Abstract
Steganalysis plays a vital role in cybersecurity in today’s digital era where exchange of malicious information can be done easily across web pages. Steganography techniques are used to hide data in an object where the existence of hidden information is also obscured. Steganalysis is the process for detection of steganography within an object and can be categorized as active and passive steganalysis. Passive steganalysis tries to classify a given object as a clean or modified object. Active steganalysis aims to extract more details about hidden contents such as length of embedded message, region of inserted message, key used for embedding, required by cybersecurity experts for comprehensive analysis. Images being a viable source of exchange of information in the era of internet, social media are the most susceptible source for such transmission. Many researchers have worked and developed techniques required to detect and alert about such counterfeit exchanges over the internet. Literature present in passive and active image steganalysis techniques, addresses these issues by detecting and unveiling details of such obscured communication respectively. This paper provides a systematic and comprehensive review of work done on active image steganalysis techniques using deep learning techniques. This review will be helpful to the new researchers to become aware and build a strong foundation of literature present in active image steganalysis using deep learning techniques. The paper also includes various steganographic algorithms, dataset and performance evaluation metrics used in literature. Open research challenges and possible future research directions are also discussed in the paper.
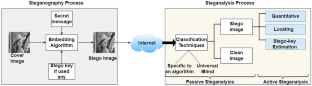










Similar content being viewed by others
Data availability
Not Applicable.
References
Ambalavanan A and Chandramouli R (2006) A Bayesian image steganalysis approach to estimate the embedded secret message. In: proceedings of the 7th multimedia and security workshop 2005, MM and Sec’05, vol 2006, pp 33–38. doi: https://doi.org/10.1145/1073170.1073177
Bagnall RJ (2002) Reversing the steganography myth in terrorist operations: the asymmetrical threat of simple intelligence dissemination techniques using common tools. Sans Inf Secur Read Room 19:1–16
Bas P, Filler T, Pevný T (2011) Break our steganographic system: the ins and outs of organizing BOSS. In: lecture notes in computer science (including subseries lecture notes in artificial intelligence and lecture notes in bioinformatics), vol 6958 LNCS, pp 59–70. doi: https://doi.org/10.1007/978-3-642-24178-9_5
Bedi P, Singhal A (2022) Estimating cover image for Universal payload region detection in Stego Images. J King Saud Univ Comput Inf Sci. https://doi.org/10.1016/J.JKSUCI.2022.01.010
Bengio Y, Louradour J, Collobert R, and Weston J (2009) Curriculum learning. In: proceedings of the 26th annual international conference on machine learning. vol 382, pp 41–48. doi: https://doi.org/10.1145/1553374.1553380
Bhatt C, Kumar I, Vijayakumar V, Singh KU, Kumar A (2021) The state of the art of deep learning models in medical science and their challenges. Multimed Syst 27(4):599–613. https://doi.org/10.1007/S00530-020-00694-1/FIGURES/10
Boroumand M, Chen M, Fridrich J (2018) Deep residual network for steganalysis of digital images. IEEE Trans Inf Forensics Secur 14(5):1181–1193. https://doi.org/10.1109/TIFS.2018.2871749
Chaumont M (2020) Deep learning in steganography and steganalysis. Digital media steganography: principles, algorithms, and advances. Elsevier, New York, pp 321–349. https://doi.org/10.1016/B978-0-12-819438-6.00022-0
Chen C and Shi YQ (2008) JPEG image steganalysis utilizing both intrablock and interblock correlations. In: proceedings-IEEE international symposium on circuits and systems, pp 3029–3032. doi: https://doi.org/10.1109/ISCAS.2008.4542096
Chen M, Sedighi V, Boroumand M, and Fridrich J (2017) JPEG-phase-aware convolutional neural network for steganalysis of JPEG images. In: IH and MMSec 2017-proceedings of the 2017 ACM workshop on information hiding and multimedia security, pp 75–84. doi: https://doi.org/10.1145/3082031.3083248.
