Abstract
Extraction of built-up area (BUA) is essential for proper city planning and management. It enables the concerned authorities to formulate better city development policies and manage emergent disasters. However, the traditionally used optical data present spectral confusion where BUAs are mixed with other features adding to management complexities. Therefore, an advanced automated method is required to extract the spectral and textural features from satellite data for the pattern recognition of BUA. Landsat-8 Operational Land Imager (OLI) has been used in the current study to identify the pattern and extract BUA of Gujranwala, Pakistan. First, eight textural features based on the gray-level co-occurrence matrix (GLCM) are selected and combined with multispectral data. Then, feature selection methods are applied to select optimal features used to train the proposed support vector machine (SVM) classifier. Finally, the results from SVM classifiers are compared with k-nearest neighbor (k-NN) and backpropagation neural network (BP-NN) to highlight any improvements in results. The comparisons show that the proposed approach increases the overall accuracy of linear-SVM by 8.41%, radial basis function SVM by 8.3%, BP-NN by 7.63%, and k-NN by 6.6%. This can help city managers and planners to extract critical BUA information in otherwise unplanned and rapidly expanding cities to move toward smart and sustainable cities.










Similar content being viewed by others
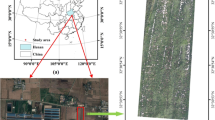
Data availability
Data are available with the first author and can be shared with anyone upon reasonable request.
References
Aboelata A, Sodoudi S (2020) Evaluating the effect of trees on UHI mitigation and reduction of energy usage in different built up areas in Cairo. Build Environ 168:106490
Aborisade D, Ojo J, Amole A, Durodola A (2014) Comparative analysis of textural features derived from GLCM for ultrasound liver image classification. Energy 2(10)
Akbarpour N, Salehi-Amiri A, Hajiaghaei-Keshteli M, Oliva D (2021) An innovative waste management system in a smart city under stochastic optimization using vehicle routing problem. Soft Comput 25(8):6707–6727
Alkhammash E (2020) Formal modelling of owl ontologies-based requirements for the development of safe and secure smart city systems. Soft Comput 24(15):11095–11108
As-syakur A, Adnyana I, Arthana IW, Nuarsa IW JRS (2012) Enhanced built-up and bareness index (EBBI) for mapping built-up and bare land in an urban area 4(10):2957–2970
Aslam B, Maqsoom A, Khalid N, Ullah F, Sepasgozar S (2021) Urban overheating assessment through prediction of surface temperatures: a case study of Karachi, Pakistan. ISPRS Int J Geo-Inf 10(8):539
Atif, S., Umar, M., & Ullah, F. (2021). Investigating the flood damages in Lower Indus Basin since 2000: Spatiotemporal analyses of the major flood events. Natural Hazards, 1–27.
Awais M, Aslam B, Maqsoom A, Khalil U, Ullah F, Azam S, Imran M (2021) Assessing nitrate contamination risks in groundwater: a machine learning approach. Appl Sci 11(21):10034
Azhar A, Adil S (2016) Effect of agglomeration on socio-economic outcomes: a district level panel study of Punjab. The Pakistan development review, 155–170. Accepted from http://www.jstor.org/stable/44986481
Bhatti SS, Tripathi NK (2014) Built-up area extraction using Landsat 8 OLI imagery. Gisci Remote Sens 51(4):445–467
Bouzekri S, Lasbet AA, Lachehab A (2015) A new spectral index for extraction of built-up area using Landsat-8 data. J Indian Soc Remote Sens 43(4):867–873
Bramhe VS, Ghosh SK, Garg PK (2020) Extraction of built-up areas from Landsat-8 OLI data based on spectral-textural information and feature selection using support vector machine method. Geocarto Int 35(10):1067–1087
Clausi DA (2002) An analysis of co-occurrence texture statistics as a function of grey level quantization. Canadian J Remote Sens 28(1):45–62
Cover T, Hart P (1967) Nearest neighbor pattern classification. IEEE Trans Inf Theory 13(1):21–27
Dasarathy BV (1991) Nearest neighbor (NN) norms: NN pattern classification techniques. IEEE Comput Soc Tutor
