Abstract
Image segmentation is an important part of image processing, which directly affects the quality of image processing results. Threshold segmentation is the simplest and most widely used segmentation method. However, the best method to determine the threshold has always been a NP-hard problem. Therefore, this paper proposes Kapur’s entropy image segmentation based on multi-strategy manta ray foraging optimization, which has a good effect in CEC 2017 test function and image segmentation. Manta ray foraging optimization (MRFO) is a new intelligent optimization algorithm, which has good searchability, but the local development ability is insufficient, so it can not effectively find a reliable point. To solve this defect, this paper proposes a multi-strategy learning manta ray foraging optimization algorithm, referred to as MSMRFO, which uses saltation learning to speed up the communication within the population and improve the convergence speed, and then puts forward a behavior selection strategy to judge the current situation of the population, Tent disturbance and Gaussian mutation are used to avoid the algorithm falling into local optimization and improve the convergence speed of the algorithm. In the complete CEC 2017 test set, MSMRFO is compared with 8 algorithms, including FA_CL and ASBSO are variants of new algorithms proposed in recent years. The results show that MSMRFO has good optimization ability and universality. In nine underwater image data sets, MSMRFO has better segmentation quality than the other eight algorithms, and the segmentation indicators under high threshold processing has better advantages.






Similar content being viewed by others
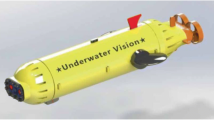
Data availability
The data used to support the findings of this study are available from the corresponding author upon request.
References
Abd El Aziz M, Ewees AA, Hassanien AE (2017) Whale optimization algorithm and moth-flame optimization for multilevel thresholding image segmentation. Expert Syst Appl 83:242–256. https://doi.org/10.1016/j.eswa.2017.04.023
Abd Elaziz M, Yousri D, Al-qaness MA, AbdelAty AM, Radwan AG, Ewees AA (2021) A Grunwald–Letnikov based Manta ray foraging optimizer for global optimization and image segmentation. Eng Appl Artif Intell 98:104105. https://doi.org/10.1016/j.engappai.2020.104105
Akay B (2013) A study on particle swarm optimization and artificial bee colony algorithms for multilevel thresholding. Appl Soft Comput 13(6):3066–3091. https://doi.org/10.5555/2467341.2467507
Akdag O, Yeroglu C (2021) Optimal directional overcurrent relay coordination using MRFO algorithm: A case study of adaptive protection of the distribution network of the Hatay province of Turkey. Electr Power Syst Res 192:106998. https://doi.org/10.1016/j.epsr.2020.106998
Alturki FA, Farh MH, Al-Shamma’a AA, AlSharabi K (2020) Techno-economic optimization of small-scale hybrid energy systems using manta ray foraging optimizer. Electronics 9(12):2045. https://doi.org/10.3390/electronics9122045
Aly M, Rezk H (2021) A MPPT based on optimized FLC using manta ray foraging optimization algorithm for thermo-electric generation systems. Int J Energy Res 45(9):13897–13910. https://doi.org/10.1002/er.6728
Bao X, Jia H, Lang C (2019) A novel hybrid Harris hawks optimization for color image multilevel thresholding segmentation. IEEE Access 7:76529–76546. https://doi.org/10.1109/ACCESS.2019.2921545
Ben UC, Akpan AE, Mbonu CC, Ebong ED (2021) Novel methodology for interpretation of magnetic anomalies due to two-dimensional dipping dikes using the Manta ray foraging optimization. J Appl Geophys 192:104405. https://doi.org/10.1016/j.jappgeo.2021.104405
Das S, Suganthan PN (2010) Differential evolution: A survey of the state-of-the-art. IEEE Trans Evol Comput 15(1):4–31. https://doi.org/10.1109/TEVC.2010.2059031