Chen M, Boroumand M, and Fridrich J (2018) Deep learning regressors for quantitative steganalysis. In: is and t international symposium on electronic imaging science and technology, pp 160–167. doi: https://doi.org/10.2352/ISSN.2470-1173.2018.07.MWSF-160
Chutani S, Goyal A (2018) Improved universal quantitative steganalysis in spatial domain using ELM ensemble. Multimed Tools Appl 77(6):7447–7468. https://doi.org/10.1007/S11042-017-4656-3/FIGURES/7
Clark E (2019) GE Engineer Charged in Elaborate Theft of Trade Secrets. https://blog.twinstate.com/news/ge-trade-secrets-theft (Accessed Jan. 20, 2022)
Farooq N, Selwal A (2023) Image steganalysis using deep learning: a systematic review and open research challenges. J Ambient Intell Humaniz Comput 14(6):7761–7793. https://doi.org/10.1007/S12652-023-04591-Z
Fridrich J, Goljan M (2004) On estimation of secret message length in LSB steganography in spatial domain. Steganography Watermarking Multimedia Contents 5306:23. https://doi.org/10.1117/12.521350
Fridrich J, Kodovsky J (2012) Rich models for steganalysis of digital images. IEEE Trans Inf Forensics Secur 7(3):868–882. https://doi.org/10.1109/TIFS.2012.2190402
Fridrich J, Goljan M, Du R (2001) Steganalysis based on JPEG compatibility. Multimed Syst Appl IV 4518:275–280. https://doi.org/10.1117/12.448213
Fridrich J, Goljan M, Soukal D, Holotyak T (2005) Forensic steganalysis: determining the stego key in spatial domain steganography. Steganography Watermarking of Multimedia Contents 5681:631. https://doi.org/10.1117/12.585987
Fridrich J, Goljan M, and Soukal D (2004) Searching for the stego-key. In: security, steganography, and watermarking of multimedia contents VI, vol 5306, p. 70. doi: https://doi.org/10.1117/12.521353
Goodfellow I, Bengio Y, and Courville A (2016) Deep learning. MIT Press
Guan Q, Dong J, and Tan T, Blind Quantitative steganalysis based on feature fusion and gradient boosting. In: lecture notes in computer science (including subseries lecture notes in artificial intelligence and lecture notes in bioinformatics), 2010, vol 6526 LNCS, pp 266–279. doi: https://doi.org/10.1007/978-3-642-18405-5_22
Gui X, Li X, and Yang B (2012) Improved payload location for LSB matching steganography. In: proceedings-international conference on image processing, ICIP, pp 1125–1128. doi: https://doi.org/10.1109/ICIP.2012.6467062
Gul G, Kurugollu F (2010) Svd-based universal spatial domain image steganalysis. IEEE Trans Inf Forensics Secur 5(2):349–353. https://doi.org/10.1109/TIFS.2010.2041826
Hu J, Shen L, and Sun G (2018) Squeeze-and-excitation networks. In: proceedings of the IEEE conference on computer vision and pattern recognition, pp 7132–7141
Hussain I, Zeng J, Xinhong X, Tan S (2020) A survey on deep convolutional neural networks for image steganography and steganalysis. KSII Trans Int Inf Syst 14(3):1228–1248. https://doi.org/10.3837/TIIS.2020.03.017
Ker AD (2008) Locating Steganographic Payload via WS Residuals. In: proceedings of the 10th ACM workshop on multimedia and security, pp 27–32
Ker AD and Lubenko (2009) Feature reduction and payload location with WAM steganalysis. In: media forensics and security, international society for optics and photonics, p 72540A
Ker AD, Böhme R (2008) Revisiting weighted stego-image steganalysis. Forensics Steganography Watermarking Multimedia Contents 6819:681905. https://doi.org/10.1117/12.766820
Kodovský J, Fridrich J, Holub V (2012) Ensemble classifiers for steganalysis of digital media. IEEE Trans Inf Forensics Secur 7(2):432–444. https://doi.org/10.1109/TIFS.2011.2175919
Kodovský J and Fridrich J (2010) Quantitative steganalysis of LSB embedding in JPEG domain. In: MM and sec’10-proceedings of the 2010 ACM SIGMM multimedia and security workshop, pp 187–197. doi: https://doi.org/10.1145/1854229.1854265
Kodovský J andx Fridrich J (2013) Quantitative steganalysis using rich models. In: media watermarking, security, and forensics, vol 8665: 86650. doi: https://doi.org/10.1117/12.2001563
Kodovsky J, Fridrich J (2010) Quantitative structural steganalysis of Jsteg. IEEE Trans Inf Forensics Secur 5(4):681–693. https://doi.org/10.1109/TIFS.2010.2056684