De Benedictis L, Tajoli L (2011) The world trade network. World Econ 34(8):1417–1454
Feng S, Fan F (2021) Impervious surface extraction based on different methods from multiple spatial resolution images: a comprehensive comparison. Int J Digital Earth 14:1–27
Firozjaei MK, Sedighi A, Kiavarz M, Qureshi S, Haase D, Alavipanah SK (2019) Automated built-up extraction index: a new technique for mapping surface built-up areas using LANDSAT 8 OLI imagery. Remote Sens 11(17):1966
Fukunaga K (2013) Introduction to statistical pattern recognition: Elsevier
Gillani S, Rehman S, Ahmad H, Rehman A, Ali S, Ahmad A, Ateeq Z (2019) Appraisal of urban heat Island over Gujranwala and its environmental impact assessment using satellite imagery 1:1–14
Gong P, Liang S, Carlton EJ, Jiang Q, Wu J, Wang L, Remais JV (2012) Urbanisation and health in China. The Lancet 379(9818):843–852. https://doi.org/10.1016/S0140-6736(11)61878-3
Gopal S, Woodcock C (1996) Remote sensing of forest change using artificial neural networks. IEEE Trans Geosci Remote Sens 34(2):398–404
Graf ABA, Smola AJ, Borer S (2003) Classification in a normalized feature space using support vector machines. IEEE Trans Neural Networks 14(3):597–605. https://doi.org/10.1109/TNN.2003.811708
Guha S, Govil H, Dey A, Gill N (2018) Analytical study of land surface temperature with NDVI and NDBI using Landsat 8 OLI and TIRS data in Florence and Naples city, Italy. Eur J Remote Sens 51(1):667–678. https://doi.org/10.1080/22797254.2018.1474494
Halder B, Bandyopadhyay J, Banik P (2021) Monitoring the effect of urban development on urban heat island based on remote sensing and geo-spatial approach in Kolkata and adjacent areas. India. Sustain Cities Soc 74:103186
Hall-Beyer M (2017) Practical guidelines for choosing GLCM textures to use in landscape classification tasks over a range of moderate spatial scales. Int J Remote Sens 38(5):1312–1338
Haralick RM, Shanmugam K, Dinstein IH (1973) Textural features for image classification. IEEE Trans Syst Man Cybernet (6):610–621
Huang X, Zhang L, Li P (2007) Classification and extraction of spatial features in urban areas using high-resolution multispectral imagery. IEEE Geosci Remote Sens Lett 4(2):260–264. https://doi.org/10.1109/LGRS.2006.890540
İlsever M, Ünsalan C (2013) Locating the urban area in satellite images to detect changes in them. In: Paper presented at the 2013 6th international conference on recent advances in space technologies (RAST)
Ilyas H, Ilyas S, Ahmad S, Nawaz Chaudhry M (2017) Waste generation rate and composition analysis of solid waste in Gujranwala City Pakistan. Int J Waste Resourc. https://doi.org/10.4172/2252-5211.1000297
Jehangir W, Qureshi A, Ali N (2002) Conjunctive water management in the Rechna Doab: an overview of resources and issues
Jiang C, Miao Y, Xi Z (2021) A new method of extracting built-up area based on multi-source remote sensing data: a case study of baoding Central City, China. Geocarto International (just-accepted), 1–13
Jimenez-Rodriguez LO, Arzuaga-Cruz E, Velez-Reyes M (2007) Unsupervised linear feature-extraction methods and their effects in the classification of high-dimensional data. IEEE Trans Geosci Remote Sens 45(2):469–483. https://doi.org/10.1109/TGRS.2006.885412
Kaimaris D, Patias P (2016) Population estimation in an urban area with remote sensing and geographical information systems. Int J Adv Remote Sens GIS 5(6):1795–1812
Kalra D, Pradhan MR (2021) Enduring data analytics for reliable data management in handling smart city services. Soft Comput 25:1–13
Kawamura M (1996) Relation between social and environmental conditions in Colombo Sri Lanka and the urban index estimated by satellite remote sensing data. In: Paper presented at the proceedings of 51st annual conference of the Japan society of civil engineers
Kwak N, Choi C-H (2002) Input feature selection for classification problems. IEEE Trans Neural Networks 13(1):143–159
Li E, Du P, Samat A, Xia J, Che M (2015) An automatic approach for urban land-cover classification from Landsat-8 OLI data. Int J Remote Sens 36(24):5983–6007
