Abstract
With the increasing popularity of product assortments by recommender system, it becomes increasingly important for online platform and sellers to investigate their economic impact and manage the links of products. Different from the previous product network, this study constructs and investigates the role of similarity product network from similar products’ recommender system by using data from Taobao.com. The characters of similarity product network exhibits influence on product demand. Mining of similar product’s link reveals that the more a product is being linked, the greater of the demand, the impact of product’s degree is different from type of products. In addition, the results show that the centralization of network has a negative impact on focal product’s demand. This study also examines the spillover effect of similar products’ reviews (UGC) as well as similar products’ description (MGC), especially focuses on the semantic similarity. The results reveal that the semantic similarity of recommended product’s reviews and products’ description have negative spillover effect on demand. The more similar of recommended product’s reviews and the product’s description, the stronger the effect. Specifically, similarity of MGC exhibits a stronger impact than that of UGC on focal product’s demand for search goods than for experience goods. The findings provide insights to marketing practitioners by helping understand the effects of similarity and link of product on the consumer’s decision.




Similar content being viewed by others
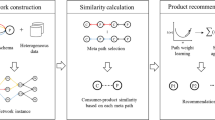
References
Sarwar, B., Karypis, G., Konstan, J., & Riedl, J. (2001). Item-based collaborative filtering recommendation algorithms. In Proceedings of the 10th international conference on World Wide Web (pp. 285–295). ACM.
Van Trijp, H. C., Hoyer, W. D., & Inman, J. J. (1996). Why switch? Product category: Level explanations for true variety-seeking behavior. Journal of Marketing Research, 33, 281–292.
Pathak, B., Garfinkel, R., & Gopal, R. D. (2010). Empirical analysis of the impact of recommender systems on sales. Journal of Management Information Systems, 27(2), 159–188.
Oestreicher-Singer, G., & Sundararajan, A. (2012). The visible hand? Demand effects of recommendation networks in electronic markets. Management Science, 58(11), 1963–1981.
Oestreicher-Singer, G., Libai, B., Sivan, L., Carmi, E., & Yassin, O. (2013). The network value of products. Journal of Marketing, 77(3), 1–14.
Lin, Z., Goh, K. Y., & Heng, C. S. (2017). The demand effects of product recommendation networks: An empirical analysis of network diversity and stability. Management Information Systems Quarterly, 41(2), 397–426.
Henderson, J. M., & Quandt, R. E. (1958). Microeconomic theory. New York: McGraw-Hill.
Ratneshwar, S., & Shocker, A. D. (1991). Substitution in use and the role of usage context in product category structures. Journal of Marketing Research, 28(3), 281–295.
Park, C. W., Milberg, S., & Lawson, R. (1991). Evaluation of brand extensions: The role of product feature similarity and brand concept consistency. Journal of Consumer Research, 18(2), 185–193.
Muthukrishnan, A. V., & Weitz, B. A. (1991). Role of product knowledge in evaluation of brand extension. Advances in Consumer Research, 18(1), 407–413.
Lefkoff-Hagius, R., & Mason, C. H. (1993). Characteristic, beneficial, and image attributes in consumer judgments of similarity and preference. Journal of Consumer Research, 20(1), 100–110.
Bangyong, L., Juanzi, L. I., & Kehong, W. (2004). Web page recommendation model for the semantic web. Journal of Tsinghua University, 44(9), 1271–1272.
Zhai, Z., Liu, B., Xu, H., et al. (2011). Clustering product features for opinion mining. In Proceedings of the fourth ACM international conference on Web search and data mining (pp. 347–354). ACM.
Loken, B., & Ward, J. (1990). Alternative approaches to understanding the determinants of typicality. Journal of Consumer Research, 17(2), 111–126.
Hem, L. E., De Chernatony, L., & Iversen, N. M. (2003). Factors influencing successful brand extensions. Journal of Marketing Management, 19(7–8), 781–806.
Yeo, J., & Park, J. (2006). Effects of parent-extension similarity and self regulatory focus on evaluations of brand extensions. Journal of Consumer Psychology, 16(3), 272–282.
