Abstract
Analysis of audio from real-life environments and their categorization into different acoustic scenes can make context-aware devices and applications more efficient. Unlike speech, such signals have overlapping frequency content while spanning a much larger audible frequency range. Also, they are less structured than speech/music signals. Wavelet transform has good time-frequency localization ability owing to its variable-length basis functions. Consequently, it facilitates the extraction of more characteristic information from environmental audio. This paper attempts to classify acoustic scenes by a novel use of wavelet-based mel-scaled features. The design of the proposed framework is based on the experiments conducted on two datasets which have same scene classes but differ with regard to sample length and amount of data (in hours). It outperformed two benchmark systems, one based on mel-frequency cepstral coefficients and Gaussian mixture models and the other based on log mel-band energies and multi-layer perceptron. We also present an investigation on the use of different train and test sample duration for acoustic scene classification.








Similar content being viewed by others
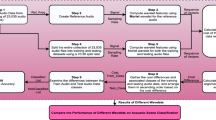
References
Aucouturier JJ, Défréville B, Pachet F (2007) The bag-of-frames approach to audio pattern recognition: a sufficient model for urban soundscapes but not for polyphonic music. J Acoustic Soc Amer 122(2):881–891
Barchiesi D, Giannoulis D, Stowell D, Plumbley MD (2015) Acoustic scene classification: classifying environments from the sounds they produce. IEEE Signal Proc Mag 32(3):16–34
Bisot V, Serizel R, Essid S, Richard G (2017) Feature learning with matrix factorization applied to acoustic scene classification. IEEE/ACM Trans Audio, Speech, Language Process 25(6):1216–1229
Brown GJ, Cooke M (1994) Computational auditory scene analysis. Comput Speech Language 8(4):297–336
Brummer N (2007) FoCal multi-class: toolkit for evaluation, fusion and calibration of multi-class recognition scores. Tutorial and user manual. Software available at https://sites.google.com/site/nikobrummer/focal
Chu S, Narayanan S, Kuo CCJ (2009) Environmental sound recognition with time-frequency audio features. IEEE Trans Audio, Speech, and Language Process 17 (6):1142–1158
Daubechies I (1992) Ten lectures on wavelets, 61. Siam
Davis SB, Mermelstein P (1990) Comparison of parametric representations for monosyllabic word recognition in continuously spoken sentences. In: Readings in speech recognition. Elsevier, 65–74
Dubois D, Guastavino C, Raimbault M (2006) A cognitive approach to urban soundscapes: Using verbal data to access everyday life auditory categories. Acta Acustic United Acustica 92(6):865–874
Eghbal-Zadeh H, Lehner B, Dorfer M, Widmer G (2016) CP-JKU Submissions for DCASE-2016: a hybrid approach using binaural i-vectors and deep convolutional neural networks. In: IEEE AASP Challenge on detection and classification of acoustic scenes and events (DCASE 2016), Budapest, Hungary, Tech. Rep
Gabor D (1946) Theory of communication. part 1: the analysis of information. J Institut Electr Engineers-Part III: Radio Commun Eng 93(26):429–441
Ghodasara V, Naser DS, Waldekar S, Saha G (2015) Speech/music classification using block based MFCC features. Music Information Retrieval Evaluation eXchange (MIREX)
Ghodasara V, Waldekar S, Paul D, Saha G (2016) Acoustic scene classification using block-based MFCC features. In: IEEE AASP Challenge on detection and classification of acoustic scenes and events (DCASE 2016), Budapest, Hungary, Tech. Rep
Giannoulis D, Benetos E, Stowell D, Rossignol M, Lagrange M, Plumbley MD (2013) Detection and classification of acoustic scenes and events: an IEEE AASP challenge. In: 2013 IEEE Workshop on Applications of Signal Processing to Audio and Acoustics (WASPAA), pp 1–4
Gowdy JN, Tufekci Z (2000) Mel-scaled discrete wavelet coefficients for speech recognition. In: Proceedings of the 2000 IEEE international conference on Acoustics, speech, and signal processing. ICASSP’00, vol 3, pp 1351–1354
Kim K, Youn DH, Lee C (2000) Evaluation of wavelet filters for speech recognition. In: 2000 IEEE international conference on Systems, man, and cybernetics, vol 4, pp 2891–2894
