Abstract
More complexities in urban areas due to the existence of buildings with roofs made of various materials, roads and parking lots, diverse plant species and vehicles along with developed very high spatial resolution (VHR) remote sensing imageries lead to increase the difficulties of urban objects recognition. Defining human cognition semantic categories of land cover objects is proposed in this paper to improve the object-based scene analysis. After performing the segmentation algorithm, semantically feature fusion of the VHR imagery and Lidar DSM is performed in the context of object-based procedures. For this reason, high-level semantics composed of human cognition object categorization and visual–conceptual descriptions of land cover objects in the complex urban scene is used for low-level features measurement followed by classification rules generation. The pan-sharpened WorldView-2 satellite image and Lidar DSM over a complex urban area in Rio de Janeiro (Brazil) are used to evaluate the capabilities of the proposed semantic object-based scene analysis. The evaluation of the obtained object recognition results shows the 93.98% overall accuracy and 0.937 Kappa coefficient. Comparing the obtained semantic object recognition results with non-semantic object-based procedures indicates the improvements in overall accuracy for 20.68 and 2.18% in object-based spectral and object-based spectral–structural classifications, respectively. Increasing the true positive pixels of the spectrally and height similar object categories (such as buildings and road), and using threshold training without requiring high-dimensional training samples are some of the advantages of the proposed semantic object-based scene analysis method.






Similar content being viewed by others
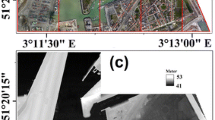
Data availability statement
The datasets analyzed during the current study are available in the IEEE GRSS data fusion committee at https://www.grss-ieee.org.
References
Abdi, G., Samadzadegan, F., Reinartz, P.: Deep learning decision fusion for the classification of urban remote sensing data. J. Appl. Remote Sens. 12(1), 016038 (2018). https://doi.org/10.1117/1.JRS.12.016038
Abdollahi, A., Pradhan, B., Shukla, N., Chakraborty, S., Alamri, A.: Multi-object segmentation in complex urban scenes from high-resolution remote sensing data. J. Remote Sens. 13, 3710 (2021). https://doi.org/10.3390/rs13183710
Baatz, M., Schape, A.: Multi-resolution segmentation: an optimization approach for high quality multi-scale image segmentation. In: Salzburg, T.S., Blaschke, T., Griesebner, G. (eds.) Angewandte Geographische Informationsverabeitung. XII. Beitragezum AGIT-Symp., pp. 12–23 (2000)
Bao, H., Ming, D., Guo, Y., Zhang, K., Zhou, K., Du, S.: DFCNN-based semantic recognition of urban functional zones by integrating remote sensing data and POI data. J. Remote Sens. 12, 1088 (2020). https://doi.org/10.3390/rs12071088
Blaschke, T.: Object based image analysis for remote sensing. ISPRS Int. J. Photogramm. Remote Sens. 65(1), 2–16 (2010)
Du, S., Zhang, F., Zhang, X.: Semantic classification of urban buildings combining VHR images and GIS data. In: GEOBIA 2016: Solutions and Synergies. University of Twente Faculty of Geo-Information and Earth Observation (ITC) (2016)
Du, S., Du, S., Liu, B., Zhang, X.: Incorporating DeepLabv3+ and object-based image analysis for semantic segmentation of very high resolution remote sensing images. Int. J. Digit. Earth 14(3), 357–378 (2020). https://doi.org/10.1080/17538947.2020.1831087
Jacquin, A., Misakova, L., Gay, M.: A hybrid object-based classification approach for mapping urban sprawl in periurban environment. Landsc. Urban Plan. 84(2008), 152–165 (2008)
Ji, S., Jun, H.: Deep Learning model for form recognition and structural member classification of East Asian traditional buildings. Sustainability 12, 5292 (2020). https://doi.org/10.3390/su12135292
Kussul, N., Lavreniuk, M., Skakun, S., Shelestov, A.: Deep learning classification of land cover and crop types using remote sensing data. IEEE Geosci. Remote Sens. Lett. (2017). https://doi.org/10.1109/LGRS.2017.2681128