Demšar J (2006) Statistical comparisons of classifiers over multiple data sets. J Mach Learn Res 7:1–30. https://doi.org/10.5555/1248547.1248548
Ekinci S, Izci D, Hekimoğlu B (2021) Optimal FOPID speed control of DC motor via opposition-based hybrid manta ray foraging optimization and simulated annealing algorithm. Arab J Sci Eng 46(2):1395–1409. https://doi.org/10.1007/s13369-020-05050-z
Elmaadawy K, Abd Elaziz M, Elsheikh AH, Moawad A, Liu B, Lu S (2021) Utilization of random vector functional link integrated with manta ray foraging optimization for effluent prediction of wastewater treatment plant. J Environ Manag 298:113520. https://doi.org/10.1016/j.jenvman.2021.113520
Erdmann H, Wachs-Lopes G, Gallao C, Ribeiro MP, Rodrigues PS (2015) A study of a firefly meta-heuristics for multithreshold image segmentation. In Developments in medical image processing and computational vision. Springer, Cham. pp. 279–295 https://doi.org/10.1007/978-3-319-13407-9_17
Fathy A, Rezk H, Yousri D (2020) A robust global MPPT to mitigate partial shading of triple-junction solar cell-based system using manta ray foraging optimization algorithm[J]. Sol Energy 207:305–316. https://doi.org/10.1016/j.solener.2020.06.108
Fayad H, Hatt M, Visvikis D (2015) PET functional volume delineation using an Ant colony segmentation approach. (2015):1745–1745. https://jnm.snmjournals.org/content/56/supplement_3/1745.short
Fogel DB, Atmar JW (1990) Comparing genetic operators with Gaussian mutations in simulated evolutionary processes using linear systems. Biol Cybern 63(2):111–114. https://doi.org/10.1007/BF00203032
Ghosh KK, Guha R, Bera SK, Kumar N, Sarkar R (2021) S-shaped versus V-shaped transfer functions for binary Manta ray foraging optimization in feature selection problem. Neural Comput & Applic 33(17):11027–11041. https://doi.org/10.1007/s00521-020-05560-9
Haralick RM, Shapiro LG (1985) Image segmentation techniques. Comput Vis Graph Image Process 29(1):100–132. https://doi.org/10.1016/S0734-189X(85)90153-7
Hassan MH, Houssein EH, Mahdy MA, Kamel S (2021) An improved manta ray foraging optimizer for cost-effective emission dispatch problems. Eng Appl Artif Intell 100:104155. https://doi.org/10.1016/j.engappai.2021.104155
Hemeida MG, Alkhalaf S, Mohamed AAA, Ibrahim AA, Senjyu T (2020) Distributed generators optimization based on multi-objective functions using manta rays foraging optimization algorithm (MRFO). Energies 13(15):3847. https://doi.org/10.3390/en13153847
Hemeida MG, Ibrahim AA, Mohamed AAA, Alkhalaf S, El-Dine AMB (2021) Optimal allocation of distributed generators DG based Manta ray foraging optimization algorithm (MRFO). Ain Shams Eng J 12(1):609–619. https://doi.org/10.1016/j.asej.2020.07.009
Higashi N, Iba H (2003, April) Particle swarm optimization with Gaussian mutation. In: Proceedings of the 2003 IEEE swarm intelligence symposium. SIS'03 (cat. No. 03EX706). IEEE. pp. 72-79 https://doi.org/10.1109/SIS.2003.1202250
Houssein EH, Zaki GN, Diab AAZ, Younis EM (2021) An efficient Manta ray foraging optimization algorithm for parameter extraction of three-diode photovoltaic model. Comput Electr Eng 94:107304. https://doi.org/10.1016/j.compeleceng.2021.107304
Houssein EH, Ibrahim IE, Neggaz N, Hassaballah M, Wazery YM (2021) An efficient ECG arrhythmia classification method based on Manta ray foraging optimization. Expert Syst Appl 181:115131. https://doi.org/10.1016/j.eswa.2021.115131
Houssein EH, Emam MM, Ali AA (2021) Improved manta ray foraging optimization for multi-level thresholding using COVID-19 CT images. Neural Comput & Applic 33(24):16899–16919. https://doi.org/10.1007/s00521-021-06273-3
Islam MJ, Luo P, Sattar J (2020) Simultaneous enhancement and super-resolution of underwater imagery for improved visual perception. arXiv preprint arXiv:2002.01155. https://doi.org/10.48550/arXiv.2002.01155
