Abstract
The magnetic shape memory alloy (MSMA)-based actuator, as a new type of actuator, has a great application prospect in the micro-precision positioning field. However, the input-to-output hysteresis nonlinearity largely hinders its wide application. In this paper, a Takagi–Sugeno fuzzy neural network (TSFNN) model based on the modified bacteria foraging algorithm (MBFA) is innovatively utilized to describe the complex hysteresis nonlinearity of the MSMA-based actuator, and the parameters of TSFNN are optimized by the MBFA. The TSFNN is a combination of the fuzzy-logic system and neural network; thus, it has the capability of approximating the nonlinear mapping function and self-adjustment and is suitable for hysteresis modeling. The MBFA, which can obtain better optimization values, is employed for the parameter identification procedure. To demonstrate the effectiveness of the proposed model, a TSFNN based on the gradient descent algorithm (GDA) is used for comparison. Experimental results clearly show that the proposed modeling method can accurately describe the hysteresis nonlinearity of the MSMA-based actuator and has significance for its future application.










Similar content being viewed by others
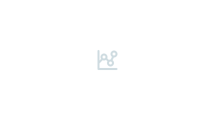
References
Kohl, M., Gueltig, M., Pinneker, V., Yin, R., Wendler, F., Krevet, B.: Magnetic shape memory microactuators. Micromachines 5(4), 1135–1160 (2014)
Jani, J., Leary, M., Subic, A., Gibson, M.: A review of shape memory alloy research, applications and opportunities. Mater. Des. 56, 1078–1113 (2014)
Zhang, Q.X., Fu, Q.H., Wang, L.P., Gao, Y.H.: Research and experimental analysis of damping characteristics of magnetic shape memory alloy. Trans. Electr. Electron. Mater. 19(4), 272–278 (2018)
Faran, E., Shilo, D.: Ferromagnetic shape memory alloys-challenges, applications, and experimental characterization. Exp. Tech. 40, 1005–1031 (2016)
Oonishi, A., Hirata, K., Yoo, B., Niguchi, N.: Frequency response characteristics for linear actuator made by NiMnGa shape memory alloy. Int. J. Appl. Electromagn. Mech. 39(1–4), 913–918 (2012)
Zhang, X.Y., Wang, Y., Wang, C.L., Su, C.-Y., Li, Z., Chen, X.K.: Adaptive estimated inverse output-feedback quantized control for piezoelectric positioning stage. IEEE. Trans. Cybern. 49(6), 2106–2118 (2019)
Yu, Y.W., Zhang, C., Zhou, M.L.: NARMAX model-based hysteresis modeling of magnetic shape memory alloy actuators. IEEE Trans. Nanotechnol. 19, 1–4 (2020)
Baghel, A., Kulkarni, S.: Parameter identification of the Jiles–Atherton hysteresis model using a hybrid technique. IET Electr. Power Appl. 6(9), 689–695 (2012)
Xu, R., Zhou, M.L.: Elman neural network-based identification of Krasnosel’skii–Pokrovskii model for magnetic shape memory alloys actuator. IEEE Trans. Magn. 53, Article ID 2002004 (2017)
Minorowicz, B., Nowak, A., Stefanski, F.: Hysteresis modelling in electromechanical transducer with magnetic shape memory alloy. Przeglad Elektrotechniczny 11, 244–247 (2014)
Tu, F.Q., Hu, S.M., Zhuang, Y.H., Lv, J., Wang, Y.X., Sun, Z.: Hysteresis curve fitting optimization of magnetic controlled shape memory alloy actuator. Actuators 5(4), 25 (2016)
Son, N.N., Anh, H.P.H.: Adaptive displacement online control of shape memory alloys actuator based on neural networks and hybrid differential evolution algorithm. Neurocomputing 166, 464–474 (2015)
Zhou, M.L., Wang, S.B., Gao, W.: Neural network model for hysteresis nonlinearity of magnetic shape memory alloy actuator. J. Comput. Theor. Nanosci. 10(12), 2931–2935 (2013)
Zhou, M.L., Wang, Y.F., Xu, R., Zhang, Q., Zhu, D.: Feed-forward control for magnetic shape memory alloy actuators based on the radial basis function neural network model. J. Appl. Biomater Funct. Mater. 15(suppl 1), 25–30 (2017)
Yilmaz, S., Oysal, Y.: Fuzzy wavelet neural network models for prediction and identification of dynamical systems. IEEE Trans. Neural Netw. Learn. Syst. 21(10), 1599–1609 (2010)
