Abstract
Atypical behavioral viewing pattern is one of the core deficits of individuals with Autism Spectrum Disorder (ASD). This diminishes their ability to understand the communicator’s facial emotional expression and often misinterpret one’s intended emotion. Here, we investigated the feasibility of using one’s gaze-related indices to estimate distinctive changes corresponding to various emotions. We designed a usability study with nine individuals with ASD and Typically Developing (TD) individuals who were exposed to Virtual Reality (VR) based social scenarios. The VR scenes presented virtual characters who narrated their social experience in the form of short stories with context-relevant emotional expressions. Simultaneously, we collected one’s gaze-related physiological indices (PIs) and behavioral looking pattern indices (BIs) using a technologically-enhanced eye-tracker. Subsequently, these PIs and BIs were used to classify the implications of the emotional expressions both within and across the ASD and TD groups. Results of the usability study indicate that one’s gaze-related indices can be discriminated with 97% accuracy for various emotions for intra-group analysis and 100% accuracy for inter-group analysis.







Similar content being viewed by others
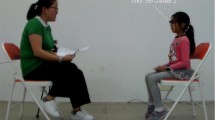
Explore related subjects
Discover the latest articles, news and stories from top researchers in related subjects.References
Access Policy Practice Advice: Autism Spectrum Disorder (2011) http://www.dhs.vic.gov.au/__data/assets/pdf_file/0010/681247/dsd_cis_access_policy_practice_advice_utism_spectrum_disorder_1011.pdf. Retrieved 1 May 2016
American Psychiatric Association (APA) (2013) Diagnostic and statistical manual of mental disorders (DSM-5®). American Psychiatric Pub
Aracena C, Basterrech S, Snáel V, Velásquez J (2015) Neural networks for emotion recognition based on eye tracking data. In: 2015 IEEE international conference on systems, man, and cybernetics, IEEE, pp 2632-2637
Autism cases rise in last two decades (2017) The Times of India. https://timesofindia.indiatimes.com/city/visakhapatnam/autism-cases-rise-in-last-two-decades/articleshow/57967289.cms. Retrieved 4 July 2018
Babu PRK, Oza P, Lahiri U (2018) Gaze-sensitive virtual reality based social communication platform for individuals with autism. IEEE Trans Affect Comput 9(4):450–462
Begeer S, Koot HM, Rieffe C, Terwogt MM, Stegge H (2008) Emotional competence in children with autism: diagnostic criteria and empirical evidence. Dev Rev 28(3):342–369
Behoora I, Tucker CS (2015) Machine learning classification of design team members’ body language patterns for real time emotional state detection. Des Stud 39:100–127
Bekele E, Crittendon J, Zheng Z, Swanson A, Weitlauf A, Warren Z, Sarkar N (2014) Assessing the utility of a virtual environment for enhancing facial affect recognition in adolescents with autism. J Autism Dev Disord 44(7):1641–1650
Bours CCAH, Bakker-Huvenaars MJ, Tramper J, Bielczyk N, Scheepers F, Nijhof KS, Buitelaar JK (2018) Emotional face recognition in male adolescents with autism spectrum disorder or disruptive behavior disorder: an eye-tracking study. Eur Child Adolesc Psychiatry 27(9):1143–1157
Breiman L (2017) Classification and regression trees. Routledge
Brock SE, Change C, Catewood V (2004) The identification of autism spectrum disorders. http://www.csus.edu/indiv/b/brocks/Workshops/NASP/Autism.pdf. Retrieved 22 June 2018
Chandler S, Charman T, Baird G, Simonoff E, Loucas TOM, Meldrum D, Pickles A (2007) Validation of the social communication questionnaire in a population cohort of children with autism spectrum disorders. J Am Acad Child Adolesc Psychiatry 46(10):1324–1332
Cheong S, Oh SH, Lee SY (2004) Support vector machines with binary tree architecture for multi-class classification. Neural Inform Process Lett Rev 2(3):47–51
Coon H, Villalobos ME, Robison RJ, Camp NJ, Cannon DS, Allen-Brady K, McMahon WM (2010) Genome-wide linkage using the Social responsiveness scale in utah autism pedigrees. Molecular Autism 1(1):8
Cortes C, Vapnik V (1995) Support-vector networks. Machine learning 20(3):273–297
Data and Statistics (2018) Centers for disease control and prevention. www.cdc.gov/ncbddd/autism/data. Retrieved 10 Apr 2018
Elisseeff A, Pontil M (2003) Leave-one-out error and stability of learning algorithms with applications. NATO Sci Ser Sub Ser iii Comput Syst Sci 190:111–130
Frazier TW, Klingemier EW, Parikh S, Speer L, Strauss MS, Eng C, Youngstrom EA (2018) Development and validation of objective and quantitative eye tracking—based measures of autism risk and symptom levels. J Am Acad Child Adolesc Psychiatry 57(11):858–866
