Abstract
Hand, foot and mouth disease (HFMD) is a susceptible viral infectious disease to infants and children, which led to millions of cases and hundreds of deaths annually in China. Existing predictive methods commonly learn the development patterns from historical observations. However, almost all these methods are neglect the immediate impact of exogenous factors on HFMD transmission. To solve the limitation, we consider the approximately unidirectional influences from temperature to confirmed cases and then propose a transfer one-step-ahead learning (Tr-OSH) method to establish their association. The Tr-OSH method first extract the unidirectional representation from temperature observations, and subsequently transfer the obtained representation for HFMD cases prediction. Moreover, we notice the independent correlation of each time step and period, and generate the independent representation by the relevance to upcoming values. Intensive experiments on real-world HFMD datasets demonstrate that our Tr-OSH method much efficaciously improves prediction accuracy.






Similar content being viewed by others
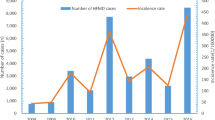
Explore related subjects
Discover the latest articles, news and stories from top researchers in related subjects.Data Availability
The data used to support the findings of this study are available from the corresponding author upon request.
References
Alabdulrazzaq H, Alenezi MN, Rawajfih Y, Alghannam BA, Al-Hassan AA, Al-Anzi FS (2021) On the accuracy of arima based prediction of covid-19 spread. Results Phys 27:104509. https://doi.org/10.1016/j.rinp.2021.104509
Alfred R, Obit JH (2021) The roles of machine learning methods in limiting the spread of deadly diseases: a systematic review. Heliyon. https://doi.org/10.1016/j.heliyon.2021.e07371
Baireddy S, Desai SR, Mathieson JL, Foster RH, Chan MW, Comer ML, Delp EJ (2021) Spacecraft time-series anomaly detection using transfer learning. In: Proceedings of the IEEE conference on computer vision and pattern recognition workshops, IEEE, virtual, pp 1951–1960. https://doi.org/10.1109/CVPRW53098.2021.00223
Cho K, van Merrienboer B, Bahdanau D, Bengio Y (2014) On the properties of neural machine translation: encoder–decoder approaches. In: Proceedings of the 8th workshop on syntax, semantics and structure in statistical translation. Association for Computational Linguistics, Doha, pp 103–111. https://doi.org/10.3115/v1/W14-4012
Gao Q, Liu Z, Xiang J, Tong M, Zhang Y, Wang S, Zhang Y, Lu L, Jiang B, Bi P (2021) Forecast and early warning of hand, foot, and mouth disease based on meteorological factors: evidence from a multicity study of 11 meteorological geographical divisions in mainland china. Environ Res 192:11031. https://doi.org/10.1016/j.envres.2020.110301
Goodfellow I, Bengio Y, Courville A (2016) Deep Learning. MIT Press. http://www.deeplearningbook.org
Gupta P, Malhotra P, Narwariya J, Vig L, Shroff G (2020) Transfer learning for clinical time series analysis using deep neural networks. J Healthc Inf Res 4(2):112–137. https://doi.org/10.1007/s41666-019-00062-3
Hamilton JD (1994) Time series analysis. Princeton University Press, Princeton
Harutyunyan H, Khachatrian H, Kale DC, Steeg GV, Galstyan A (2019) Multitask learning and benchmarking with clinical time series data. Sci Data 6(1):1–18. https://doi.org/10.1038/s41597-019-0103-9
Hochreiter S, Schmidhuber J (1997) Long short-term memory. Neural Comput 9(8):1735–1780
Koh WM, Badaruddin H, La H, Chen MIC, Cook AR (2018) Severity and burden of hand, foot and mouth disease in Asia: a modelling study. BMJ Glob Health 3(1):e000442. https://doi.org/10.1136/bmjgh-2017-000442
Li H, Lao Q (2017) The pulmonary complications associated with ev71-infected hand-foot-mouth disease. Radiol Infect Dis 4(4):137–142. https://doi.org/10.1016/j.jrid.2017.01.001
Lim B, Zohren S (2021) Time-series forecasting with deep learning: a survey. Philos Trans R Soc A Math Phys Eng Sci 379(2194):20200209. https://doi.org/10.1098/rsta.2020.0209
Liu Y, Feng G, Tsui KL, Sun S (2021) Forecasting influenza epidemics in hong kong using google search queries data: A new integrated approach. Expert Syst Appl 185:115604. https://doi.org/10.1016/j.eswa.2021.115604
Ma J, Cheng JC, Ding Y, Lin C, Jiang F, Wang M, Zhai C (2020) Transfer learning for long-interval consecutive missing values imputation without external features in air pollution time series. Adv Eng Inform 44:101092. https://doi.org/10.1016/j.aei.2020.101092
Mills TC (2019) Chapter 4-arima models for nonstationary time series. In: Applied time series analysis. Academic Press, pp 57–69. https://doi.org/10.1016/B978-0-12-813117-6.00004-1
Miotto R, Li L, Kidd BA, Dudley JT (2016) Deep patient: an unsupervised representation to predict the future of patients from the electronic health records. Sci Rep 6(1):1–10. https://doi.org/10.1038/srep26094
Pearson D, Basu R, Wu XM, Ebisu K (2020) Temperature and hand, foot and mouth disease in California: an exploratory analysis of emergency department visits by season, 2005–2013. Environ Res 185:109461. https://doi.org/10.1016/j.envres.2020.109461