Lin W, Sun Y, Wang D, Li Y, Yu X (2021) Estimation model of dust deposition capacity of common vegetation based on spectral characteristics in Shanghai, China. Sustain Cities Soc 70:102915
Liu H, Motoda H (2007) Computational methods of feature selection: CRC Press
Liu J, Liu Z, Zhang H, Yuan H, Manokaran KB, Maheshwari M (2021) Multi-sensor information fusion for IoT in automated guided vehicle in smart city. Soft Comput 25:1–13
Mangan SD, Oral GK, Sozen I, Kocagil IE (2020) Evaluation of settlement textures in terms of building energy, economic performance, and outdoor thermal comfort. Sustain Cities Soc 56:102110
Maqsoom A, Aslam B, Gul ME, Ullah F, Kouzani AZ, Mahmud M, Nawaz A (2021) Using multivariate regression and ANN models to predict properties of concrete cured under hot weather. Sustainability 13(18):10164
Melgani F, Bruzzone L (2004) Classification of hyperspectral remote sensing images with support vector machines. IEEE Trans Geosci Remote Sens 42(8):1778–1790
Meng Q, Zhang L, Sun Z, Meng F, Wang L, Sun Y (2018) Characterizing spatial and temporal trends of surface urban heat island effect in an urban main built-up area: a 12-year case study in Beijing, China. Remote Sens Environ 204:826–837
Molina DE, Gleich D, Datcu M (2010) Gibbs random field models for model-based despeckling of SAR images. IEEE Geosci Remote Sens Lett 7(1):73–77. https://doi.org/10.1109/LGRS.2009.2020698
Munawar HS, Ullah F, Khan SI, Qadir Z, Qayyum S (2021a) UAV assisted spatiotemporal analysis and management of bushfires: a case study of the 2020 victorian bushfires. Fire 4(3):40
Munawar HS, Ullah F, Qayyum S, Heravi A (2021b) Application of deep learning on UAV-based aerial images for flood detection. Smart Cities 4(3):1220–1242
Munawar HS, Ullah F, Qayyum S, Khan SI, Mojtahedi M (2021c) UAVs in disaster management: application of integrated aerial imagery and convolutional neural network for flood detection. Sustainability 13(14):7547
Narkhede S (2018) Understanding auc-roc curve. Towards Data Sci 26:220–227
Nations U (2015) The world population prospects: 2015 Revision. Accepted from https://www.un.org/en/development/desa/publications/world-population-prospects-2015-revision.html
Naz N, Zaidi S-U-H (2013) Historical perspective of urban development of Gujranwala. J Res Arch Plan 14(1):21–38. Accepted from https://jrap.neduet.edu.pk/arch-journal/JRAP_2013(FirstIssue)/JRAP-2013(FirstIssue).pdf#page=26
Neelakandan S, Berlin M, Tripathi S, Devi VB, Bhardwaj I, Arulkumar N (2021) IoT-based traffic prediction and traffic signal control system for smart city. Soft Comput 25:1–8
Ohana-Levi N, Paz-Kagan T, Panov N, Peeters A, Tsoar A, Karnieli A (2019) Time series analysis of vegetation-cover response to environmental factors and residential development in a dryland region. Gisci Remote Sens 56(3):362–387
Patel N, Mukherjee R (2015) Extraction of impervious features from spectral indices using artificial neural network. Arab J Geosci 8(6):3729–3741. https://doi.org/10.1007/s12517-014-1492-x
Pohjalainen J, Räsänen O, Kadioglu S (2015) Feature selection methods and their combinations in high-dimensional classification of speaker likability, intelligibility and personality traits. Comput Speech Language 29(1):145–171
Prasomsup W, Piyatadsananon P, Aunphoklang W, Boonrang A (2020) Extraction Technic for Built-up Area Classification in Landsat 8 Imagery. Int J Environ Sci Develop 11(1):15–20
Qayyum S, Ullah F, Al-Turjman F, Mojtahedi M (2021) Managing smart cities through six sigma DMADICV method: A review-based conceptual framework. Sustain Cities Soc 72:103022
Rahman G, Rahman A-U, Anwar M, Khan M, Ashraf H, Zafar U (2017) Socio-economic damages caused by the 2014 Flood in Punjab Province, Pakistan. Proceed Pakistan Acad Sci 54:365–374
Rumelhart DE, Hinton GE, Williams RJ (1986) Learning representations by back-propagating errors. Nature 323(6088):533–536. https://doi.org/10.1038/323533a0
Shahfahad Mourya M, Kumari B, Tayyab M, Paarcha AA, Rahman A (2020) Indices based assessment of built-up density and urban expansion of fast growing Surat city using multi-temporal Landsat data sets. GeoJournal. https://doi.org/10.1007/s10708-020-10148-w