Lin, D., Wang, X.Y., Nian, F.Z., Zhang, Y.L.: Dynamic fuzzy neural networks modeling and adaptive backstepping tracking control of uncertain chaotic systems. Neurocomputing 73(16–18), 2873–2881 (2010)
Juang, C., Hsieh, C.: A locally recurrent fuzzy neural network with support vector regression for dynamic-system modeling. IEEE Trans. Fuzzy Syst. 18(2), 261–273 (2010)
Zhang, S., Jiang, H., Yin, Y., Xiao, W., Zhao, B.: The prediction of the gas utilization ratio based on TS fuzzy neural network and particle swarm optimization. Sensors 18(2), 625 (2018)
Liu, J., Yin, T., Xie, X., Tian, E., Fei, S.: Event-triggered state estimation for T–S fuzzy neural networks with stochastic cyber-Attacks. Int. J. Fuzzy Syst. 21(2), 532–544 (2019)
Zhang, K., Qian, F., Liu, M.: A survey on fuzzy neural network technology. Inf. Control 32(5), 431–435 (2003)
Shah, H., Tairan, N., Garg, H., Ghazali, R.: Global gbest guided-artificial bee colony algorithm for numerical function optimization. Computers 7(4), 69 (2018)
Garg, H.: Solving structural engineering design optimization problems using an artificial bee colony algorithm. J. Ind. Manag. Opt. 10(3), 777–794 (2014)
Garg, H.: An efficient biogeography based optimization algorithm for solving reliability optimization problems. Swarm Evol. Comput. 24, 1–10 (2015)
Garg, H.: A hybrid GSA-GA algorithm for constrained optimization problems. Inf. Sci. 478, 499–523 (2019)
Garg, H.: A hybrid PSO-GA algorithm for constrained optimization problems. Appl. Math. Comput. 274, 292–305 (2016)
Haber, R.E., Beruvides, G., Quiza, R., Hernandez, A.: A simple multi-objective optimization based on the cross-entropy method. IEEE Access 5, 22272–22281 (2017)
Beruvides, G., Quiza, R., Haber, R.E.: Multi-objective optimization based on an improved cross-entropy method. A case study of a micro-scale manufacturing process. Inf. Sci. 334–335, 161–173 (2016)
La Fe-Perdomo, I., Beruvides, G., Quiza, R., Haber, R.E., Rivas, M.: Automatic selection of optimal parameters based on simple soft-computing methods: a case study of micromilling processes. IEEE Trans. Ind. Inf. 15(2), 800–811 (2019)
Chen, H., Zhu, Y., Hu, K.: Adaptive bacterial foraging optimization. Abstr. Appl. Anal. 2011, Article ID 108269 (2011)
Korani, W., Dorrah, H., Emara, H.: Bacterial foraging oriented by particle swarm optimization strategy for PID tuning. In: IEEE international symposium computational intelligence robotics automation (CIRA). pp. 445–450 (2009)
Farhy, L.S.: Modeling of oscillations in endocrine networks with feedback. Method. Enzymol. 384, 54–81 (2004)
Wang, L., Tang, D.B.: An improved adaptive genetic algorithm based on hormone modulation mechanism for job-shop scheduling problem. Exp. Syst. Appl. 38(6), 7243–7250 (2011)
Sadeghzadeh, A., Asua, E., Feuchtwanger, J., Etxebarria, V., Garcia-Arribas, A.: Ferromagnetic shape memory alloy actuator enabled for nanometric position control using hysteresis compensation. Sens. Actuators Phys. 182, 122–129 (2012)
Sarawate, N., Dapino, M.: Dynamic sensing behavior of ferromagnetic shape memory Ni-Mn-Ga. Smart Mater. Struct. 18, Article ID 104014 (2009)
Schluter, K., Riccardi, L., Raatz, A.: An open-loop control approach for magnetic shape memory actuators considering temperature variations. Adv. Sci. Technol. 78, 119–124 (2013)
Lin, J.H., Chiang, M.H.: Tracking control of a magnetic shape memory actuator using an inverse Preisach model with modified fuzzy sliding mode control. Sensors 16(9), 1368 (2016)
Sutor, A., Rupitsch, S., Lerch, R.: A Preisach-based hysteresis model for magnetic and ferroelectric hysteresis. Appl. Phys. A 100(2), 425–430 (2010)
Minorowicz, B., Stefanski, F., Sedziak, D.: Hysteresis modeling and position control of actuator with magnetic shape memory alloy. In: International carpathian control conference, pp. 505–510 (2016)
