Abstract
Six population-based hybrid algorithms are applied to train the multilayer perceptron (MLP) to improve the classification accuracy, in the stability assessment. A complex problem of slope stability against failure is designed in Optum G2 software. Considering four key factors of shear strength of clayey soil, slope angle, the ratio of foundation distance from the slope to the foundation length, and the applied surcharge, the stability or failure of the proposed slope are anticipated. The provided data are used to develop the MLP combined with biogeography-based optimization (BBO), ant colony optimization (ACO), genetic algorithm (GA), evolutionary strategy (ES), particle swarm optimization (PSO) and probability-based incremental learning (PBIL). The results revealed that the BBO-MLP with the obtained area under the receiving operating characteristic curve (AUROC) of 0.995 and the classification ratio (CR) of 92.4% is the most accurate model followed by GA-MLP (AUROC = 0.960 and CR = 84.3%), PBIL-MLP (AUROC = 0.948 and CR = 79.3%), ES-MLP (AUROC = 0.879 and CR = 65.7%), PSO-MLP (AUROC = 0.878 and CR = 71.3%), and ACO-MLP (AUROC = 0.798 and CR = 60.7%).






Similar content being viewed by others
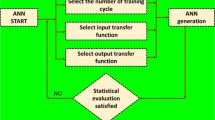
References
Xiao J, Gong W, Martin JR II, Shen M, Luo Z (2016) Probabilistic seismic stability analysis of slope at a given site in a specified exposure time. Eng Geol 212:53–62
Li S, Zhao H, Ru Z, Sun Q (2016) Probabilistic back analysis based on Bayesian and multi-output support vector machine for a high cut rock slope. Eng Geol 203:178–190
Xu C, Wang L, Tien YM, Chen J-M, Juang CH (2014) Robust design of rock slopes with multiple failure modes: modeling uncertainty of estimated parameter statistics with fuzzy number. Environ Earth Sci 72:2957–2969
Khoshnevisan S, Gong W, Wang L, Juang CH (2014) Robust design in geotechnical engineering—an update. Georisk Assess Manag Risk Engineered Syst Geohazards 8:217–234
Zhou X-P, Zhu B-Z, Juang C-H, Wong LNY (2018) A stability analysis of a layered-soil slope based on random field. Bull Eng Geol Env 2018:1–15
Li D-Q, Jiang S-H, Cao Z-J, Zhou W, Zhou C-B, Zhang L-M (2015) A multiple response-surface method for slope reliability analysis considering spatial variability of soil properties. Eng Geol 187:60–72
Abusharar SW, Han J (2011) Two-dimensional deep-seated slope stability analysis of embankments over stone column-improved soft clay. Eng Geol 120:103–110
Georgiadis K (2010) Undrained bearing capacity of strip footings on slopes. J Geotech Geoenviron Eng 136:677–685
Li AJ, Khoo SY, Wang Y, Lyamin AV (2014) Application of neural network to rock slope stability assessments. CRC Press-Taylor & Francis Group, Boca Raton
Qian ZG, Li AJ, Merifield RS, Lyamin AV (2014) Slope stability charts for two-layered purely cohesive soils based on finite-element limit analysis methods. Int J Geomech 15:06014022
McCulloch WS, Pitts W (1943) A logical calculus of the ideas immanent in nervous activity. Bull Math Biophys 5:115–133
Bui X-N, Nguyen H, Le H-A, Bui H-B, Do N-H (2019) Prediction of blast-induced air over-pressure in open-pit mine: assessment of different artificial intelligence techniques. Natural Resour Res 2019:1–21
Torabi M, Hashemi S, Saybani MR, Shamshirband S, Mosavi A (2019) A Hybrid clustering and classification technique for forecasting short-term energy consumption. Environ Progress Sustain Energy 38:66–76
Nguyen H, Bui X-N, Bui H-B, Mai N-L (2018) A comparative study of artificial neural networks in predicting blast-induced air-blast overpressure at Deo Nai open-pit coal mine. Vietnam. Neural Comput Appl 2018:1–17
Moayedi H, Mehdi R, Abolhasan S, Wan AWJ, Safuan ARA (2019) Optimization of ANFIS with GA and PSO estimating α in driven shafts. Eng Comput 35:1–12
Shahsavar A, Khanmohammadi S, Karimipour A, Goodarzi M (2019) A novel comprehensive experimental study concerned synthesizes and prepare liquid paraffin-Fe3O4 mixture to develop models for both thermal conductivity & viscosity: a new approach of GMDH type of neural network. Int J Heat Mass Transf 131:432–441
Qasem SN, Samadianfard S, Kheshtgar S, Jarhan S, Kisi O, Shamshirband S, Chau K-W (2019) Modeling monthly pan evaporation using wavelet support vector regression and wavelet artificial neural networks in arid and humid climates. Eng Appl Comput Fluid Mech 13:177–187
Jain AK, Mao J, Mohiuddin KM (1996) Artificial neural networks: a tutorial. Computer 29:31–44
