. 53(2), 190–197 (2015)
Xie, J., Yu, F., Huang, T., Xie, R., Liu, J., Wangz, C., Liu, Y.: A survey of machine learning techniques applied to software defined networking (SDN): research issues and challenges. IEEE Commun. Surv. Tutor. 21, 393–430 (2019)
Kosmidesa, P., Adamopouloua, E., Demestichasa, K., Anagnostoua, M., Rouskasb, A.: On Intelligent Base Station Activation for Next Generation Wireless Networks, the 6th International Conference on Emerging Ubiquitous Systems and Pervasive Networks. Elsevier, Amsterdam (2015)
Li, R., Zhao, Z., Chen, X., Zhang, H.: Energy saving through a learning framework in greener cellular radio access networks. In: Proceedings of GLOBECOM, pp. 1556–1561 (2012)
Ding, H., Zhao, F., Tian, J., Li, D., Zhang, H.: A deep reinforcement learning for user association and power control in heterogeneous net works. Ad Hoc Netw. 102, 102069 (2020)
Yu, Y., Wang, T., Liew, S.: Deep-reinforcement learning multiple access for heterogeneous wireless networks. IEEE Int. Conf. Commun. (ICC) 37, 1277–1290 (2018)
Onireti, O.: A cell outage management framework for dense heterogeneous networks. IEEE Trans. Veh. Technol. 65, 2097–2113 (2016)
Mohammadi, M., Al-Fuqaha, A.: Enabling cognitive smart cities using big data and machine learning: approaches and challenges. IEEE Commun. Mag. 56, 94–101 (2018)
He, Y.: Software-defined networks with mobile edge computing and caching for smart cities: a big data deep reinforcement learning approach. IEEE Commun. Mag. 55, 31–37 (2017)
Jia, G., Yang, Z., Lam, H., Shi, J., Shikh-Bahaei, M.: Channel assignment in uplink wireless communication using machine learning approach. IEEE Commun. Lett. 24, 787–791 (2020)
Zappone, A., Sanguinetti, L., Debbah, M.: User association and load balancing for massive MIMO through deep learning. In: Proceedings of IEEE Asilomar Conference on Signals, Systems, and Computers, pp. 1262–1266 (2018)
Lin, P.: Large-scale and high-dimensional cell outage detection in 5G self-organizing networks. In: Proceedings of APSIPA Annual Summit and Conference, pp. 8–12 (2019)
Pervez, F., Jaber, M., Qadir, J., Younis, S., Imran, M.: Fuzzy Q-learning-based user-centric backhaul-aware user cell association scheme. In: Proceedings of IWCMC, pp. 1840–1845 (2017)
Kumar, Y., Farooq, H., Imran, A.: Fault prediction and reliability analysis in a real cellular network. In: 13th International Wireless Communications and Mobile Computing Conference (IWCMC), pp. 1090–1095 (2017)
Song, Ronggong, Willink, Tricia: Machine Learning-Based Traffic Classification of Wireless Traffic, International Conference on Military Communications and Information Systems (ICMCIS), (2019)
Han, J., Kamber, M., Pei, J.: Data Mining: Concepts and Techniques, 3rd edn. Morgan Kaufmann Publisher, Burlington (2011)
Nguyen, T., Armitage, G., Branch, P., Zander, S.: Timely and continuous machine-learning-based classification for interactive IP traffic. IEEE/ACM Trans. Netw. 20, 1880–1894 (2012)
Al-Issax, A., Bentaleb, A., Barakabitzex, A., Zinnery, T., Ghita, B.: Bandwidth Prediction Schemes for Defining Bitrate Levels in SDN-enabled Adaptive Streaming, 15th International Conference on Network and Service Management (CNSM) (2019)
Testi, E., Favarelli, E., Giorgetti, A.: Machine Learning For User Traffic Classification in Wireless Systems. 26th European Signal Processing Conference (EUSIPCO) (2018)
Barki, L., Shidling, A., Meti, N., Narayan, D., Mulla, M.: Detection of distributed denial of service attacks in software defined networks. In: Proceedings of IEEE ICACCI, IEEE, pp. 2576–2581 (2016)
Fan, Z., Liu, R.: Investigation of machine learning based network traffic classification. In: Proceedings of ISWCS, pp. 1–6 (2017)