Jena B, Naik MK, Panda R, Abraham A (2021) Maximum 3D Tsallis entropy based multilevel thresholding of brain MR image using attacking Manta ray foraging optimization. Eng Appl Artif Intell 103:104293. https://doi.org/10.1016/j.engappai.2021.104293
Jia H, Lang C, Oliva D, Song W, Peng X (2019) Dynamic Harris hawks optimization with mutation mechanism for satellite image segmentation. Remote Sens 11(12):1421. https://doi.org/10.3390/rs11121421
Jin H, Li Y, Xing B, Wang L (2016) A geometric image segmentation method based on a bi-convex, fuzzy, variational principle with teaching-learning optimization. J Intell Fuzzy Syst 31(6):3075–3081. https://doi.org/10.3233/JIFS-169193
Karaboga D, Basturk B (2008) On the performance of artificial bee colony (ABC) algorithm. Appl Soft Comput 8(1):687–697. https://doi.org/10.1016/j.asoc.2007.05.007
Kennedy J, Eberhart RC (1997, October) A discrete binary version of the particle swarm algorithm. In: 1997 IEEE international conference on systems, man, and cybernetics. Computational cybernetics and simulation. IEEE. (Vol. 5, pp. 4104-4108) https://doi.org/10.1109/ICSMC.1997.637339
Meng XB, Gao XZ, Lu L, Liu Y, Zhang H (2016) A new bio-inspired optimisation algorithm: bird swarm algorithm. J Exp Theor Artif Intell 28(4):673–687. https://doi.org/10.1080/0952813X.2015.1042530
Micev M, Ćalasan M, Ali ZM, Hasanien HM, Aleem SHA (2021) Optimal design of automatic voltage regulation controller using hybrid simulated annealing–Manta ray foraging optimization algorithm. Ain Shams Eng J 12(1):641–657. https://doi.org/10.1016/j.asej.2020.07.010
Mirjalili S, Lewis A (2016) The whale optimization algorithm. Adv Eng Softw 95:51–67. https://doi.org/10.1016/j.advengsoft.2016.01.008
Mirjalili S, Mirjalili SM, Lewis A (2014) Grey wolf optimizer. Adv Eng Softw 69:46–61. https://doi.org/10.1016/j.advengsoft.2013.12.007
Pare S, Kumar A, Bajaj V, Singh GK (2016) A multilevel color image segmentation technique based on cuckoo search algorithm and energy curve. Appl Soft Comput 47:76–102. https://doi.org/10.1016/j.asoc.2016.05.040
Peng H, Zhu W, Deng C, Wu Z (2021) Enhancing firefly algorithm with courtship learning. Inf Sci 543:18–42. https://doi.org/10.1016/j.ins.2020.05.111
Peng H, Zeng Z, Deng C, Wu Z (2021) Multi-strategy serial cuckoo search algorithm for global optimization. Knowl-Based Syst 214:106729. https://doi.org/10.1016/j.knosys.2020.106729
Rao RV, Savsani VJ, Vakharia DP (2011) Teaching–learning-based optimization: a novel method for constrained mechanical design optimization problems. Comput Aided Des 43(3):303–315. https://doi.org/10.1016/j.cad.2010.12.015
Rashedi E, Nezamabadi-Pour H, Saryazdi S (2009) GSA: a gravitational search algorithm. Inf Sci 179(13):2232–2248. https://doi.org/10.1016/j.ins.2009.03.004
Rather SA, Bala PS (2021) Constriction coefficient based particle swarm optimization and gravitational search algorithm for multilevel image thresholding. Expert Syst 38(7):e12717. https://doi.org/10.1111/exsy.12717
Saleh S, Kalyankar NV, Khamitkar SD (2010) Image segmentation by using threshold techniques. J Comput 2:2151–9617. https://arxiv.53yu.com/abs/1005.4020
Salgotra R, Singh U (2019) The naked mole-rat algorithm. Neural Comput & Applic 31(12):8837–8857. https://doi.org/10.1007/s00521-019-04464-7
Sayed GI, Solyman M, Hassanien AE (2019) A novel chaotic optimal foraging algorithm for unconstrained and constrained problems and its application in white blood cell segmentation. Neural Comput & Applic 31(11):7633–7664. https://doi.org/10.1007/s00521-018-3597-8
Shaheen AM, Ginidi AR, El-Sehiemy RA, Ghoneim SS (2020) Economic power and heat dispatch in cogeneration energy systems using manta ray foraging optimizer. IEEE Access 8:208281–208295. https://doi.org/10.1109/ACCESS.2020.3038740