Lagrange M, Lafay G, Défréville B, Aucouturier JJ (2015) The bag-of-frames approach: A not so sufficient model for urban soundscapes. J Acoustic Soc Amer 138(5):EL487–EL492
Li Y, Zhang X, Jin H, Li X, Wang Q, He Q, Huang Q (2018) Using multi-stream hierarchical deep neural network to extract deep audio feature for acoustic event detection. Multimed Tool Appl 77(1):897–916
Lyon RF (2010) Machine hearing: an emerging field. IEEE Signal Proc Mag 27 (5):131–139
Ma J, Wang R, Ji W, Zheng H, Zhu E, Yin J (2019) Relational recurrent neural networks for polyphonic sound event detection. Multimedia Tools and Applications 1–19
Mallat SG (1989) A theory for multiresolution signal decomposition: the wavelet representation. IEEE Trans Pattern Anal Mach Intell 11(7):674–693
Mesaros A, Heittola T, Eronen A, Virtanen T (2010) Acoustic event detection in real life recordings. In: IEEE 2010 18th European on Signal Processing Conference, pp 1267–1271
Mesaros A, Heittola T, Virtanen T (2016) TUT database for acoustic scene classification and sound event detection. In: IEEE 2016 24th European on Signal Processing Conference (EUSIPCO), pp 1128–1132
Mesaros A, Heittola T, Benetos E, Foster P, Lagrange M, Virtanen T, Plumbley M (2017) Detection and classification of acoustic scenes and events: outcome of the DCASE 2016 challenge. IEEE/ACM Transactions on Audio, Speech and Language Processing
Mesaros A, Heittola T, Diment A, Elizalde B, Shah A, Vincent E, Raj B, Virtanen T (2017) DCASE 2017 challenge setup: tasks, datasets and baseline system. In: DCASE 2017-Workshop on Detection and Classification of Acoustic Scenes and Events
Mesaros A, Heittola T, Virtanen T (2018) Acoustic scene classification: an overview of DCASE 2017 challenge entries. In: 16th International Workshop on Acoustic Signal Enhancement (IWAENC)
Mun S, Park S, Han D, Ko H (2017) Generative adversarial network based acoustic scene training set augmentation and selection using SVM hyper-plane. Tech. rep., Tech. Rep., DCASE2017 Challenge
Phan H, Chén OY, Koch P, Pham L, McLoughlin I, Mertins A, De Vos M (2018) Beyond equal-length snippets: How long is sufficient to recognize an audio scene?. arXiv:181101095
Rabaoui A, Davy M, Rossignol S, Ellouze N (2008) Using one-class SVMs and wavelets for audio surveillance. IEEE Trans Inform Forensics Secur 3(4):763–775
Rakotomamonjy A, Gasso G (2015) Histogram of gradients of time-frequency representations for audio scene classification. IEEE/ACM Transactions on Audio Speech and Language Processing (TASLP) 23(1):142–153
Sahidullah M, Saha G (2012) Design, analysis and experimental evaluation of block based transformation in MFCC computation for speaker recognition. Speech Comm 54(4):543–565
Stowell D, Giannoulis D, Benetos E, Lagrange M, Plumbley MD (2015) Detection and classification of acoustic scenes and events. IEEE Trans Multimed 17 (10):1733–1746
Tang G, Liang R, Xie Y, Bao Y, Wang S (2018) Improved convolutional neural networks for acoustic event classification. Multimedia Tools and Applications 1–16
Tufekci Z, Gowdy J (2000) Feature extraction using discrete wavelet transform for speech recognition. In: 2000 Proceedings of the IEEE on Southeastcon, pp 116–123
Tzanetakis G, Essl G, Cook P (2001) Audio analysis using the discrete wavelet transform. In: Proc. Conf. in Acoustics and Music Theory Applications, vol 66
Waldekar S, Saha G (2018) Classification of audio scenes with novel features in a fused system framework. Digital Signal Processing
Waldekar S, Saha G (2018) Wavelet transform based mel-scaled features for acoustic scene classification. Proc Interspeech 2018:3323–3327
Weston J, Watkins C et al (1999) Support vector machines for multi-class pattern recognition. In: Esann, vol 99, pp 219–224
Author information
Authors and Affiliations
Corresponding author
Additional information
Publisher’s note
Springer Nature remains neutral with regard to jurisdictional claims in published maps and institutional affiliations.
Rights and permissions
About this article
Cite this article
Waldekar, S., Saha, G. Analysis and classification of acoustic scenes with wavelet transform-based mel-scaled features. Multimed Tools Appl 79, 7911–7926 (2020). https://doi.org/10.1007/s11042-019-08279-5
Received:
Revised:
Accepted:
Published:
Issue Date:
DOI: https://doi.org/10.1007/s11042-019-08279-5