Perrusquia A, Yu W (2021) Identification and optimal control of nonlinear systems using recurrent neural networks and reinforcement learning: an overview. Neurocomput In Press. https://doi.org/10.1016/j.neucom.2021.01.096
Qi H, Li Y, Zhang J, Chen Y, Guo Y, Xiao S, Hu J, Wang W, Zhang W, Hu Y, Li Z, Zhang Z (2020) Quantifying the risk of hand, foot, and mouth disease (hfmd) attributable to meteorological factors in east china: a time series modelling study. Sci Total Environ 728:138548. https://doi.org/10.1016/j.scitotenv.2020.138548
Shi L, Zhao H, Wu D (2019) Modeling periodic hfmd with the effect of vaccination in mainland China. Complexity 2020:8763126. https://doi.org/10.1155/2020/8763126
Shih S, Sun F, Lee H (2019) Temporal pattern attention for multivariate time series forecasting. Mach Learn 108(8–9):1421–1441. https://doi.org/10.1007/s10994-019-05815-0
Wang Z, Cai B (2021) Covid-19 cases prediction in multiple areas via shapelet learning. Appl Intell 2021:1–12. https://doi.org/10.1007/s10489-021-02391-6
Wang Z, Huang Y, He B, Luo T, Wang Y, Lin Y (2019) TDDF: HFMD outpatients prediction based on time series decomposition and heterogenous data fusion in xiamen, china. In: Proceedings of the 15th international conference advanced data mining and applications, Dalian, China, pp 658–667. https://doi.org/10.1007/978-3-030-35231-8_48
Wang Z, Huang Y, Cai B, Ma R, Wang Z (2020a) Stock turnover prediction using search engine data. J Circuits Syst Comput. https://doi.org/10.1142/S021812662150122X
Wang Z, Huang Y, He B (2020b) Dual-grained representation for hfmd prediction within public health cyber-physical systems. Softw Pract Exp Early Access. https://doi.org/10.1002/spe.2940
Wang Z, Huang Y, He B, Luo T, Wang Y, Fu Y (2020) Short-term infectious diarrhea prediction using weather and search data in Xiamen, China. Sci Program 2020:1–12. https://doi.org/10.1155/2020/8814222
Wu Y, Yang Y, Nishiura H, Saitoh M (2018) Deep learning for epidemiological predictions. In: Proceedings of the 41st international ACM SIGIR conference on research and development in information retrieval, Ann Arbor, pp 1085–1088. https://doi.org/10.1145/3209978.3210077
Xiao Y, Yin H, Zhang Y, Qi H, Zhang Y, Liu Z (2021) A dual-stage attention-based conv-lstm network for spatio-temporal correlation and multivariate time series prediction. Int J Intell Syst 36(5):2036–2057. https://doi.org/10.1002/int.22370
Xing W, Liao Q, Viboud C, Zhang J, Sun J, Wu JT, Chang Z, Liu F, Fang VJ, Zheng Y, Cowling BJ, Varma JK, Farrar JJ, Leung GM, Yu H (2014) Hand, foot, and mouth disease in china, 2008–12: an epidemiological study. Lancet Infect Dis 14(4):308–318. https://doi.org/10.1016/S1473-3099(13)70342-6
Yang B, Liu F, Liao Q, Wu P, Chang Z, Huang J, Long L, Luo L, Leung G, Cowling B, Yu H (2017) Epidemiology of hand, foot and mouth disease in china, 2008 to 2015 prior to the introduction of ev-a71 vaccine. Eurosurveillance 22(50):1–10. https://doi.org/10.2807/1560-7917.ES.2017.22.50.16-00824
Ye H, Ma X, Pan Q, Fang H, Xiang H, Shao T (2019) An adaptive approach for anomaly detector selection and fine-tuning in time series. In: Proceedings of the 1st international workshop on deep learning practice for high-dimensional sparse data, New York, pp 1–7. https://doi.org/10.1145/3326937.3341253
Zhao H, Shi L, Wang J, Wang K (2021) A stage structure hfmd model with temperature-dependent latent period. Appl Math Model 93:745–761. https://doi.org/10.1016/j.apm.2021.01.010
Zhong R, Wu Y, Cai Y, Wang R, Zheng J, Lin D, Wu H, Li Y (2018) Forecasting hand, foot, and mouth disease in Shenzhen based on daily level clinical data and multiple environmental factors. Biosci Trends 12:450–455. https://doi.org/10.5582/bst.2018.01126
Zhuang F, Qi Z, Duan K, Xi D, Zhu Y, Zhu H, Xiong H, He Q (2021) A comprehensive survey on transfer learning. Proc IEEE 109(1):43–76. https://doi.org/10.1109/JPROC.2020.3004555
Acknowledgements
This work was supported in part by the Natural Science Foundation of Fujian Province (CN) (Nos. 2021J01857 and 2021J01859). The authors would like to thank the editor and anonymous reviewers for their helpful comments in improving the manuscript quality.
Author information
Authors and Affiliations
Corresponding authors
Ethics declarations
Conflict of interest
The authors declare that they have no known competing financial interests or personal relationships that could have appeared to influence the work reported in this paper.
Additional information
Publisher's Note
Springer Nature remains neutral with regard to jurisdictional claims in published maps and institutional affiliations.
Rights and permissions
About this article
Cite this article
Huang, Y., Zhang, P., Wang, Z. et al. HFMD Cases Prediction Using Transfer One-Step-Ahead Learning. Neural Process Lett 55, 2321–2339 (2023). https://doi.org/10.1007/s11063-022-10795-9
Accepted:
Published:
Issue Date:
DOI: https://doi.org/10.1007/s11063-022-10795-9