Chang, C. C., Lin, B. C., & Chang, S. S. (2011). The relative advantages of benefit overlap versus category similarity in brand extension evaluation: The moderating role of self-regulatory focus. Marketing Letters, 22(4), 391–404.
Colucci, L., Doshi, P., Lee, K. L., Liang, J., Lin, Y., Vashishtha, I., & Jude, A. (2016). Evaluating item-item similarity algorithms for movies. In Proceedings of the 2016 CHI conference extended abstracts on human factors in computing systems (pp. 2141–2147).
Zhou, W., Mok, P. Y., Zhou, Y., Zhou, Y., Shen, J., Qu, Q., et al. (2019). Fashion recommendations through cross-media information retrieval. Journal of Visual Communication and Image Representation, 61, 112–120.
Anderson, C. (2006). The long tail: Why the future of business is selling less of more. New York: Hachette Books.
Bergemann, D., & Ozmen, D. (2006). Optimal pricing with recommender systems. In Proceedings of the 7th ACM conference on Electronic commerce (pp. 43–51).
Schafer, J. B., Konstan, J. A., & Riedl, J. (2001). E-commerce recommendation applications. In R. Kohavi & F. Provost (Eds.), Applications of data mining to electronic commerce (pp. 115–153). Berlin: Springer.
Brynjolfsson, E., Hu, Y., & Smith, M. D. (2003). Consumer surplus in the digital economy: Estimating the value of increased product variety at online booksellers. Management Science, 49(11), 1580–1596.
Fleder, D. M., Hosanagar, K., & Buja, A. (2008). Will the global village fracture into tribes: Recommender systems and their effects on consumers, Working Paper (pp. 12–30). NET Institute.
Fleder, D. M., Hosanagar, K., & Buja, A. (2010). Recommender systems and their effects on consumers: The fragmentation debate. EC, 229, 230.
Yoon, V. Y., Hostler, R. E., Guo, Z., et al. (2013). Assessing the moderating effect of consumer product knowledge and online shopping experience on using recommendation agents for customer loyalty. Decision Support Systems, 55(4), 883–893.
Panniello, U., Gorgoglione, M., & Tuzhilin, A. (2016). Research note—In CARSs we trust: How context-aware recommendations affect customers’ trust and other business performance measures of recommender systems. Information Systems Research, 27(1), 182–196.
Agarwal, P., Vempati, S., & Borar, S. (2018). Personalizing similar product recommendations in fashion E-commerce. In AI for fashion. The third international workshop on fashion and KDD.
Leem, B., & Chun, H. (2014). An impact of online recommendation network on demand. Expert Systems with Applications, 41(4), 1723–1729.
Carmi, E., Oestreicher-Singer, G., Stettner, U., & Sundararajan, A. (2017). Is oprah contagious? The depth of diffusion of demand shocks in a product network. Management Information Systems Quarterly, 41(1), 207–221.
Kumar, A., & Hosanagar, K. (2018). Measuring the value of recommendation links on product demand. Information Systems Research (forthcoming).
Chevalier, J. A., & Mayzlin, D. (2006). The effect of word of mouth on sales: Online book reviews. Journal of Marketing Research, 43(3), 345–354.
Lim, B. C., & Chung, C. M. (2011). The impact of word-of-mouth communication on attribute evaluation. Journal of Business Research, 64(1), 18–23.
Cui, G., Lui, H. K., & Guo, X. (2010). Online reviews as a driver of new product sales. In Management of e-Commerce and e-Government (ICMeCG). Fourth international conference on IEEE (pp. 20–25).
Weathers, D., Swain, S. D., & Grover, V. (2015). Can online product reviews be more helpful? Examining characteristics of information content by product type. Decision Support Systems, 79, 12–23.
Goh, K. Y., Heng, C. S., & Lin, Z. (2013). Social media brand community and consumer behavior: Quantifying the relative impact of user-and marketer-generated content. Information Systems Research, 24(1), 88–107.
Maslowska, E., Malthouse, E. C., & Viswanathan, V. (2017). Do customer reviews drive purchase decisions? The moderating roles of review exposure and price. Decision Support Systems, 98, 1–9.