Kong XW, Liu WF, and You XG (2005) Secret message location steganalysis based on local coherences of hue. In: lecture notes in computer science (including subseries lecture notes in artificial intelligence and lecture notes in bioinformatics), vol 3768 LNCS, pp 301–311. doi: https://doi.org/10.1007/11582267_27
Kumar V, Sharma S, Kumar C, Vishwa A, Sahu AK (2023) Latest trends in deep learning techniques for image steganography. Int J Digit Crime Forensics 15(1):1–14. https://doi.org/10.4018/IJDCF.318666
Lie WN, Lin GS (2005) A feature-based classification technique for blind image steganalysis. IEEE Trans Multimed 7(6):1007–1020. https://doi.org/10.1109/TMM.2005.858377
Liu J, Tang G (2012) Stego key estimation in LSB steganography. J Multimed 7(4):309. https://doi.org/10.4304/jmm.7.4.277-278
Liu J, Tian Y, Han T, Yang C, Liu W (2015) LSB steganographic payload location for JPEG-decompressed images. Digit Signal Process 38:66–76. https://doi.org/10.1016/j.dsp.2014.12.004
Liu J, Tian Y, Han T, Wang J, Luo X (2016) Stego key searching for LSB steganography on JPEG decompressed image. Sci China Inf Sci 59(3):1–15. https://doi.org/10.1007/S11432-015-5367-X
Liu J, Yang C, Wang J, Shi Y (2020) Stego key recovery method for F5 steganography with matrix encoding. Eurasip J Image Video Process 2020(1):1–17. https://doi.org/10.1186/S13640-020-00526-2
Lou DC, Chou CL, Tso HK, Chiu CC (2012) Active steganalysis for histogram-shifting based reversible data hiding. Opt Commun 285(10–11):2510–2518. https://doi.org/10.1016/j.optcom.2012.01.021
Lu J, Yang C, Liu F, Luo X (2018) Quantitative steganalysis of JPEG additive steganography based on boundary effect. J Electron Imaging 27(02):1. https://doi.org/10.1117/1.jei.27.2.023004
Lyu S, Farid H (2006) Steganalysis using higher-order image statistics. IEEE Trans Inf Forensics Secur 1(1):111–119. https://doi.org/10.1109/TIFS.2005.863485
Malhotra R, Singh P (2023) Recent advances in deep learning models: a systematic literature review. Multimed Tools Appl 2023:1–84. https://doi.org/10.1007/S11042-023-15295-Z
Mandal PC, Mukherjee I, Paul G, Chatterji BN (2022) Digital image steganography: a literature survey. Inf Sci (ny) 609:1451–1488. https://doi.org/10.1016/J.INS.2022.07.120
Mathew A, Amudha P, Sivakumari S (2021) Deep learning techniques: an overview. Adv Intell Syst Comput 1141:599–608. https://doi.org/10.1007/978-981-15-3383-9_54/TABLES/2
Mo X, Tan S, Tang W, Li B, Huang J (2023) ReLOAD: using reinforcement learning to optimize asymmetric distortion for additive steganography. IEEE Trans Inf Forensics Secur 18:1524–1538. https://doi.org/10.1109/TIFS.2023.3244094
Muralidharan T, Cohen A, Cohen A, Processing NN-S, and undefined 2022 (2022) “The infinite race between steganography and steganalysis in images,” Signal Processing, vol 201, p 108711, Accessed: Jan 25, 2023. [Online]. Available: https://www.sciencedirect.com/science/article/pii/S016516842200250X?casa_token=t0S5VoPVAi8AAAAA:s5gDY44ig26t6_iLJ8FPDrEAjEZOM4j76RKqUlp2Dck4hmFAYL7osHZtdruhqny1zjVXT_2sCw
Nissar A, Mir AH (2010) Classification of steganalysis techniques: a study. Digit Signal Process A Rev J 20(6):1758–1770. https://doi.org/10.1016/j.dsp.2010.02.003
Pevný T, Fridrich J, Ker AD (2012) From blind to quantitative steganalysis. IEEE Trans Inf Forensics Secur 7(2):445–454. https://doi.org/10.1109/TIFS.2011.2175918
Pevný T and Ker AD (2014) Steganographic key leakage through payload metadata. In: IH and MMSec 2014-proceedings of the 2014 ACM information hiding and multimedia security workshop. pp 109–114. doi: https://doi.org/10.1145/2600918.2600921.
Provos N and Honeyman P, Detecting steganographic content on the internet. In: USA Today, vol. 1001, no. 01–11, pp. 48103–4943, Aug. 2001, Accessed: Jan. 23, 2022. [Online]. Available: http://scholar.google.com/scholar?hl=en&btnG=Search&q=intitle:Detecting+Steganographic+Content+on+the+Internet#0
Qian Y, Dong J, Wang W, and Tan T (2016) Learning and transferring representations for image steganalysis using convolutional neural network. In: proceedings-international conference on image processing, ICIP, vol 2016 pp 2752–2756. doi: https://doi.org/10.1109/ICIP.2016.7532860.
