Abstract
A more efficient technique to guarantee safety and security in a variety of settings is through video surveillance, also known as closed circuit television (CCTV). It is frequently employed in strategic sectors, including security at home, public transportation, banks, and ATMs’ hubs, commercial districts, airports, and public roadways, and it is crucial for safeguarding crucial infrastructures. Due to the numerous uses, human detection in surveillance system video scenes has therefore grown in prominence in recent years. Objects of interest should be able to be found, categorized, and tracked by a real-time video surveillance system. This study provides an in-depth analysis of such video surveillance systems and presents a full assessment of methods and data sets utilized in human (object) detection. The most significant analyses of these systems are provided along with the employed architectures. To provide a clearer image and a comprehensive overview of the system, existing surveillance systems were compared in terms of their features, advantages, and challenges. These comparisons are summarized in this document. Future trends are also examined, laying the groundwork for new study avenues.








Similar content being viewed by others
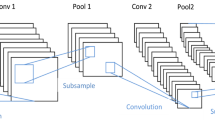
Data availability
Data availability not applicable for this research work.
References
Teddy K. A survey on behavior analysis in video surveillance applications, video surveillance, Prof. Weiyao Lin (Ed.), ISBN: 978-953-307-436-8, 2011. Available: http://www.intechopen.com/books/videosurveillanc-e/a-survey-on-behavior-analysis-in-video-surveillanceapplications.
Pawan Kumar Mishra Uttrakhand Technical University Dehradun, INDIA, G. P. Saroha Maharshi Dayanand University Rohtak. INDIA a study on video surveillance system for object detection and tracking. In: Proceedings of the 10th INDIACom; INDIACom-2016; IEEE Conference ID: 37465 2016 3rd International Conference on “Computing for Sustainable Global Development”, 16th–18th March, 2016.
Aishwarya Singh, 2019, September 4, Feature Engineering for images: a valuable Introduction of the HOG feature descriptor. https://www.analyticsvidhya.com/blog/2019/09/feature-engineering-images-introduction-hog-feature-descriptor.
ODSC, Open Data Science. Overview of YOLO object detection Algorithm. 2018. obetectionalgorithm7b52a745d3e0#:~:text=YOLO%20is%20a%20clever%20convolutional,and%20prob abilities%20for %20each%20region.
Human Detection and Tracking for Video Surveillance. A cognitive science approach, 2017 IEEE International Conference on Computer Vision Workshops (ICCVW), Date of Conference: 22–29 October 2017. https://doi.org/10.1109/ICCVW.2017.330.
Human Identification using Histogram of Oriented Gradients (HOG) and Non-Maximum Suppression (NMS) for ATM Video Surveillance. Int J Innov Res Comput Sci Technol (IJIRCST). 2021;9(3):1–10. https://doi.org/10.21276/ijircst.2021.9.3.1, www.ijircst.org (ISSN: 2347–5552; Article ID IRP1143)
Real-Time Human Detection and Action Recognition Based on CNN Classifiers S. Hoshino and Niimura K. Optical flow for real-time human detection and action recognition based on CNN classifiers. J Adv Comput Intell Intell Inform. 2019;23(4):735–42
Fast R-CNN Ross Girshick Microsoft Research rbg@microsoft.com, open access version provided by computer Vision Foundation in 2015
A review of Yolo Algorithm Developments Peiyuan Jiang, Daji Ergu*, Fangyao Liu, Ying Cai, Bo Ma, Available online at www.sciencedirect.com Procedia Computer Science 199 (2022) 1066–1073
W. Liu, D. Angelo, D. Ethan, C. Szegedy, S. Reed, C.-Y. Fu, and A. C. Berg. SSD: Single shot multibox detector. In ECCV, 2016.
Blitznet: A real-time deep network for scene understanding. 2017. arXiv:1708.02813v1 [cs.CV].
“A survey on human detection for video surveillance system,” Dharmendrakumar Viradiya Post Graduate Student Computer Science & Engineering Department B. H. Gardi College of Engineering & Technology Rajkot, Gujarat, India, January 2014, Volume 2, Issue 1 JETIR (ISSN-2349–5162).
Lavee G, Rivlin E, Rudzsky M. Understanding video events: a survey of methods for automatic interpretation of semanticoccurrences in video. IEEE Trans Syst Man Cybern C. 2009;39(5):489–504.
Performance Metrics for Evaluating Object and Human Detection and Tracking Systems, Afzal GodilRoger Bostelman Will Shackleford Tsai Hong Michael Shneier Information Access Division Information Technology Laboratory This publication is available free of charge from: https://doi.org/10.6028/NIST.IR.7972.
Athanesious J, Suresh P. Systematic survey on object tracking methods in video. Int J Adv Res Comput Eng Technol. 2012;1:242–7.
A review on object detection and tracking in video surveillance, Sanjeevkumar Angadi Assistant Professor, MIT College of Railway Engineering and Research, Barshi, Maharashtra, India Suvarna Nandyal Professor, Poojya Doddappa Appa College of Engineering, Gulbarga, Karnataka, India, International Journal of Advanced Research in Engineering and Technology (IJARET) Volume 11, Issue 9, September 2020, pp. 1033–1042, Article ID: IJARET_11_09_10, ISSN Print: 0976–6480 and ISSN Online: 0976–6499. https://doi.org/10.34218/IJARET.11.9.2020.102.
Meng F, Wang X, Wang D, Shao F, Fu L. Spatial-semantic and temporal attention mechanism-based online multi-object tracking. Sensors. 2020;20:1653.