Ahluwalia, R., Unnava, H. R., & Burnkrant, R. E. (2001). The moderating role of commitment on the spillover effect of marketing communications. Journal of Marketing Research, 38(4), 458–470.
Libai, B., Muller, E., & Peres, R. (2009). The role of within-brand and cross-brand communications in competitive growth. Journal of Marketing, 73(3), 19–34.
Peres, R., & Van den Bulte, C. (2014). When to take or forgo new product exclusivity: Balancing protection from competition against word-of-mouth spillover. Journal of Marketing, 78(2), 83–100.
Kwark, Y., Chen, J., & Raghunathan, S. (2017). User-generated content and competing firms’ product design. Management Science, 64(10), 4471–4965.
Krishnan, T. V., Bass, F. M., & Kumar, V. (2000). Impact of a late entrant on the diffusion of a new product/service. Journal of Marketing Research, 37(2), 269–278.
Krishnan, T., Seetharaman, P. B., & Vakratsas, D. (2012). The multiple roles of interpersonal communication in new product growth. International Journal of Research in Marketing, 29(3), 292–305.
Kwark, Y., Lee, G. M., Pavlou, P., et al. (2016). The spillover effects of user-generated online product reviews on purchases: Evidence from clickstream data. In ICIS 2016 proceedings.
Page, L., Brin, S., Motwani, R., & Winograd, T. (1999). The PageRank citation ranking: Bringing order to the web. Stanford InfoLab.
Ghose, A., & Ipeirotis, P. G. (2011). Estimating the helpfulness and economic impact of product reviews: Mining text and reviewer characteristics. IEEE Transactions on Knowledge and Data Engineering, 23(10), 1498–1512.
Walters, R. G. (1991). Assessing the impact of retail price promotions on product substitution, complementary purchase, and interstore sales displacement. Journal of Marketing, 55(2), 17–28.
Leone, M. R. P. (1991). Implicit price bundling of retail products: A multiproduct approach to maximizing store profitability. Journal of Marketing, 55(4), 63–76.
Ekdale, B., Namkoong, K., Fung, T. K., & Perlmutter, D. D. (2010). Why blog? (then and now): Exploring the motivations for blogging by popular American political bloggers. New Media and Society, 12(217), 217–234.
Luo, X., Gu, B., Zhang, J., et al. (2017). Expert blogs and consumer perceptions of competing brands. Management Information Systems Quarterly, 41(2), 371–395.
Hoberg, G., & Phillips, G. (2010). Product market synergies and competition in mergers and acquisitions: A text-based analysis. The Review of Financial Studies, 23(10), 3773–3811.
Shi, Z. M., Lee, G., & Whinston, A. B. (2016). Toward a better measure of business proximity: Topic modeling for industry intelligence. Management Information Systems Quarterly, 40(4), 1035–1056.
Khessina, O. M., & Reis, S. (2016). The limits of reflected glory: The beneficial and harmful effects of product name similarity in the US network TV program industry, 1944–2003. Organization Science, 27(2), 411–427.
Hong, Y., Chen, P. Y., & Hitt, L. M. (2012). Measuring product type with dynamics of online product review variance. In ICIS 2012 proceedings.
Nelson, P. (1974). Advertising as information. Journal of Political Economy, 82(4), 729–754.
Manchanda, P., Dubé, J.-P., & Chintagunta, G. P. K. (2006). The effect of banner advertising on internet purchasing. Journal of Marketing Research, 43(1), 98–108.
Zhang, J., & Wedel, M. (2009). The effectiveness of customized promotions in online and offline stores. Journal of Marketing Research, 46(2), 190–206.
Scholz, M., Dorner, V., Landherr, A., & Probst, F. (2013). Awareness, interest, and purchase: The effects of user-and marketer-generated content on purchase decision processes. In 34th international conference on information systems (pp. 1–17).
Mulhern, F. J., & Leone, R. P. (1991). Implicit price bundling of retail products: A multiproduct approach to maximizing store profitability. The Journal of Marketing, 55(4), 63–76.