Laliberte, A.S., Browning, D.M., Rango, A.: A comparison of three feature selection methods for object-based classification of sub-decimeter resolution UltraCam-L imagery. Int. J. Appl. Earth Obs. Geoinf. 15(2012), 70–78 (2012)
Marc-Zwecker, S., Asnoune, K., Wemmert, C.: A fuzzy-rule based ontology for urban object recognition. In: Proceedings of the International Conference on Knowledge Engineering and Ontology Development (KEOD-2014), pp. 153–160 (2014). https://doi.org/10.5220/0005026601530160
Masayu, N., Hanani, M.S., Zuraihan, M., Ashnita, R., Fazly, A.M., Helmi Zulhaidi M.S.: Urban building detection using object-based image analysis (OBIA) and machine learning (ML) algorithms. In: IOP Conference Series: Earth and Environmental Science, Advanced Geospatial and Surveying Conference, vol. 620 (2020)
Myint, S.W., Gober, P., Brazel, A., Grossman-Clarke, S., Weng, Q.: Per-pixel vs object-based classification of urban land cover extraction using high spatial resolution imagery. Remote Sens. Environ. (2011). https://doi.org/10.1016/j.rse.2010.12.017
Neupane, B., Horanont, T., Aryal, J.: Deep learning-based semantic segmentation of urban features in satellite images: a review and meta-analysis. J Remote Sens. 13, 808 (2021). https://doi.org/10.3390/rs13040808
Salehi, B., Zhang, Y., Zhong, M., Dey, V.: Object-based classification of urban areas using VHR imagery and height points ancillary data. J. Remote Sens. 4, 2256–2276 (2012). https://doi.org/10.3390/rs4082256
Tabib Mahmoudi, F., Samadzadegan, F., Reinartz, P.: Object oriented image analysis based on multi-agent recognition system. Comput. Geosci. 54, 219–230 (2013)
Tian, S., Ma, A., Zheng, Z., Zhong, Y.: Hi-UCD: a large-scale dataset for urban semantic change detection in remote sensing imagery, In: ML4D Workshop at Conference on Neural Information Processing Systems (NeurIPS 2020), Vancouver, Canada (2020)
Xie, X., Zhou, X., Li, J., Dai, W.: An ontology-based framework for complex urban object recognition through integrating visual features and interpretable semantics. Complexity (2020). https://doi.org/10.1155/2020/5125891
Yi, Y., Zhang, Z., Zhang, W., Zhang, C., Li, W., Zhao, T.: Semantic segmentation of urban buildings from VHR remote sensing imagery using a deep convolutional neural network. J. Remote Sens. 11, 1774 (2019). https://doi.org/10.3390/rs11151774
Zhang, L., Wang, S., Liu, B.: Deep learning for semantic analysis: a survey. Wiley Interdiscip. Rev. Data Min. Knowl. Discov. (2018). https://doi.org/10.1002/widm.1253
Zhao, Z., Bo, Y., Chen, J., Tiede, D., Blaschke, T., Emery, W.J.: Exploring semantic elements for urban scene recognition: deep integration of high-resolution imagery and OpenStreetMap (OSM). ISPRS J. Photogramm. Remote Sens. 151(2019), 237–250 (2019)
Author information
Authors and Affiliations
Contributions
Fatemeh Tabib Mahmoudi as the author of this manuscript, wrote the main text and prepare all of the figures, tables, results and discussions.
Corresponding author
Ethics declarations
Conflict of interest
The authors declare that they have no known competing financial interests or personal relationships that could have appeared to influence the work reported in this paper.
Additional information
Publisher's Note
Springer Nature remains neutral with regard to jurisdictional claims in published maps and institutional affiliations.
Rights and permissions
Springer Nature or its licensor (e.g. a society or other partner) holds exclusive rights to this article under a publishing agreement with the author(s) or other rightsholder(s); author self-archiving of the accepted manuscript version of this article is solely governed by the terms of such publishing agreement and applicable law.
About this article
Cite this article
Tabib Mahmoudi, F. Semantic object-based urban scene analysis for feature fusion of VHR imagery and Lidar DSM. SIViP 17, 1723–1731 (2023). https://doi.org/10.1007/s11760-022-02383-0
Received:
Revised:
Accepted:
Published:
Issue Date:
DOI: https://doi.org/10.1007/s11760-022-02383-0