Bagherpour P, Cheraghi SA, Bin MohdMokji M. Upper body tracking using KLT and Kalman filter. Procedia Comput Sci. 2012;13:185–91. https://doi.org/10.1016/j.procs.2012.09.127.
Balasubramanian A, Kamate S, Yilmazer N. Utilization of robust video processing techniques to aid efficient object detection and tracking. Procedia Comput Sci. 2014;36:579–86. https://doi.org/10.1016/j.procs.2014.09.057.
Viola P, Jones MJ and Snow D. Detecting pedestrians using patterns of motion and appearance. The 9th ICCV, Nice, France, volume 1, 2003; p 734–41.
Ben Ayed A, Ben Halima M, Alimi AM. MapReduce-based text detection in big data natural scene videos. Procedia Comput Sci. 2015;53:216–23. https://doi.org/10.1016/j.procs.2015.07.297.
Krizhevsky A, Sutskever I, Hinton GE. Image net classification with deep convolutional neural networks. Commun ACM. 2017;60:84–90. https://doi.org/10.1145/3065386.
Kim B, Lee J. A video-based fire detection using deep learning models. Appl Sci. 2019;9: 2862. https://doi.org/10.3390/app9142862.
A Comparative Study of Various Object Detection Algorithms and Performance Analysis Anand John1, Divyakant Meva,Dept. of Computer Applications, Marwari University, Rajkot, India Corresponding Author: anand_john@yahoo.com, DOI:https://doi.org/10.26438/ijcse/v8i10.158163 | Available online at: www.ijcseonline.org Received: 20/Oct/2020, Accepted: 24/Oct/2020, Published: 31/Oct/2020.
Srivastava S, Divekar AV, Anilkumar C, Naik I, Kulkarni V, Pattabiraman V. Comparative analysis of deep learning image detection algorithms. J Big Data. 2021;8:66. https://doi.org/10.1186/s40537-021-00434-w.
Overview of Object Detection Algorithms Using Convolutional Neural Networks Junsong Ren, Yi Wang*, School of Computer Science and Engineering, Sichuan University of Science and Engineering, Yibing, China, Journal of Computer and Communications, 2022, 10, 115–132, https://www.scirp.org/journal/jcc ISSN Online: 2327–5227 ISSN Print: 2327–5219.
Yu F and Koltun V. Multi-scale context aggregation by dilated convolutions. 2015. https://arxiv.org/abs/1511.07122.
Howard AG, Zhu M, Chen B, Kalenichenko D, Wang W, Weyand T et al. Mobilenets: efficient convolutional neural networks for mobile vision applications. 2017. https://arxiv.org/abs/1704.04861.
Noh H, Hong S, Han B. Learning deconvolution network for semantic segmentation. Proc IEEE Int Conf Comput Vis Santiago. 2015. https://doi.org/10.1109/ICCV.2015.178.
Chen R, Wang M, Lai Y. Analysis of the role and robustness of artificial intelligence in commodity image recognition under deep learning neural network. PLoS ONE. 2020;15: e0235783. https://doi.org/10.1371/journal.pone.0235783.
He K, Zhang X, Ran S, Sun J. Deep residual learning for image recognition. In: Proceedings of the 2016 IEEE Conference on Computer Vision and Pattern Recognition (CVPR), Seattle, WA, USA, 2016; pp. 770–8.
Eom H, Lee D, Han S, Hariyani YS, Lim Y, Sohn I, Park K, Park C. End-to-end deep learning architecture for continuous blood pressure estimation using attention mechanism. Sensors. 2020;20:2338.
Avidan S. Support vector is tracking. IEEE Trans Pattern Anal Mach Intell. 2004;26:1064–72. https://doi.org/10.1109/TPAMI.2004.53.
Badrinarayanan V, Perez P, Le Clerc F and Oisel L (2007) Probabilistic color and adaptive multi-feature tracking with dynamically switched priority between cues. In: 2007 IEEE 11th International Conference on Computer Vision. IEEE, p. 1–8. https://doi.org/10.1109/ICCV.2007.4408955.
Krizhevsky A, Sutskever I, Hinton GE. ImageNet classification with deep convolutional neural networks. Adv Neural Inf Process Syst. 2012;25:1097–105.
Xu B, Wang N, Chen T and Li M (2015) Empirical evaluation of rectified activations in convolutional network. https://arxiv.org/abs/1505.00853
Clevert D-A, Unterthiner T and Hochreiter S (2015) Fast and accurate deep network learning by Exponential Linear Units (ELUs). https://arxiv.org/abs/1511.07289.
Funding
There is no funding received for this work.
Author information
Authors and Affiliations
Corresponding author
Ethics declarations
Conflict of Interest
The authors declare that there are no conflicts of interest regarding the publication of this paper.
Additional information
Publisher's Note
Springer Nature remains neutral with regard to jurisdictional claims in published maps and institutional affiliations.
This article is part of the topical collection “Advances in Computational Intelligence for Artificial Intelligence, Machine Learning, Internet of Things and Data Analytics” guest edited by S. Meenakshi Sundaram, Young Lee, and Gururaj K. S.
Rights and permissions
Springer Nature or its licensor (e.g. a society or other partner) holds exclusive rights to this article under a publishing agreement with the author(s) or other rightsholder(s); author self-archiving of the accepted manuscript version of this article is solely governed by the terms of such publishing agreement and applicable law.
About this article
Cite this article
Usha Rani, J., Raviraj, P. Real-Time Human Detection for Intelligent Video Surveillance: An Empirical Research and In-depth Review of its Applications. SN COMPUT. SCI. 4, 258 (2023). https://doi.org/10.1007/s42979-022-01654-4
Received:
Accepted:
Published:
DOI: https://doi.org/10.1007/s42979-022-01654-4