Shi K, Huang C, Yu B, Yin B, Huang Y, Wu J (2014) Evaluation of NPP-VIIRS night-time light composite data for extracting built-up urban areas. Remote Sens Lett 5(4):358–366. https://doi.org/10.1080/2150704X.2014.905728
Sun L, Tang L, Shao G, Qiu Q, Lan T, Shao J (2020) A machine learning-based classification system for urban built-up areas using multiple classifiers and data sources. Remote Sens 12(1):91
Tan Y, Xiong S, Yan P (2020) Multi-branch convolutional neural network for built-up area extraction from remote sensing image. Neurocomputing 396:358–374. https://doi.org/10.1016/j.neucom.2018.09.106
Tian P, Li J, Cao L, Pu R, Wang Z, Zhang H, Gong H (2021) Assessing spatial characteristics of urban heat islands from the perspective of an urban expansion and ecological landscape. Sustain Cities Soc 74:103208
Ullah F, Khan SI, Munawar HS, Qadir Z, Qayyum S (2021a) UAV based spatiotemporal analysis of the 2019–2020 new south wales bushfires. Sustainability 13(18):10207
Ullah F, Qayyum S, Thaheem MJ, Al-Turjman F, Sepasgozar SM (2021b) Risk management in sustainable smart cities governance: a TOE framework. Technol Forecast Soc Change 167:120743
Vapnik V (2013) The nature of statistical learning theory: Springer
Vigneshwaran S, Kumar SV (2018) Extraction of built-up area using high resolution sentinel-2a and google satellite imagery. Int Arch Photogrammetry Remote Sens Spatial Inf Sci 42
Wang K, Li Z, Zhang J, Wu X, Jia M, Wu L (2020) Built-up land expansion and its impacts on optimizing green infrastructure networks in a resource-dependent city. Sustain Cities Soc 55:102026
Waqar MM, Mirza JF, Mumtaz R, Hussain EJOASR (2012) Development of new indices for extraction of built-up area and bare soil from landsat data 1(1):4
Weizman L, Goldberger J (2009) Urban-area segmentation using visual words. IEEE Geosci Remote Sens Lett 6(3):388–392
Whitney AW (1971) A direct method of nonparametric measurement selection. IEEE Trans Comput 100(9):1100–1103
Xie W, Lei J, Fang S, Li Y, Jia X, Li M (2021) Dual feature extraction network for hyperspectral image analysis. Pattern Recogn 118:107992
Xu H (2008) A new index for delineating built-up land features in satellite imagery. Int J Remote Sens 29(14):4269–4276
Xu J, Xiao W, He T, Deng X, Chen W (2021) Extraction of built-up area using multi-sensor data—a case study based on Google earth engine in Zhejiang Province, China. Int J Remote Sens 42(2):389–404. https://doi.org/10.1080/01431161.2020.1809027
Yu B, Tang M, Wu Q, Yang C, Deng S, Shi K, Chen Z (2018) Urban built-up area extraction from log-transformed NPP-VIIRS nighttime light composite data. IEEE Geosci Remote Sens Lett 15(8):1279–1283
Zhang H, Lin H, Li Y (2015) Impacts of feature normalization on optical and sar data fusion for land use/land cover classification. IEEE Geosci Remote Sens Lett 12(5):1061–1065. https://doi.org/10.1109/LGRS.2014.2377722
Zhao Q, Shi C-Z, Luo L-P (2014) Role of the texture features of images in the diagnosis of solitary pulmonary nodules in different sizes. Chin J Cancer Res 26(4):451
Zhong P, Wang R (2007) A multiple conditional random fields ensemble model for urban area detection in remote sensing optical images. IEEE Trans Geosci Remote Sens 45(12):3978–3988
Funding
This research did not receive any specific grant from funding agencies in the public, commercial, or not-for-profit sectors.
Author information
Authors and Affiliations
Corresponding author
Ethics declarations
Conflict of interest
The authors declare no conflict of interest.
Additional information
Communicated by Irfan Uddin.
Publisher's Note
Springer Nature remains neutral with regard to jurisdictional claims in published maps and institutional affiliations.
Rights and permissions
About this article
Cite this article
Maqsoom, A., Aslam, B., Yousafzai, A. et al. Extracting built-up areas from spectro-textural information using machine learning. Soft Comput 26, 7789–7808 (2022). https://doi.org/10.1007/s00500-022-06794-6
Accepted:
Published:
Issue Date:
DOI: https://doi.org/10.1007/s00500-022-06794-6