Abstract
For an orthogonal frequency-division multiplexing (OFDM) system in high-mobility applications, channel suffers from both frequency-selective and time-selective fading introduced by Doppler shift. Large pilot overhead is needed to estimate the numerous parameters of doubly-selective channel, resulting in low-spectral efficiency. In this paper, considering the correlation of practical wireless channels in high-dimensional signal spaces, a recursive channel estimation scheme based on compressed sensing (CS) is proposed for high-mobility OFDM systems to reduce the pilot overhead. Specifically, by exploiting the sparsity of OFDM channel in basis expansion model (BEM), the sparse BEM coefficients is estimated instead of numerous channel taps. Then we theoretically verify that the BEM coefficients corresponding successive OFDM symbols also share a common support. To utilize this temporal correlation of BEM coefficients, a recursive channel estimation algorithm derived from the classical modified CS algorithm is proposed to improve the present estimation by prior channel information from previous estimation. Theoretical and simulation results demonstrate that the proposed recursive channel estimation scheme preforms better than conventional schemes in various scenarios, even with less pilot overhead.



Similar content being viewed by others
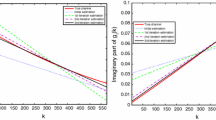
References
Savaux, V., & Louët, Y. (2017). LMMSE channel estimation in OFDM context: A review. IET Signal Processing, 11(2), 123–134.
Hao, J., Wang, J., & Wu, Y. (2015). A new equalizer in doubly-selective channels for TDS-OFDM. IEEE Transactions on Broadcasting, 61(1), 91–97.
Yinsheng, L., Zhenhui, T., Hongjie, H., et al. (2014). Channel estimation for OFDM. IEEE Communications Surveys & Tutorials, 16(4), 1891–1908.
Ronald, N., & Markus, R. (2017). OFDM and FBMC-OQAM in doubly-selective channels: Calculating the bit error probability. IEEE Communications Letters, 21(6), 1297–1300.
Zhang, L., Hong, Z., Thibault, L., et al. (2014). A low-complexity robust OFDM receiver for fast fading channels. IEEE Transaction on Broadcasting, 60(2), 347–357.
Andreas, M., & Marc, E. (2014). The computational complexity of the restricted isometry property, the nullspace property, and related concepts in compressed sensing. IEEE Transactions on Information Theory, 60(2), 1248–1259.
Baraniuk, R. (2017). A survey on compressed sensing in vehicular infotainment systems. IEEE Communications Surveys & Tutorials, 19(4), 2662–2680.
Raghavan, V., & Sayeed, A. (2011). Sublinear capacity scaling laws for sparse MIMO channels. IEEE Transactions on Information Theory, 57(1), 345–364.
Neda, A., Wibowo, H., & Branka, V. (2012). A new iterative doppler-assisted channel estimation joint with parallel ICI cancellation for high-mobility MIMO-OFDM systems. IEEE Transactions on Vehicular Technology, 61(4), 1577–1589.
Taubock, G., Hlawatsch, F., Eiwen, D., et al. (2010). Compressive estimation of doubly-selective channels in multicarrier systems: Leakage effects and sparsity-enhancing processing. IEEE Journal of Selected Topics in Signal Processing, 4(2), 255–271.
Peng, C., Zhuo, C., Yun, R., et al. (2013). Channel estimation for OFDM systems over doubly-selective channels: A distributed compressive sensing based approach. IEEE Transactions on Communication, 61(10), 4173–4185.
Xiang, R., Meixia, T., & Wen, C. (2016). Compressed channel estimation with position based ICI elimination for high-mobility SIMO-OFDM systems. IEEE Transactions on Vehicular Technology, 65(8), 6204–6216.
Xiang, R., Wen, C., & Meixia, T. (2015). Position-based compressed channel estimation and pilot design for high-mobility OFDM systems. IEEE Transactions on Vehicular Technology, 64(5), 1918–1929.
Telatar, I., & Tse, D. (2000). Capacity and mutual information of wideband multipath fading channels. IEEE Transactions on Information Theory, 46(4), 1384–1400.
Zhen, G., Linglong, D., Wei, D., et al. (2016). Structured compressive sensing-based spatio-temporal joint channel estimation for FDD massive MIMO. IEEE Transactions on Communications, 64(2), 601–617.
Dongjae, L. (2016). MIMO OFDM channel estimation via block stagewise orthogonal matching pursuit. IEEE Communications Letters, 20(10), 2115–2118.
Zhen, G., Linglong, D., Wei, D., et al. (2015). Spatially common sparsity based adaptive channel estimation and feedback for FDD massive MIMO. IEEE Transactions on Signal Processing, 63(23), 6169–6183.
Durisi, G., Schuster, U., Bolcskei, H., et al. (2010). Non-coherent capacity of under-spread fading channels. IEEE Transactions on Information Theory, 56(1), 367–395.
Krahmer, F., Deanna, N., & Rachel, W. (2015). Compressive sensing with redundant dictionaries and structured measurements. SIAM Journal on Mathematical Analysis, 47(6), 4606–4629.
Soomin, L., & Angelia, N. (2013). Distributed random projection algorithm for convex optimization. IEEE Journal of Selected Topics in Signal Processing, 7(2), 221–229.
Dennis, S., Saikat, C., & Mikael, S. (2014). Distributed greedy pursuit algorithms. Signal Processing, 105, 298–315.
Qun, M., & Yi, S. (2012). A remark on the restricted isometry property in orthogonal matching pursuit. IEEE Transactions on Information Theory, 58(6), 3654–3656.
Coralia, C., & Andrew, T. (2015). A new and improved quantitative recovery analysis for iterative hard thresholding algorithms in compressed sensing. IEEE Transactions on Information Theory, 61(4), 2019–2042.
Acknowledgements
This research is funded by the Natural Science Foundation of China (Grant: 61271251), the Program for New Century Excellent Talents in University (Grant: NCET -11 - 0873), the Program for Innovative Research Team in University of Chongqing (Grant: KJTD 201343), Program for Fundamental Research of Chongqing Communication Institute (Grant: TZ -CQTY- Y- C-2016 -023) and the open subject of the Chongqing key laboratory of emergency communication (Grant: IRT1299).
Author information
Authors and Affiliations
Corresponding author
Rights and permissions
About this article
Cite this article
Wang, K., Liu, J., Gan, Z. et al. Compressed Sensing Based Recursive Estimation of Doubly-Selective Channels for High-Mobility OFDM Systems. Wireless Pers Commun 102, 1387–1400 (2018). https://doi.org/10.1007/s11277-017-5201-4
Published:
Issue Date:
DOI: https://doi.org/10.1007/s11277-017-5201-4