Abstract
Purpose
Respiratory motion of thoracic organs poses a severe challenge for the administration of image-guided radiotherapy treatments. Providing online and up-to-date volumetric information during free breathing can improve target tracking, ultimately increasing treatment efficiency and reducing toxicity to surrounding healthy tissue. In this work, a novel population-based generative network is proposed to address the problem of 3D target location prediction from 2D image-based surrogates during radiotherapy, thus enabling out-of-plane tracking of treatment targets using images acquired in real time.
Methods
The proposed model is trained to simultaneously create a low-dimensional manifold representation of 3D non-rigid deformations and to predict, ahead of time, the motion of the treatment target. The predictive capabilities of the model allow correcting target location errors that can arise due to system latency, using only a baseline volume of the patient anatomy. Importantly, the method does not require supervised information such as ground-truth registration fields, organ segmentation, or anatomical landmarks.
Results
The proposed architecture was evaluated on both free-breathing 4D MRI and ultrasound datasets. Potential challenges present in a realistic therapy, like different acquisition protocols, were taken into account by using an independent hold-out test set. Our approach enables 3D target tracking from single-view slices with a mean landmark error of 1.8 mm, 2.4 mm and 5.2 mm in volunteer MRI, patient MRI and US datasets, respectively, without requiring any prior subject-specific 4D acquisition.
Conclusions
This model presents several advantages over state-of-the-art approaches. Namely, it benefits from an explainable latent space with explicit respiratory phase discrimination. Thanks to the strong generalization capabilities of neural networks, it does not require establishing inter-subject correspondences. Once trained, it can be quickly deployed with an inference time of only 8 ms. The results show the capability of the network to predict future anatomical changes and track tumors in real time, yielding statistically significant improvements over related methods.










Similar content being viewed by others
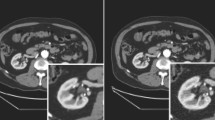
References
Arnold P, Preiswerk F, Fasel B, Salomir R, Scheffler K, Cattin PC (2011) 3D organ motion prediction for mr-guided high intensity focused ultrasound. In: International conference on medical image computing and computer-assisted intervention. Springer, pp 623–630
Balakrishnan G, Zhao A, Sabuncu MR, Guttag J, Dalca AV (2019) Voxelmorph: a learning framework for deformable medical image registration. IEEE Trans Med Imaging 38(8):1788–1800
Boye D, Samei G, Schmidt J, Székely G, Tanner C (2013) Population based modeling of respiratory lung motion and prediction from partial information. In: Medical imaging 2013: image processing. International Society for Optics and Photonics, p 86690U
Coupé P, Hellier P, Kervrann C, Barillot C (2009) Nonlocal means-based speckle filtering for ultrasound images. IEEE Trans Image Process 18(10):2221–2229
Ehrhardt J, Werner R, Schmidt-Richberg A, Handels H (2009) 4D motion modeling: estimation of respiratory motion for radiation therapy. Biological and medical physics, biomedical engineering, vol 25. Springer, Berlin
Fayad HJ, Buerger C, Tsoumpas C, Cheze-Le-Rest C, Visvikis D (2012) A generic respiratory motion model based on 4D MRI imaging and 2D image navigators. In: 2012 IEEE nuclear science symposium and medical imaging conference record (NSS/MIC)
Feng L, Axel L, Chandarana H, Block KT, Sodickson DK, Otazo R (2016) Xd-grasp: golden-angle radial MRI with reconstruction of extra motion-state dimensions using compressed sensing. Magn Reson Med 75(2):775–788
Garau N, Via R, Meschini G, Lee D, Keall PJ, Riboldi M, Baroni G, Paganelli C (2019) A ROI-based global motion model established on 4DCT and 2D cine-MRI data for MRI-guidance in radiation therapy. Phys Med Biol 64:045002
Giger A, Sandkühler R, Jud C, Bauman G, Bieri O, Salomir R, Cattin C (2018) Respiratory motion modelling using cgans. In: International conference on medical image computing and computer-assisted intervention. Springer, pp 81–88
Girdhar R, Fouhey DF, Rodriguez M, Gupta A (2016) Learning a predictable and generative vector representation for objects. In: European conference on computer vision. Springer, pp 484–499
Wendy H, Fang-Fang Y, Jing C, Lei R (2020) Volumetric cine magnetic resonance imaging (VC-MRI) using motion modeling, free-form deformation and multi-slice undersampled 2D cine MRI reconstructed with spatio-temporal low-rank decomposition. Quant Imaging Med Surg 10(2):432
Lauren H, Rojano K, Clifford R, Austen C, Todd DW, Jeffrey B, Olga G, Jeff M, Sasa M, Parag P (2018) Phase I trial of stereotactic MR-guided online adaptive radiation therapy (smart) for the treatment of oligometastatic or unresectable primary malignancies of the abdomen. Radiother Oncol 126(3):519–526