Song, C., Park, Y., Golani, K., Kim, Y., Bhatt, K., Goswami, K.: Machine-learning based threat-aware system in software defined networks. In: Proceedings of IEEE ICCCN, pp. 1–9 (2017)
Glick, M., Rastegarfar, H.: Scheduling and control in hybrid data centers. In: Proceedings IEEE PHOSST’17, pp. 115–116 (2017)
Xiao, P., Qu, W., Qi, H., Xu, Y., Li, Z.: An efficient elephant flow detection with cost-sensitive in SDN. In: Proceedings of IEEE INISCom’15, pp. 24–28, (2015)
Huang, T., Zhang, R., Zhou, C., Sun, L.: QARC: video quality aware rate control for real-time video streaming based on deep reinforcement learning. ACM Multimedia Conference, ACM (2018)
Abdelhadi Azzouni, A., Guy Pujolle, G.: Neutm: A neural network-based framework for traffic matrix prediction in SDN. In: proceedings of the IEEE/IFIP Network Operations and Management Symposium, NOMS (2018). https://doi.org/10.1109/NOMS.2018.8406199
Carner, J., Mestres, A., Alarcn, E., Cabellos, A.: Machine learning-based network modeling: An artificial neural network model vs a theoretical inspired model. In: Proceedings of IEEE ICUFN’17, pp. 522–524 (2017)
Jain, S., Khandelwal, M., Katkar, A., Nygate, J.: Applying big data technologies to manage QoS in an SDN. In: Proceedings of IEEE CNSM’16, pp. 302–306 (2016)
Pasquini, R., Stadler, R.: Learning end-to-end application QoS from OpenFlow switch statistics. In: Proceedings of IEEE NETSOFT’17, pp. 1–9 (2017)
Letaifa, A.: Adaptive QoE monitoring architecture in SDN networks: Video streaming services case. In: Proceedings of IEEE IWCMC’17, pp. 1383–1388 (2017)
Abar, T., Letaifa, A., Asmi, S.: Machine learning based QoE prediction in SDN networks. In: Proceedings of IEEE IWCMC’17, pp. 1395–1400 (2017)
Tayyaba, S., Khattak, H., Almogren, A., Shah, M., Din, I., Alkhalifa, I., Guizani, M.: 5G vehicular network resource management for improving radio access through machine learning. IEEE Access 8, 6792–6800 (2020)
Comaneci, D., Dobre, C.: Securing networks using SDN and machine learning. In: IEEE International Conference on Computational Science and Engineering (2018)
Murudkar, Chetana V., Gitlin, Richard D.: QoE-driven Anomaly Detection in Self Organizing Mobile Networks Using Machine Learning, 18th annual IEEE Wireless Telecommunications Symposium (WTS) (2019)
Murudkar, C., Gitlin, R.: Machine learning for QoE prediction and anomaly detection in self-organizing mobile networking systems. Int. J. Wirel. Mob. Netw. (IJWMN) (2019). https://doi.org/10.2139/ssrn.3383948
Lim, S.: Software defined network detection system. Int. J. Recent Technol. Eng. (IJRTE) 8, 1391–1395 (2019)
Yao, H., Mai, T., Xu, X., Zhang, P., Li, M., Liu, Y.: NetworkAI: an intelligent network architecture for self-learning control strategies in software defined networks. IEEE Internet Things J. 5, 4319–4327 (2018)
Zhu, L., Tang, X., Shen, M., Du, X., Guizani, M.: Privacy-preserving DDoS attack detection using cross-domain traffic in software defined networks. IEEE J. Sel. Areas Commun. 36, 628–643 (2018)
Côté, D.: Using machine learning in communication networks. J. Opt. Commun. Netw. 10, D100–D109 (2018)
Gazis, V., Sasloglou, K., Frangiadakis, N., Kikiras, P., Merentitis, A., Mathioudakis, K., Mazarakis, G.: Cooperative communication in channel assignment strategies for IEEE 802.11k WLAN systems. In: IEEE 24th Annual International Symposium on Personal, Indoor, and Mobile Radio Communications (PIMRC), pp. 1924–1929 (2013)
Seyedebrahimi, M., Bouhafs, F., Raschella, A., Mackay, M., Shi, Q.: Fine-grained radio resource management to control interference in dense wi-fi networks. In: IEEE Wireless Communications and Networking Conference (WCNC), pp. 1–6 (2017)
