Abstract
Localization of active neural source (ANS) from measurements on head surface is vital in magnetoencephalography. As neuron-generated magnetic fields are extremely weak, significant uncertainties caused by stochastic measurement interference complicate its localization. This paper presents a novel computational method based on reconstructed magnetic field from sparse noisy measurements for enhanced ANS localization by suppressing effects of unrelated noise. In this approach, the magnetic flux density (MFD) in the nearby current-free space outside the head is reconstructed from measurements through formulating the infinite series solution of the Laplace’s equation, where boundary condition (BC) integrals over the entire measurements provide “smooth” reconstructed MFD with the decrease in unrelated noise. Using a gradient-based method, reconstructed MFDs with good fidelity are selected for enhanced ANS localization. The reconstruction model, spatial interpolation of BC, parametric equivalent current dipole-based inverse estimation algorithm using reconstruction, and gradient-based selection are detailed and validated. The influences of various source depths and measurement signal-to-noise ratio levels on the estimated ANS location are analyzed numerically and compared with a traditional method (where measurements are directly used), and it was demonstrated that gradient-selected high-fidelity reconstructed data can effectively improve the accuracy of ANS localization.





Similar content being viewed by others
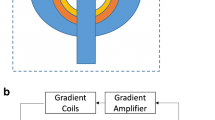
References
Ahonen AI, Hämäläinen MS, Ilmoniemi RJ, Kajola MJ, Knuutila JET, Simola JT, Vilkman VA (1993) Sampling theory for neuromagnetic detector arrays. IEEE Trans Biomed Eng 40:859–869
Ammari H, Vogelius MS, Volkov D (2001) Asymptotic formulas for perturbations in the electromagnetic fields due to the presence of inhomogeneities of small diameter. II. The full Maxwell equations. J Math Pures Appl 80(8):769–814
Ammari H, Bao G, Fleming JL (2002) An inverse source problem for Maxwell’s equations in magnetoencephalography. SIAM J Appl Math 62(4):1369–1382
Ammari H, Moskow S, Vogelius MS (2003) Boundary integral formulae for the reconstruction of electric and electromagnetic inhomogeneities of small volume. ESAIM Control Optim Calc Var 9:49–66
Auranen T, Nummenmaa A, Vanni S, Vehtari A, Hämäläinen MS, Lampinen J, Jääskeläinen IP (2009) Automatic fMRI-guided MEG multidipole localization for visual responses. Hum Brain Mapp 30:1087–1099
Babiloni F, Babiloni C, Carducci F, Romani GL, Rossini PM, Angelone LM, Cincotti F (2004) Multimodal integration of EEG and MEG data: a simulation study with variable signal-to-noise ratio and number of sensors. Hum Brain Mapp 22:52–62
deMunck JC, Huizenga HM, Waldorp LJ, Heethaar RA (2002) Estimating stationary dipoles from MEG/EEG data contaminated with spatially and temporally correlated background noise. IEEE Trans Signal Process 50(7):1565–1572
Fukushima M, Yamashita O, Kanemura A, Ishii S, Kawato M, Sato M (2012) A state-space modeling approach for localization of focal current sources from MEG. IEEE Trans Biomed Eng 59(6):1561–1571
Golubic SJ, Susac A, Grilj V, Ranken D, Huonker R, Haueisen J, Supek S (2011) Size matters: MEG empirical and simulation study on source localization of the earliest visual activity in the occipital cortex. Med Biol Eng Comput 49:545–554
Gonzalez-Moreno A, Aurtenetxe S, Lopez-Gracia M-E, del Pozo F, Maestu F, Nevado A (2014) Signal-to-noise ratio of the MEG signal after preprocessing. J Neurosci Methods 222:56–61
Hämäläinen MS, Hari R, Ilmoniemi RJ, Knuutila J, Lounasmaa OV (1993) Magnetoencephalography: theory, instrumentation and applications to non-invasive studies of the working human brain. Rev Mod Phys 65(2):413–498