Jud C, Preiswerk F, Cattin PC (2015) Respiratory motion compensation with topology independent surrogates. In: Workshop on imaging and computer assistance in radiation therapy
Klein S, Staring M, Murphy K, Viergever MA, Pluim JPW (2009) Elastix: a toolbox for intensity-based medical image registration. IEEE Trans Med Imaging 29(1):196–205
Kurenkov A, Ji J, Garg A, Mehta V, Gwak JY, Choy C, Savarese S (2018) Deformnet: free-form deformation network for 3d shape reconstruction from a single image. In: 2018 IEEE winter conference on applications of computer vision (WACV). IEEE, pp 858–866
Kurz C, Buizza G, Landry G, Kamp F, Rabe M, Paganelli C, Baroni G, Reiner M, Keall PJ, van den Berg CAT (2020) Medical physics challenges in clinical MR-guided radiotherapy. Radiat Oncol 15:1–16
Küstner T, Fuin N, Hammernik K, Bustin A, Qi H, Hajhosseiny R, Masci PG, Neji R, Rueckert D, Botnar RM, Prieto C (2020) Cinenet: deep learning-based 3D cardiac cine MRI reconstruction with multi-coil complex-valued 4D spatio-temporal convolutions. Sci Rep 10(1):1–13
Mezheritsky T, Romaguera LV, Kadoury S (2020) 3D ultrasound generation from partial 2D observations using fully convolutional and spatial transformation networks. In: 2020 IEEE 17th international symposium on biomedical imaging (ISBI). IEEE, pp 1808–1811
Paganelli C, Lee D, Kipritidis J, Whelan B, Greer PB, Baroni G, Riboldi M, Keall P (2018) Feasibility study on 3D image reconstruction from 2D orthogonal cine-MRI for MRI-guided radiotherapy. J Med Imaging Radiat Oncol 62(3):389–400
Preiswerk F, De Luca V, Arnold P, Celicanin Z, Petrusca L, Tanner C, Bieri O, Salomir R, Cattin PC (2014) Model-guided respiratory organ motion prediction of the liver from 2D ultrasound. Med Image Anal 18(5):740–751
Romaguera LV, Plantefève R, Romero FP, Hébert F, Carrier J-F, Kadoury S (2020) Prediction of in-plane organ deformation during free-breathing radiotherapy via discriminative spatial transformer networks. Med Image Anal 64:101754
Seregni M, Paganelli C, Kipritidis J, Baroni G, Riboldi M (2017) Out-of-plane motion correction in orthogonal cine-MRI registration. Radiother Oncol 123:S147–S148
Stemkens B, Paulson ES, Tijssen RHN (2018) Nuts and bolts of 4D-MRI for radiotherapy. Phys Med Biol 63(21):21TR01
Stemkens B, Tijssen RHN, De Senneville BD, Lagendijk JJW, Van Den Berg CAT (2016) Image-driven, model-based 3d abdominal motion estimation for MR-guided radiotherapy. Phys Med Biol 61(14):5335
Tanner C, Yang M, Samei G, Székely G (2016) Influence of inter-subject correspondences on liver motion predictions from population models. In: 2016 IEEE 13th international symposium on biomedical imaging (ISBI). IEEE, pp 286–289
Tanner C, Zur Y, French K, Samei G, Strehlow J, Sat G, McLeod H, Houston G, Kozerke, Székely G, Melzer A Preusser T (2016) In vivo validation of spatio-temporal liver motion prediction from motion tracked on mr thermometry images. Int J Comput Assist Radiol Surg 11(6:1143–1152
von Siebenthal M, Székely G, Lomax A, Cattin PC (2007) Inter-subject modelling of liver deformation during radiation therapy. In: International conference on medical image computing and computer-assisted intervention. Springer, pp 659–666
von Siebenthal M, Szekely G, Gamper U, Boesiger P, Lomax A, Cattin PC (2007) 4D MR imaging of respiratory organ motion and its variability. Phys Med Biol 52(6):1547
Vorontsov E, Molchanov P, Byeon W, De Mello S, Jampani V, Liu M-Y, Kadoury S, Kautz J (2019) Boosting segmentation with weak supervision from image-to-image translation. arXiv preprint arXiv:1904.01636
Wilms M, Werner R, Yamamoto T, Handels H, Ehrhardt J (2017) Subpopulation-based correspondence modelling for improved respiratory motion estimation in the presence of inter-fraction motion variations. Phys Med Biol 62(14):5823
Funding
This work was partly funded by an NSERC collaborative research and development project (CRDPJ-517413-17) and by the Canada First Research Excellence Fund through the TransMedTech Institute.
Author information
Authors and Affiliations
Corresponding author
Ethics declarations
Conflict of interest
The authors declare that they have no conflict of interests.
Ethical approval
All procedures performed in studies involving human participants were in accordance with the ethical standards of the institutional and/or national research committee and with the 1964 Helsinki declaration and its later amendments or comparable ethical standards.
Informed consent
Informed consent was obtained from all individual participants included in the study.
Additional information
Publisher's Note
Springer Nature remains neutral with regard to jurisdictional claims in published maps and institutional affiliations.
Supplementary Information
Below is the link to the electronic supplementary material.
Rights and permissions
About this article
Cite this article
Romaguera, L.V., Mezheritsky, T., Mansour, R. et al. Predictive online 3D target tracking with population-based generative networks for image-guided radiotherapy. Int J CARS 16, 1213–1225 (2021). https://doi.org/10.1007/s11548-021-02425-x
Received:
Accepted:
Published:
Issue Date:
DOI: https://doi.org/10.1007/s11548-021-02425-x