Quach TT (2011) Optimal cover estimation methods and steganographic payload location. IEEE Trans Inf Forensics Secur 6(4):1214–1222. https://doi.org/10.1109/TIFS.2011.2160855
Quach T-T (2014) Cover estimation and payload location using Markov random fields. In: Media Watermarking, Security, and Forensics 2014, vol 9028, p 90280H. doi: https://doi.org/10.1117/12.2032711
Ruan F, Zhang X, Zhu D, Xu Z, Wan S, Qi L (2020) Deep learning for real-time image steganalysis: a survey. J Real-Time Image Process 17(1):149–160. https://doi.org/10.1007/S11554-019-00915-5/TABLES/6
Schaefer G and Stich M (2003) UCID: an uncompressed color image database. In: storage and retrieval methods and applications for multimedia 2004, vol 5307, pp 472–480. doi: https://doi.org/10.1117/12.525375
Selvaraj A, Ezhilarasan A, Wellington SLJ, Sam AR (2021) Digital image steganalysis: a survey on paradigm shift from machine learning to deep learning based techniques. IET Image Process 15(2):504–522. https://doi.org/10.1049/ipr2.12043
Simmons GJ (1984) The prisoners problem. Advances in cryptology: proceedings of crypto, 83, pp 51–67. [Online]. Available: http://www.cs.nccu.edu.tw/~raylin/UndergraduateCourse/ComtenporaryCryptography/Spring2009/ThePrisonerProblem.pdf
Singh P, Gupta S, Gupta V (2023) Multi-objective hyperparameter optimization on gradient-boosting for breast cancer detection. Int J Syst Assur Eng Manag 1:1–11. https://doi.org/10.1007/S13198-023-01955-8/TABLES/8
Singhal A and Bedi P, Blind Quantitative Steganalysis using SVD Features. In: 2018 international conference on advances in computing, communications and informatics, ICACCI 2018, 2018, pp. 369–374. doi: https://doi.org/10.1109/ICACCI.2018.8554947.
Singhal A and Bedi P, “Blind quantitative steganalysis using CNN–long short-term memory architecture. In: strategic system assurance and business analytics. Asset analytics (performance and safety management), pp 175–186. doi: https://doi.org/10.1007/978-981-15-3647-2_14
Singhal A and Bedi P (2022) Universal Quantitative steganalysis using deep residual networks. In: international conference on innovative computing and communications. Advances in intelligent systems and computing, pp 465–475. doi: https://doi.org/10.1007/978-981-16-3071-2_37
Singhal A, Bedi P (2021) Multi-class blind steganalysis using deep residual networks. Multimed Tools Appl 80(9):13931–13956. https://doi.org/10.1007/s11042-020-10353-2
Stier C (2010) Russian spy ring hid secret messages on the web. New Sci. https://www.newscientist.com/article/dn19126-russian-spy-ring-hid-secret-messages-on-the-web/ (Accessed Jan. 19, 2022).
Sun Y, Zhang H, Zhang T, Wang R (2019) Deep neural networks for efficient steganographic payload location. J Real-Time Image Process 16(3):635–647. https://doi.org/10.1007/S11554-019-00849-Y/TABLES/7
Sun Y and Li T (2019) A Method for Quantitative steganalysis based on deep learning. In: 2019 2nd international conference on information systems and computer aided education, ICISCAE 2019, pp 302–309. doi: https://doi.org/10.1109/ICISCAE48440.2019.221640.