Han L, Embrechts M, Szymanski B, Sternickel K, Ross A (2013) Sigma tuning of gaussian kernels: detection of ischemia fro-m magnetocardiograms. U.S. Patent No. 8,527,435. Washington, DC: U.S. Patent and Trademark Office
Hofmann SG, Smits JA (2008) Cognitive-behavioral therapy for adult anxiety disorders: a meta-analysis of randomized placebo-controlled trials. J Clin Psychiatry 69(4):621
Kliemann D, Dziobek I, Hatri A, Steimke R, Heekeren HR (2010) Atypical reflexive gaze patterns on emotional faces in autism spectrum disorders. J Neurosci 30(37):12281–12287
Kuriakose S, Lahiri U (2015) Understanding the psycho-physiological implications of interaction with a virtual reality-based system in adolescents with autism: a feasibility study. IEEE Trans Neural Syst Rehabil Eng 23(4):665–675
Matsuda S, Minagawa Y, Yamamoto J (2015) Gaze behavior of children with ASD toward pictures of facial expressions. Autism Res Treat. https://doi.org/10.1155/2015/617190
Nesterova A, Aysina R, Suslova T (2015) Recent technologies to improving social and communication skills in children with asd: systematization of approaches and methods. Mod Appl Sci 9(11):38
Nugrahaningsih N, Porta M (2014) Pupil size as a biometric trait. In: Cantoni V, Dimov D, Tistarelli M (eds) Biometric authentication. BIOMET 2014. Lecture notes in computer science, vol 8897. Springer, Cham, pp 222–233
Russell SJ, Norvig P (2016) Artificial intelligence: a modern approach. Pearson Education Limited, Malaysia
Saito T, Rehmsmeier M (2015) The precision-recall plot is more informative than the ROC plot when evaluating binary classifiers on imbalanced datasets. PLoS One 10(3):e0118432
Schurgin MW, Nelson J, Iida S, Ohira H, Chiao JY, Franconeri SL (2014) Eye movements during emotion recognition in faces. J Vision 14(13):14–14
Silberberg D, Arora N, Bhutani V, Durkin M, Gulati S (2013) Neuro-developmental disorders in India–an INCLEN Study (IN6-2.001). Neurology, 80(7 Supplement), IN6-2
Spence SH, Barrett PM, Turner CM (2003) Psychometric properties of the spence children’s anxiety scale with young adolescents. J Anxiety Disord 17(6):605–625
Teaching Aspergers Students Using Visual Imagery. My Aspergers Child (2007) http://www.myasperger-schild.com/2007/08/using-visual-thinking-skills-to-support.html. Retrieved 10 Aug 2016
Tharp JA, Wendelken C, Mathews CA, Marco EJ, Schreier H, Bunge SA (2015) Tourette syndrome: complementary insights from measures of cognitive control, eyeblink rate, and pupil diameter. Front Psychiatry 6:95
Tsang V (2018) Eye-tracking study on facial emotion recognition tasks in individuals with high-functioning autism spectrum disorders. Autism 22(2):161–170
Uljarevic M, Hamilton A (2013) Recognition of emotions in autism: a formal meta-analysis. J Autism Dev Disord 43(7):1517–1526
Vabalas A, Freeth M (2016) Brief report: patterns of eye movements in face to face conversation are associated with autistic traits: Evidence from a student sample. J Autism Dev Disord 46(1):305–314
Wang Q, Lu L, Zhang Q, Fang F, Zou X, Yi L (2018) Eye avoidance in young children with autism spectrum disorder is modulated by emotional facial expressions. J Abnorm Psychol 127(7):722
Watling R, Deitz J, Kanny EM, McLaughlin JF (1999) Current practice of occupational therapy for children with autism. Am J Occup Ther 53(5):498–505
Yoon HJ, Carmichael TR, Tourassi G (2014) Gaze as a biometric. In: Medical Imaging 2014: image perception, observer performance, and technology assessment, vol 9037. International Society for Optics and Photonics. https://www.spiedigitallibrary.org/conference-proceedings-of-spie/9037/903707/Gaze-as-a-biometric/10.1117/12.2044303.short?SSO=1
Acknowledgements
The authors wish to thank the Pearl Special Needs Foundation, Ahmedabad and KGP Hospital, Baroda for helping us in enrolling the participants in our study. Also we thank Cognitive Science Research Initiative under DST, India for partially funding this research. We would also like to express our gratitude to Ministry of Electronics and Information Technology, India for offering fellowship support to the researcher under Visvesvaraya PhD Scheme.
Author information
Authors and Affiliations
Corresponding author
Additional information
Publisher's Note
Springer Nature remains neutral with regard to jurisdictional claims in published maps and institutional affiliations.
Rights and permissions
About this article
Cite this article
Krishnappa Babu, P.R., Lahiri, U. Classification approach for understanding implications of emotions using eye-gaze. J Ambient Intell Human Comput 11, 2701–2713 (2020). https://doi.org/10.1007/s12652-019-01329-8
Received:
Accepted:
Published:
Issue Date:
DOI: https://doi.org/10.1007/s12652-019-01329-8