Dieu Tien B, Viet-Ha N, Nhat-Duc H (2018) Prediction of soil compression coefficient for urban housing project using novel integration machine learning approach of swarm intelligence and multi-layer perceptron neural network. Adv Eng Inform 38:593–604
Kisi O, Yaseen ZM (2019) The potential of hybrid evolutionary fuzzy intelligence model for suspended sediment concentration prediction. CATENA 174:11–23
Dieu Tien B, Shahabi H, Shirzadi A, Chapi K, Nhat-Duc H, Binh Thai P, Quang-Thanh B, Chuyen-Trung T, Panahi M, Bin Ahamd B, Saro L (2018) A novel integrated approach of relevance vector machine optimized by imperialist competitive algorithm for spatial modeling of shallow landslides. Remote Sens 10:1538
Hoang N-D, Pham A-D (2016) Hybrid artificial intelligence approach based on metaheuristic and machine learning for slope stability assessment: a multinational data analysis. Expert Syst Appl 46:60–68
Gandomi A, Kashani A, Mousavi M, Jalalvandi M (2017) Slope stability analysis using evolutionary optimization techniques. Int J Numer Anal Meth Geomech 41:251–264
Li D-Q, Zheng D, Cao Z-J, Tang X-S, Phoon K-K (2016) Response surface methods for slope reliability analysis: review and comparison. Eng Geol 203:3–14
Taylor DW (1937) Stability of earth slopes. J Boston Soc Civ Eng 24:197–246
Griffiths DV, Lane PA (1999) Slope stability analysis by finite elements. Geotechnique 49:387–403
Choobbasti AJ, Farrokhzad F, Barari A (2009) Prediction of slope stability using artificial neural network (case study: Noabad, Mazandaran, Iran). Arab J Geosci 2:311–319
Srivastava A, Babu GLS (2009) Effect of soil variability on the bearing capacity of clay and in slope stability problems. Eng Geol 108:142–152
Zhang ZF, Liu ZB, Zheng LF, Zhang Y (2014) Development of an adaptive relevance vector machine approach for slope stability inference. Neural Comput Appl 25:2025–2035
Lim K, Li A, Lyamin A (2015) Three-dimensional slope stability assessment of two-layered undrained clay. Comput Geotech 70:1–17
Jellali B, Frikha W (2017) Constrained particle swarm optimization algorithm applied to slope stability. Int J Geomech 17:06017022
Sun Y, Jiang Q, Yin T, Zhou C (2018) A back-analysis method using an intelligent multi-objective optimization for predicting slope deformation induced by excavation. Eng Geol 239:214–228
Bui X-N, Muazu MA, Nguyen H (2019) Optimizing Levenberg–Marquardt backpropagation technique in predicting factor of safety of slopes after two-dimensional OptumG2 analysis. Eng Comput 2019:1–12
Qian Z, Li A, Chen W, Lyamin A, Jiang J (2019) An artificial neural network approach to inhomogeneous soil slope stability predictions based on limit analysis methods. Soils Found 59:2. https://doi.org/10.1016/j.sandf.2018.10.008
Zhou H, Liu H, Yin F, Chu J (2018) Upper and lower bound solutions for pressure-controlled cylindrical and spherical cavity expansion in semi-infinite soil. Comput Geotech 103:93–102
Zhou J, Chen Q, Wang J (2017) Rigid block based lower bound limit analysis method for stability analysis of fractured rock mass considering rock bridge effects. Comput Geotech 86:173–180
Rao PP, Liu Y, Cui JF (2015) Bearing capacity of strip footings on two-layered clay under combined loading. Comput Geotech 69:210–218
Zhang S, Karimi S, Shamshirband S, Mosavi A (2019) Optimization algorithm for reduction the size of Dixon resultant matrix: a case study on mechanical application. CMC Comput Mater Continua 58:567–583
Dorigo M (1992) Optimization, learning and natural algorithms. PhD Thesis, presented in Politecnico di Milano, Italy
Dorigo M, Maniezzo V, Colorni A (1996) Ant system: optimization by a colony of cooperating agents. IEEE Trans Syst Man Cybern B Cybern 26:29–41
Dorigo M, Bonabeau E, Theraulaz G (2000) Ant algorithms and stigmergy. Future Gener Comput Syst 16:851–871
Dorigo M, Blum C (2005) Ant colony optimization theory: a survey. Theoret Comput Sci 344:243–278
Simon D (2008) Biogeography-based optimization. IEEE Trans Evol Comput 12:702–713
Ahmadlou M, Karimi M, Alizadeh S, Shirzadi A, Parvinnejhad D, Shahabi H, Panahi M (2018) Flood susceptibility assessment using integration of adaptive network-based fuzzy inference system (ANFIS) and biogeography-based optimization (BBO) and BAT algorithms (BA). Geocarto Int 2018:1–21
Ma H, Simon D (2011) Blended biogeography-based optimization for constrained optimization. Eng Appl Artif Intell 24:517–525
Ergezer M, Simon D, Du D (2009) Oppositional biogeography-based optimization. IEEE
Rechenberg I (1994) Evolutionsstrategie ’94, Werkstatt Bionik und Evolutionstechnik, Frommann-Holzboog, Stuttgart- Bad Cannstadt, Stuttgart (In German)