Tabares-Soto R, Raúl RP, Gustavo I (2019) Deep learning applied to steganalysis of digital images: a systematic review. IEEE Access 7:68970–68990. https://doi.org/10.1109/ACCESS.2019.2918086
Tang W, Li B, Barni M, Li J, Huang J (2021) An automatic cost learning framework for image steganography using deep reinforcement learning. IEEE Trans Inf Forensics Secur 16:952–967. https://doi.org/10.1109/TIFS.2020.3025438
Trivedi S, Chandramouli R (2005) Secret key estimation in sequential steganography. IEEE Trans Signal Process 53(2):746–757. https://doi.org/10.1109/TSP.2004.839925
Veena ST, Arivazhagan S (2018) Quantitative steganalysis of spatial LSB based stego images using reduced instances and features. Patt Recognit Lett 105:39–49. https://doi.org/10.1016/j.patrec.2017.08.016
Veena ST, Arivazhagan S (2019) Universal secret payload location identification in spatial LSB stego images. Ann Des Telecommun Telecommun 74(5–6):273–286. https://doi.org/10.1007/S12243-018-0676-X/TABLES/3
Wang Y, Moulin P (2007) Optimized feature extraction for learning-based image steganalysis. IEEE Trans Inf Forensics Secur 2(1):31–45. https://doi.org/10.1109/TIFS.2006.890517
Wang J, Yang C, Wang P, Song X, Lu J (2020) Payload location for JPEG image steganography based on co-frequency sub-image filtering. Int J Distrib Sens Netw 16(1):1550147719899569. https://doi.org/10.1177/1550147719899569
Wang J, Yang C, Zhu M, Song X, Liu Y, Lian Y (2021) JPEG image steganography payload location based on optimal estimation of cover co-frequency sub-image. Eurasip J Image Video Process 2021(1):1–14. https://doi.org/10.1186/S13640-020-00542-2/FIGURES/7
Wu S, Zhong S, Liu Y (2018) Deep residual learning for image steganalysis. Multimed Tools Appl 77(9):10437–10453. https://doi.org/10.1007/s11042-017-4440-4
Xu G, Wu HZ, Shi YQ (2016a) Structural design of convolutional neural networks for steganalysis. IEEE Signal Process Lett 23(5):708–712. https://doi.org/10.1109/LSP.2016.2548421
Xu C, Liu J, Gan J, Luo X (2018) Stego key recovery based on the optimal hypothesis test. Multimed Tools Appl 77(14):17973–17992. https://doi.org/10.1007/s11042-017-4878-4
Xu G, Wu HZ, and Shi YQ (2016) Ensemble of CNNs for steganalysis: an empirical study. In: IH and MMSec 2016-proceedings of the 2016 ACM information hiding and multimedia security workshop, pp 103–107. doi: https://doi.org/10.1145/2909827.2930798.
Yang C, Luo X, Lu J, Liu F (2018) Extracting hidden messages of MLSB steganography based on optimal stego subset. Sci China Inf Sci 61(11):3. https://doi.org/10.1007/s11432-017-9328-2
Yang C, Liu F, Ge S, Lu J, Huang J (2019) Locating secret messages based on quantitative steganalysis. Math Biosci Eng 16(5):4908–4922. https://doi.org/10.3934/mbe.2019247
Ye J, Ni J, Yi Y (2017) Deep learning hierarchical representations for image steganalysis. IEEE Trans Inf Forensics Secur 12(11):2545–2557. https://doi.org/10.1109/TIFS.2017.2710946
Yedroudj M, Comby F, and Chaumont M (2018) Yedroudj-net: an efficient CNN for spatial steganalysis. In: ICASSP, IEEE international conference on acoustics, speech and signal processing-proceedings, 2018, pp 2092–2096. doi: https://doi.org/10.1109/ICASSP.2018.8461438.
You W, Zhang H, Zhao X (2021) A siamese CNN for image steganalysis. IEEE Trans Inf Forensics Secur 16:291–306. https://doi.org/10.1109/TIFS.2020.3013204
Zeng J, Tan S, Li B, Huang J (2017) Large-scale JPEG image steganalysis using hybrid deep-learning framework. IEEE Trans Inf Forensics Secur 13(5):1200–1214. https://doi.org/10.1109/TIFS.2017.2779446
Zou Y, Zhang G, Liu L (2019) Research on image steganography analysis based on deep learning. J vis Commun Image Represent 60:266–275. https://doi.org/10.1016/J.JVCIR.2019.02.034
Acknowledgements
We seriously thank the editor and anonymous reviewers for their valuable comments and suggestions which have immensely helped in improving the quality of our paper.
Funding
No funding was received to assist with the preparation of this manuscript.
Author information
Authors and Affiliations
Corresponding author
Ethics declarations
Conflict of interest
The authors declare that they have no known competing financial interests or personal relationships that could have appeared to influence the work reported in this paper.
Additional information
Publisher's Note
Springer Nature remains neutral with regard to jurisdictional claims in published maps and institutional affiliations.
Appendix 1
Rights and permissions
Springer Nature or its licensor (e.g. a society or other partner) holds exclusive rights to this article under a publishing agreement with the author(s) or other rightsholder(s); author self-archiving of the accepted manuscript version of this article is solely governed by the terms of such publishing agreement and applicable law.
About this article
Cite this article
Bedi, P., Singhal, A. & Bhasin, V. Deep learning based active image steganalysis: a review. Int J Syst Assur Eng Manag 15, 786–799 (2024). https://doi.org/10.1007/s13198-023-02203-9
Received:
Revised:
Accepted:
Published:
Issue Date:
DOI: https://doi.org/10.1007/s13198-023-02203-9