Upadhyay P, Chhabra JK (2020) Kapur’s entropy based optimal multilevel image segmentation using crow search algorithm. Appl Soft Comput 97:105522. https://doi.org/10.1016/j.asoc.2019.105522
Wang S, Jia H, Peng X (2020) Modified salp swarm algorithm based multilevel thresholding for color image segmentation. Math Biosci Eng 17(1):700–724. https://doi.org/10.3934/mbe.2020036
Whitley D (1994) Genetic algorithm tutorial. Stat Comput 4(2):65–85. https://doi.org/10.1007/BF00175354
Xin Lv, Xiaodong M, Jun Z (2021) Multi-threshold image segmentation based on improved sparrow search algorithm. Syst Eng Electron Technol 43(2):10. https://doi.org/10.12305/j.issn.1001-506X.2021.02.05
Xin LV, Xiaodong M, Jun Z, Zhen W (2021) Chaotic sparrow search optimization algorithm. J Beijing Univ Aeronaut Astronaut 47(8):1712–1720. https://doi.org/10.13700/j.bh.1001-5965.2020.0298
Xu H, Song H, Xu C, Wu X, Yousefi N (2020) Exergy analysis and optimization of a HT-PEMFC using developed Manta ray foraging optimization algorithm. Int J Hydrog Energy 45(55):30932–30941. https://doi.org/10.1016/j.ijhydene.2020.08.053
Xue J, Shen B (2020) A novel swarm intelligence optimization approach: sparrow search algorithm. Syst Sci Control Eng 8(1):22–34. https://doi.org/10.1080/21642583.2019.1708830
Yang XS, Deb S (2009, December) Cuckoo search via Lévy flights. In: 2009 world congress on nature & biologically inspired computing (NaBIC). IEEE. pp. 210-214 https://doi.org/10.1109/NABIC.2009.5393690
Yang Z, Wu A (2020) A non-revisiting quantum-behaved particle swarm optimization based multilevel thresholding for image segmentation. Neural Comput & Applic 32(16):12011–12031. https://doi.org/10.1007/s00521-019-04210-z
Yu Y, Gao S, Wang Y, Cheng J, Todo Y (2018) ASBSO: an improved brain storm optimization with flexible search length and memory-based selection. IEEE Access 6:36977–36994. https://doi.org/10.1109/ACCESS.2018.2852640
Zhao D, Liu L, Yu F, Heidari AA, Chen H (2020) Ant colony optimization with horizontal and vertical crossover search: fundamental visions for multi-threshold image segmentation. Expert Syst Appl, http://aliasgharheidari.com:114122. https://doi.org/10.1016/j.eswa.2020.114122
Zhao W, Zhang Z, Wang L (2020) Manta ray foraging optimization: an effective bio-inspired optimizer for engineering applications. Eng Appl Artif Intell 87:103300. https://doi.org/10.1016/j.engappai.2019.103300
Zhou Y, Yang X, Ling Y, Zhang J (2018) Meta-heuristic moth swarm algorithm for multilevel thresholding image segmentation. Multimed Tools Appl 77(18):23699–23727. https://doi.org/10.1007/s11042-018-5637-x
Acknowledgements
This work is supported by the National Natural Science Foundation of China (Nos.62002046,62006106),The Project Supported by Zhejiang Provincial Natural Science Foundation of China(No.LQ21F020005), Basic public welfare research program of Zhejiang Province(No.LGG18E050011).
Author information
Authors and Affiliations
Corresponding authors
Ethics declarations
Conflict of interest
The authors declare that they have no conflicts of interest.
Additional information
Publisher’s note
Springer Nature remains neutral with regard to jurisdictional claims in published maps and institutional affiliations.
Appendix
Appendix
Rights and permissions
Springer Nature or its licensor holds exclusive rights to this article under a publishing agreement with the author(s) or other rightsholder(s); author self-archiving of the accepted manuscript version of this article is solely governed by the terms of such publishing agreement and applicable law.
About this article
Cite this article
Zhu, D., Zhou, C., Qiu, Y. et al. Kapur’s entropy underwater image segmentation based on multi-strategy Manta ray foraging optimization. Multimed Tools Appl 82, 21825–21863 (2023). https://doi.org/10.1007/s11042-022-14024-2
Received:
Revised:
Accepted:
Published:
Issue Date:
DOI: https://doi.org/10.1007/s11042-022-14024-2