Minorowicz, B., Leonetti, G., Stefanski, F., Binetti, G., Naso, D.: Design, modelling and control of a micro-positioning actuator based on magnetic shape memory alloys. Smart Mater. Struct. 25, Article ID 075005 (2016)
Shakiba, S., Zakerzadeh, M., Ayati, M.: Experimental characterization and control of a magnetic shape memory alloy actuator using the modified generalized rate-dependent Prandtl–Ishlinskii hysteresis model. Proc. Inst. Mech. Eng. Part I J. Syst. Control Eng. 232(5), 506–518 (2018)
Zhou, M.L., He, S.B., Hu, B., Zhang, Q.: Modified KP model for hysteresis of magnetic shape memory alloy actuator. IETE Tech. Rev. 32(1), 29–36 (2015)
Riccardi, L., Naso, D., Janocha, H., Turchiano, B.: A precise positioning actuator based on feedback-controlled magnetic shape memory alloys. Mechatronics 22(5), 568–576 (2012)
Zhou, M.L., Zhang, Q.: Hysteresis model of magnetically controlled shape memory alloy based on a PID neural network. IEEE Trans. Magn. 51, Article ID 7301504 (2015)
Serpico, C., Visone, C.: Magnetic hysteresis modeling via feed-forward neural networks. IEEE Trans. Magn. 34(3), 623–628 (1998)
Tai, N., Ahn, K.: A hysteresis functional link artificial neural network for identification and model predictive control of SMA actuator. J. Process Control 22(4), 766–777 (2012)
Wang, H., Song, G.B.: Innovative NARX recurrent neural network model for ultra-thin shape memory alloy wire. Neurocomputing 134, 289–295 (2014)
Liu, Y., Yang, D.K., Nan, N., Li, G., Zhang, J.J.: Strong convergence analysis of batch gradient-based learning algorithm for training pi-sigma network based on TSK fuzzy models. Neural Process Lett. 43, 745–758 (2016)
Passino, K.: Biomimicry of bacterial foraging for distributed optimization and control. IEEE Control Syst. Mag. 22(3), 52–67 (2002)
Eberhart, R., Kennedy, J.: Particle swarm optimization. Proc. IEEE Int. Conf. Neural. Netw. 4, 1942–1948 (1995)
Roberge, V., Tarbouchi, M., Labonte, G.: Comparison of parallel genetic algorithm and particle swarm ptimization for real-time UAV path planning. IEEE Trans. Ind. Inf. 9(1), 132–141 (2013)
Sun, Z.Y., Bo, S., Xi, N., Yang, R.G., Hao, L.N., Chen, L.L.: Compensating asymmetric hysteresis for nanorobot motion control. In: Proceedings of the IEEE international conference robotics Automation. pp. 3501–3506 (2015)
Sun, K.K., Qiu, J.B., Karimi, H.R., Gao, H.J.: A Novel finite-time control for nonstrict feedback saturated nonlinear systems with tracking error constraint. IEEE Trans. Syst. Man Cy.-S. 1, 1 (2019). https://doi.org/10.1109/TSMC.2019.2958072
Sun, K.K., Mou, S.S., Qiu, J.B., Wang, T., Gao, H.J.: Adaptive fuzzy control for non-triangular structural stochastic switched nonlinear systems with full state constraints. IEEE Trans. Fuzzy Syst. 27(8), 1587–1601 (2018)
Acknowledgements
This work was supported in part by the National Natural Science Foundation of China under Grant 51675228 and the Program of Science and Technology Development Plan of Jilin Province of China under Grants 20180101052JC, 20190303020SF.
Author information
Authors and Affiliations
Contributions
All authors contributed to the study conception and design. Material preparation, data collection, and analysis were performed by [CZ], [YY], and [YW]. The manuscript was written by [CZ] and [MZ]. [MZ], [YY], and [YW] reviewed and edited this manuscript. The funding acquisition and supervision were performed by [MZ]. All authors read and approved the manuscript.
Corresponding author
Rights and permissions
About this article
Cite this article
Zhang, C., Yu, Y., Wang, Y. et al. Takagi–Sugeno Fuzzy Neural Network Hysteresis Modeling for Magnetic Shape Memory Alloy Actuator Based on Modified Bacteria Foraging Algorithm. Int. J. Fuzzy Syst. 22, 1314–1329 (2020). https://doi.org/10.1007/s40815-020-00826-9
Received:
Revised:
Accepted:
Published:
Issue Date:
DOI: https://doi.org/10.1007/s40815-020-00826-9