Hartog, F., Raschella, A., Bouhafs, F., Kempker, P., Boltjes, B., Seyedebrahimi, M.: A pathway to solving the wi-fi tragedy of the commons in apartment blocks. In: 27th International Telecommunication Networks and Applications Conference (ITNAC), pp. 1–6 (2017)
Moura, H., Alves, A., Borges, J., Macedo, D., Vieira, M.: Ethanol: a software-defined wireless networking architecture for IEEE 802.11 networks, computer communications, pp. 176–188. Elsevier, Amsterdam (2020)
Lei, T., Wen, X., Lu, Z., Li, Y.: A semi-matching based load balancing scheme for dense IEEE 802.11 WLANs. IEEEIEEE Access 5, 15332–15339 (2017)
Peng, M., He, G., Wang, L., Kai, C.: AP selection scheme based on achievable throughputs in SDN-enabled WLANs. IEEE Access 7, 4763–4772 (2019)
Ernst, J., Kremer, S., Rodrigues, J.: A utility based access point selection method for IEEE 802.11 wireless networks with enhanced quality of experience. In: Proceedings of IEEE ICC, pp. 2363–2368 (2014)
Chen, J., Liu, B., Zhou, H., Yu, Q., Gui, L., Shen, X.: QoS-driven efficient client association in high-density software-defined WLAN. IEEE Trans. Veh. Technol. 66, 7372–7383 (2017)
Bojovic, B., Baldo, N., Nin-Guerrero, J., Dini, P.: A supervised learning approach to cognitive access point selection. In: GLOBECOM Workshops. IEEE, Piscataway (2011)
Wilhelmi, F., Barrachina-Muñnoz, S., Bellalta, B., Cano, C., Jonsson, A., Ram, V.: A flexible machine learning-aware architecture for future WLANs. IEEE Commun. Mag. 58, 25–31 (2020)
Testolin, A., Zanforlin, M., De Grazia,M., Munaretto, D., Zanella, A., Zorzi, M.: A machine learning approach to qoe-based video admission control and resource allocation in wireless systems. In: Ad Hoc Networking Workshop (MED-HOC-NET), IEEE, pp. 31–38 (2014)
Vassis, D., Kampouraki, A., Belsis, P., Skourlas, C.: Admission control of video sessions over ad hoc networks using neural classifiers. In: IEEE Military Communications Conference, IEEE, pp. 15–20 (2014)
Quer, G., Baldo, N., Zorzi, M.: Cognitive call admission control for voip over ieee 802.11 using bayesian networks. In: Proceedings of GLOBECOM, IEEE, pp. 1–6 (2011)
Coronado, E., Villalon, J., Garrido, A.: Wi-balance: SDN-based load-balancing in enterprise WLANs. In: IEEE Conference on Network Softwarization (NetSoft), pp. 1–2 (2017)
Jagannath, J., Polosky, N., Jagannath, A., Restuccia, F., Melodia, T.: Machine learning for wireless communications in the internet of things: a comprehensive survey. Ad Hoc Netw. 93, 101913 (2019)
Schmidt, M., Block, D., Meier, U.: Wireless interference identification with convolutional neural networks. In: 15th International Conference on Industrial Informatics (INDIN), IEEE (2017)
Sanguanpuak, T., Guruacharya, S., Rajatheva, N., Bennis, M., Latva-Aho, M.: Multi-operator spectrum sharing for small cell networks: a matching game perspective. IEEE Trans. Wirel. Commun. 16, 3761–3774 (2017)
Grimaldi, S., Mahmood, A., Gidlund, M.: An SVM-based method for classification of external interference in industrial wireless sensor and actuator networks. J. Sens. Actuator Netw. 6, 9 (2017)
Kulin, M., Kazaz, T., Moerman, I., Poorter, E.: End-to-end learning from spectrum data: a deep learning approach for wireless signal identification in spectrum monitoring applications. IEEE Access 6, 18484–18501 (2018)
Davaslioglu, K., Soltani, S., Erpek, T., Sagduyu, Y.: DeepWiFi: cognitive WiFi with deep learning. IEEE Trans. Mob. Comput. 20, 429–444 (2019)
Jeunen, O., Bosch, P., Herwegen, M., Doorselaer, K., Godman, N., Latre, S.: A machine learning approach for ieee 802.11 channel allocation. In: 14th International Conference on Network and Service Management (CNSM), pp. 28–36 (2018)