Russell, G. J., & Petersen, A. (2000). Analysis of cross category dependence in market basket selection. Journal of Retailing, 76(3), 367–392.
Grace, R. C., Kivell, B. M., & Laugesen, M. (2014). Estimating cross-price elasticity of e-cigarettes using a simulated demand procedure. Nicotine & Tobacco Research, 17(5), 592–598.
Reibstein, D. J., Youngblood, S. A., & Fromkin, H. L. (1975). Number of choices and perceived decision freedom as a determinant of satisfaction and consumer behavior. Journal of Applied Psychology, 60(4), 434.
Stephen, A. T., & Toubia, O. (2010). Deriving value from social commerce networks. Journal of Marketing Research, 47(2), 215–228.
Sinclair, P. A. (2009). Network centralization with the Gil Schmidt power centrality index. Social Networks, 31(3), 214–219.
Merida-Campos, C., & Willmott, S. (2007). The impact of betweenness in small world networks on request for proposal coalition formation problems. In Conference on artificial intelligence research and development (Vol. 163, pp. 49–56).
Schilling, M. A., & Phelps, C. C. (2007). Interfirm collaboration networks: The impact of large-scale network structure on firm innovation. Management Science, 53(7), 1113–1126.
Krackhardt, D., & Stern, R. N. (1988). Informal networks and organizational crises: An experimental simulation. Social Psychology Quarterly, 51(2), 123–140.
Cummings, J. N., & Cross, R. (2003). Structural properties of work groups and their consequences for performance. Social Networks, 25(3), 197–210.
Grund, T. U. (2012). Network structure and team performance: The case of English Premier League soccer teams. Social Networks, 34(4), 682–690.
Jones, K. S. (1972). A statistical interpretation of term specificity and its application in retrieval. Journal of Documentation, 28(1), 11–21.
Salton, G., Wong, A., & Yang, C. S. (1975). A vector space model for automatic indexing. Communications of the ACM, 18, 613–620.
Lin, Z., & Goh, K. Y. (2011). Measuring the business value of online social media content for marketers. In ICIS 2011 proceedings (Vol. 16).
Meyers-Levy, J., & Malaviya, P. (1999). Consumers’ processing of persuasive advertisements: An integrative framework of persuasion theories. Journal of Marketing, 63(Special), 45–60.
Russo, J. E., & Chaxel, A. S. (2010). How persuasive messages can influence behavior without awareness. Journal of Consumer Psychology, 20(3), 338–342.
Mas-Colell, A., Whinston, M. D., & Green, J. R. (1995). Microeconomic theory. New York: Oxford University Press.
Fleder, D., & Hosanagar, K. (2009). Blockbuster culture’s next rise or fall: The impact of recommender systems on sales diversity. Management Science, 55(5), 697–712.
Mooney, R. J., &, Roy, L. (2000). Content-based book recommending using learning for text categorization. In Proceedings of the ACM conference on digital libraries.
Ouyang, X., Zhou, P., Li, C. H., & Liu, L. (2015). Sentiment analysis using convolutional neural network. In 2015 IEEE international conference on computer and information technology; ubiquitous computing and communications; dependable, autonomic and secure computing; pervasive intelligence and computing (pp. 2359–2364).
Pei, K., Chen, Y., Ma, J., & Nie, W. (2018). Short text classification research based on TW-CNN. In PACIS 2018 Proceedings (Vol. 41).
Acknowledgements
Funding was provided by the National Natural Science Foundation of China (Grant No. 71672065).
Author information
Authors and Affiliations
Corresponding author
Ethics declarations
Conflict of interest
The authors declared that they have no conflicts of interest to this work. We declare that we do not have any commercial or associative interest that represents a conflict of interest in connection with the work submitted.
Rights and permissions
About this article
Cite this article
Huang, H.J., Yang, J. & Zheng, B. Demand effects of product similarity network in e-commerce platform. Electron Commer Res 21, 297–327 (2021). https://doi.org/10.1007/s10660-019-09352-9
Published:
Issue Date:
DOI: https://doi.org/10.1007/s10660-019-09352-9