Schwefel H-PP (1993) Evolution and optimum seeking: the sixth generation. Wiley, Hoboken
Holland JH (1975) Adaptation in natural and artificial systems: an introductory analysis with applications to biology, control, and artificial intelligence. University of Michigan Press, Ann Arbor
Moayedi H, Raftari M, Sharifi A, Jusoh WAW, Rashid ASA (2019) Optimization of ANFIS with GA and PSO estimating α ratio in driven piles. Eng Comput 2019:1–12
Bui X-N, Moayedi H, Rashid ASA (2019) Developing a predictive method based on optimized M5Rules–GA predicting heating load of an energy-efficient building system. Eng Comput 2019:1–10
Muthusamy S, Manickam LP, Murugesan V, Muthukumaran C, Pugazhendhi A (2019) Pectin extraction from Helianthus annuus (sunflower) heads using RSM and ANN modelling by a genetic algorithm approach. Int J Biol Macromol 124:750–758
Davis LD (1991) Handbook of genetic algorithms, 1st ed. Van Nostrand Reinhold Company, New York, United States. ISBN-13: 978-0442001735
Whitley D (1994) A genetic algorithm tutorial. Stat Comput 4:65–85
Gupta MM (1999) Soft computing and intelligent systems: theory and applications. Elsevier, Berlin
Ling LY (2016) Participatory search algorithms and applications, Orientador: Prof. Dr. Fernando Antonio Campos Gomide. Universidade Estadual de Campinas – UNICAMP, Public university in Campinas, Brazil
Eberhart R, Kennedy J (1995) A new optimizer using particle swarm theory. IEEE, 4–6 Oct. 1995. Nagoya, Japan. https://doi.org/10.1109/MHS.1995.494215
Gao W, Dimitrov D, Abdo H (2018) Tight independent set neighborhood union condition for fractional critical deleted graphs and ID deleted graphs. Discrete Contin Dyn Syst S 12:711–721
Gao W, Guirao JLG, Abdel-Aty M, Xi W (2019) An independent set degree condition for fractional critical deleted graphs. Discrete Contin Dyn Syst S 12:877–886
Gao W, Guirao JLG, Basavanagoud B, Wu J (2018) Partial multi-dividing ontology learning algorithm. Inf Sci 467:35–58
Gao W, Wang W, Dimitrov D, Wang Y (2018) Nano properties analysis via fourth multiplicative ABC indicator calculating. Arab J Chem 11:793–801
Gao W, Wu H, Siddiqui MK, Baig AQ (2018) Study of biological networks using graph theory. Saudi J Biol Sci 25:1212–1219
Moayedi H, Mehrabi M, Mosallanezhad M, Rashid ASA, Pradhan B (2018) Modification of landslide susceptibility mapping using optimized PSO-ANN technique. Eng Comput 2018:1–18
Kennedy J (2010) Particle swarm optimization. Encycl. Mach Learn 2010:760–766
Poli R, Kennedy J, Blackwell T (2007) Particle swarm optimization. Swarm Intell 1:33–57
Sulistiyo MD, Rismala R (2016) Implementation of evolution strategies for classifier model optimization. Indones J Comput (Indo-JC) 1:13–26
Grisales-Noreña L, Gonzalez Montoya D, Ramos-Paja C (2018) Optimal sizing and location of distributed generators based on PBIL and PSO techniques. Energies 11:1018
Dieu Tien B, Shahabi H, Shirzadi A, Chapi K, Alizadeh M, Chen W, Mohammadi A, Bin Ahmad B, Panahi M, Hong H, Tian Y (2018) Landslide detection and susceptibility mapping by AIRSAR data using support vector machine and index of entropy models in Cameron highlands, Malaysia. Remote Sens 10:1527
Pham BT, Prakash I, Jaafari A, Bui DT (2018) Spatial prediction of rainfall-induced landslides using aggregating one-dependence estimators classifier. J Indian Soc Remote Sens 46:1457–1470
Pham BT, Prakash I, Bui DT (2018) Spatial prediction of landslides using a hybrid machine learning approach based on random subspace and classification and regression Trees. Geomorphology 303:256–270
Pham BT, Bui DT, Prakash I (2018) Bagging based support vector machines for spatial prediction of landslides. Environ Earth Sci 77:146
Egan JP (1975) Signal detection theory and ROC-analysis, Academic Press series in cognition and perception. Academic Press, New York, United States
Author information
Authors and Affiliations
Corresponding author
Ethics declarations
Conflict of interest
The authors declare no conflict of interest.
Additional information
Publisher's Note
Springer Nature remains neutral with regard to jurisdictional claims in published maps and institutional affiliations.
Rights and permissions
About this article
Cite this article
Yuan, C., Moayedi, H. The performance of six neural-evolutionary classification techniques combined with multi-layer perception in two-layered cohesive slope stability analysis and failure recognition. Engineering with Computers 36, 1705–1714 (2020). https://doi.org/10.1007/s00366-019-00791-4
Received:
Accepted:
Published:
Issue Date:
DOI: https://doi.org/10.1007/s00366-019-00791-4