Lim, T., Jeon, W., Jeong, D.: Centralized channel allocation scheme in densely deployed 802.11 wireless lans. In: 18th International Conference on Advanced Communication Technology (ICACT), pp. 249–253 (2016)
Baid, A., Raychaudhuri, D.D.: Understanding channel selection dynamics in dense Wi-Fi networks. IEEE Commun. Mag. 53, 110–117 (2015)
Moura, H., Macedo, D., Vieira, M.: Automatic quality of experience management for wlan networks using multi-armed bandit. In: IFIP/IEEE Symposium on Integrated Network and Service Management (IM), pp. 279–288 (2019)
Singh, S.: SDN (Software Defined Network) and Machine Learning for High-Density WLANs. In: Proceedings of National Conference on Machine Learning, pp. 82–91 (2019)
Herzen, J., Lundgren, H., Hegde, N.: Learning Wi-Fi Performance, 12th Annual International Conference on Sensing, Communication, and Networking (SECON), IEEE (2015)
Boutaba, R., Salahuddin, M., Limam, N., Ayoubi, S., Shahriar, N., Estrada-Solano, F., Caicedo, O.: A comprehensive survey on machine learning for networking: evolution, applications and research opportunities. J. Internet Serv. Appl. 9, 1–99 (2018)
Han, K., Lee, J., Kim, B.: Machine-Learning based Loss Discrimination Algorithm for Wireless TCP Congestion Control. International Conference on Electronics, Information, and Communication (ICEIC) (2019)
Moriyama, Tomokazu, Yamamoto, Ryo, Ohzahata, Satoshi, Kato, Toshihiko: TCP Congestion Control over IEEE 802.11 Wireless Lans based on K-Means Clustering Focusing on Congestion Window Size and Round-trip Time. International Conference on Data Communication Networking (2018)
Sui, K., Zhou, M., Liu, D., Ma, M., Pei, D., Zhao, Y., Li, Z., Moscibroda, T.: Characterizing and Improving WiFi Latency in Large-Scale Operational Networks, The 14th ACM International Conference on Mobile Systems, Applications, and Services, ACM (2016)
Coronado, E., Thomas, A., Riggio, R.: Adaptive ML-based frame length optimization in enterprise SD-WLANs. J. Netw. Syst. Manage. (2020). https://doi.org/10.1007/s10922-020-09527-y
Ibarrola, E., Davis, M., Voisin, C., Close, C., Cristobo, L.: QoE enhancement in next generation wireless ecosystems: a machine learning approach. IEEE Commun. Stand. Mag. 3, 63–70 (2019)
Košťál, K., Bencel, R., Ries, M., Trúchly, P., Kotuliak, I.: High performance SDN WLAN architecture, Sensors. In: Proceedings of PMC (2019)
Wang, Z., Xu, Y., Li, L., Tian, H., Cui, S.: Handover control in wireless systems via asynchronous multi-user deep reinforcement learning. IEEE Internet Things J. 5, 4296–4307 (2018)
Zhou, P., Chang, Y., Copeland, J.: Determination of wireless networks parameters through parallel hierarchical support vector machines. IEEE Trans. Parallel Distrib. Syst. 23, 505–512 (2012)
Yu, C., Chen, K., Cheng, S.: Cognitive radio network tomography. IEEE Trans. Veh. Technol. 59, 1980–1997 (2010)
Xia, M.: Optical and wireless hybrid access networks: design and optimization. OSA/IEEE J. Opt. Commun. Netw. 4, 749–759 (2012)
Sequeira, L., Cruz, J., Ruiz-Mas, J., Saldana, J., Fernandez-Navajas, J., Almodovar, J.: Building an SDN enterprise WLAN based on virtual APs. IEEE Commun. Lett. 21, 374–377 (2017)
Author information
Authors and Affiliations
Corresponding author
Additional information
Publisher's Note
Springer Nature remains neutral with regard to jurisdictional claims in published maps and institutional affiliations.
Rights and permissions
About this article
Cite this article
Abusubaih, M. Intelligent Wireless Networks: Challenges and Future Research Topics. J Netw Syst Manage 30, 18 (2022). https://doi.org/10.1007/s10922-021-09625-5
Received:
Revised:
Accepted:
Published:
DOI: https://doi.org/10.1007/s10922-021-09625-5