Hansen PC, Kringelbach ML, Salmelin RH (2010) MEG: an introduction to methods. Oxford University, New York
Mellinger J, Schalk G, Braun C, Preissl H, Rosenstiel W, Birbaumer N, Kubler A (2007) An MEG-based brain-computer interface (BCI). Neuroimage 36(3):581–593
Nurminen J, Taulu S, Nenonen J, Helle L, Simola J, Ahonen A (2013) Improving MEG performance with additional tangential sensors. IEEE Trans Biomed Eng 60(9):2559–2566
Oishi M, Kameyama S, Masuda H, Tohyama J, Kanazawa O, Sasagawa M, Otsubo H (2006) Single and multiple clusters of magnetoencephalographic dipoles in neocortical epilepsy: significance in characterizing the epileptogenic zone. Epilepsia 47(2):355–364
Ramírez RR, Kopell BH, Butson CR, Hiner BC, Baillet S (2011) Spectral signal space projection algorithm for frequency domain MEG and EEG denoising, whitening, and source imaging. Neuroimage 56:78–92
Song T, Gaa K, Cui L, Feffer L, Lee RR, Huang MX (2008) Evaluation of signal space separation via simulation. Med Biol Eng Comput 46:923–932
Tanaka N, Stufflebeam SM (2014) Clinical application of spatiotemporal distributed source analysis in presurgical evaluation of epilepsy. Front. Hum, Neurosci 8
Taulu S, Simola J (2006) Spatiotemporal signal space separation method for rejecting nearby interference in MEG measurements. Phys Med Biol 51:1759–1768
Uusitalo MA, Ilmoniemi RJ (1997) Signal-space projection method for separating MEG or EEG into components. Med Biol Eng Comput 35:135–140
von Helmholtz H (1853) Ueber einige Gesetze der Vertheilung elektrischer Strome in Korperlichen Leitem, mit Anwendung auf die thierisch-elektrisehen Versuche. Ann Phys Chem 89(211–233):353–377
Sloane NJA, with the collaboration of RH Hardin, WD Smith and others, Tables of Spherical Codes. http://neilSloane.com/packings/
Yutaka U, Kaoru A, Tsunehiro T (2013) Development of a generative model of magnetoencephalography noise that enables brain signal extraction from single-epoch data. Med Biol Eng Comput 51(8):937–951
Acknowledgments
This study was supported by the National Natural Science Foundation of China (No. 51105329), US National Science Foundation EFRI-M3C 1137172, National Basic Research Program (973) of China (Nos. 2013CB035803 and 2011CB013300), and SUTD-ZJU Research Collaboration Grant ZJURP1200102.
Author information
Authors and Affiliations
Corresponding authors
Appendices
Appendix 1: Solutions to magnetic scalar potential in Ω outside the head
Solved by the separation-of-variable method in spherical coordinates, the solutions to Eq. (6c) with BCs (7a–d) are a linear combination of the spherical harmonic functions which are products of trigonometric functions and associated Legendre polynomials \(P_{l}^{m} \left( {\cos \theta } \right)\) of degree l and order m:
Noting that \(\psi \left| {_{r \to \infty } } \right. = 0\), \(a_{l}^{m} = b_{l}^{m} = 0\), and \(c_{l}^{m} = d_{l}^{m}\) are determined from the BCs:
where the modulus of spherical harmonics
and
Appendix 2: H-nearest neighborhood interpolation of measured data on BC surface
The second-order fitting polynomial function in Eq. (20) is used to interpolate among the sparse measured data on a three-dimensional surface:
The coefficients (g 0, g, G) in Eq. (20) are determined by LS technique weighted over the H-nearest neighbor measurements, which minimizes the objective function (21) where w i is determined by the distance δ i between the ith nearest neighbor measurement B si and the point being interpolated:
Rights and permissions
About this article
Cite this article
Shen, Hm., Lee, KM., Hu, L. et al. Effects of reconstructed magnetic field from sparse noisy boundary measurements on localization of active neural source. Med Biol Eng Comput 54, 177–189 (2016). https://doi.org/10.1007/s11517-015-1381-9
Received:
Accepted:
Published:
Issue Date:
DOI: https://doi.org/10.1007/s11517-015-1381-9